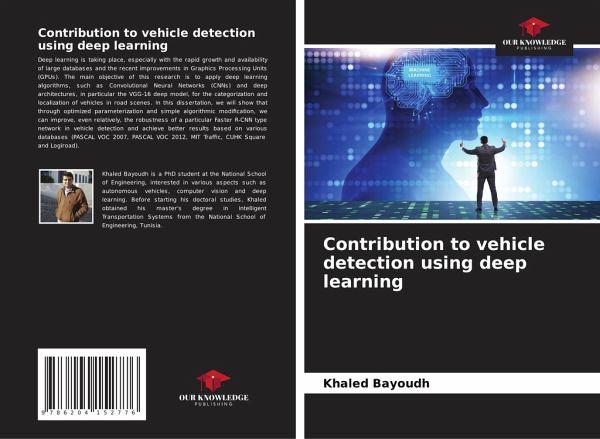
Contribution to vehicle detection using deep learning
Versandkostenfrei!
Versandfertig in 6-10 Tagen
26,99 €
inkl. MwSt.
PAYBACK Punkte
13 °P sammeln!
Deep learning is taking place, especially with the rapid growth and availability of large databases and the recent improvements in Graphics Processing Units (GPUs). The main objective of this research is to apply deep learning algorithms, such as Convolutional Neural Networks (CNNs) and deep architectures, in particular the VGG-16 deep model, for the categorization and localization of vehicles in road scenes. In this dissertation, we will show that through optimized parameterization and simple algorithmic modification, we can improve, even relatively, the robustness of a particular Faster R-CN...
Deep learning is taking place, especially with the rapid growth and availability of large databases and the recent improvements in Graphics Processing Units (GPUs). The main objective of this research is to apply deep learning algorithms, such as Convolutional Neural Networks (CNNs) and deep architectures, in particular the VGG-16 deep model, for the categorization and localization of vehicles in road scenes. In this dissertation, we will show that through optimized parameterization and simple algorithmic modification, we can improve, even relatively, the robustness of a particular Faster R-CNN type network in vehicle detection and achieve better results based on various databases (PASCAL VOC 2007, PASCAL VOC 2012, MIT Traffic, CUHK Square and Logiroad).