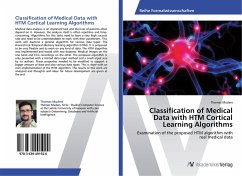
Classification of Medical Data with HTM Cortical Learning Algorithms
Examination of the proposed HTM algorithm with real medical data
Versandkostenfrei!
Versandfertig in 6-10 Tagen
19,99 €
inkl. MwSt.
PAYBACK Punkte
10 °P sammeln!
Medical data analysis is an important task and the lives of patients often depend on it. However, the analysis itself is often repetitive and time-consuming. Algorithms for this tasks need to have a very high success rate and need to be understandable to work with their parameters. This work will examine a general algorithm for various data types: The Hierarchical Temporal Memory learning algorithm (HTM). It is proposed to be very flexible and to work on any kind of data. The HTM algorithm was implemented and tested with two datasets: Medical images on the one hand and ECG recordings on the ot...
Medical data analysis is an important task and the lives of patients often depend on it. However, the analysis itself is often repetitive and time-consuming. Algorithms for this tasks need to have a very high success rate and need to be understandable to work with their parameters. This work will examine a general algorithm for various data types: The Hierarchical Temporal Memory learning algorithm (HTM). It is proposed to be very flexible and to work on any kind of data. The HTM algorithm was implemented and tested with two datasets: Medical images on the one hand and ECG recordings on the other. The proposed algorithm is only presented with a limited data input method and a small input size by its authors. These properties needed to be modified to support a bigger amount of data and also various data types. This is done with an own implementation of the HTM algorithm. The results of this work are analyzed and thoughts and ideas for future development are given at the end.