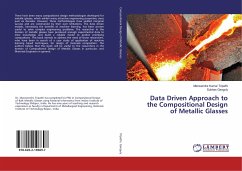
Data Driven Approach to the Compositional Design of Metallic Glasses
Versandkostenfrei!
Versandfertig in 6-10 Tagen
45,99 €
inkl. MwSt.
PAYBACK Punkte
23 °P sammeln!
There have been many compositional design methodologies developed for metallic glasses, which exhibit many attractive engineering properties, since past six decades. However, these methodologies have yielded marginal success and are constrained by their own limitations. The data driven models, harnessing the benefits of machine learning, has been proven useful to solve complex engineering problems. The researcher in the domain of metallic glasses have produced enough experimental data to mine knowledge and build a reliable model to predict promising compositions. This book intends to address t...
There have been many compositional design methodologies developed for metallic glasses, which exhibit many attractive engineering properties, since past six decades. However, these methodologies have yielded marginal success and are constrained by their own limitations. The data driven models, harnessing the benefits of machine learning, has been proven useful to solve complex engineering problems. The researcher in the domain of metallic glasses have produced enough experimental data to mine knowledge and build a reliable model to predict promising compositions. This book intends to address the need of those researchers, who have been in search of a case study of application of machine learning based techniques, for design of materials composition. The authors believe that this book will be useful to the researchers in the domain of Compositional Design of Metallic Glasses in particular, and Materials Engineers in general.