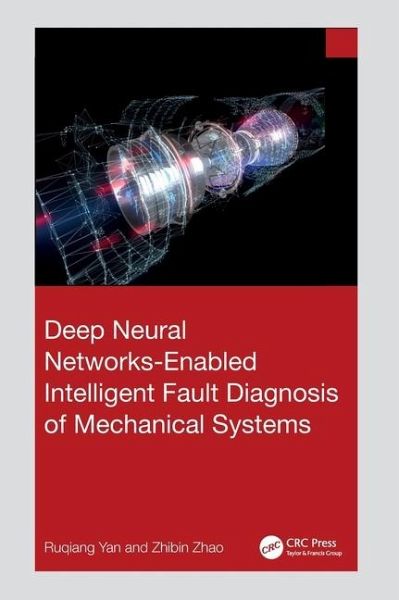
Deep Neural Networks-Enabled Intelligent Fault Diagnosis of Mechanical Systems
Versandkostenfrei!
Versandfertig in 6-10 Tagen
86,99 €
inkl. MwSt.
Weitere Ausgaben:
PAYBACK Punkte
43 °P sammeln!
The book aims to highlight the potential of deep learning (DL)-enabled methods in intelligent fault diagnosis (IFD), along with their benefits and contributions.The authors first introduce basic applications of DL-enabled IFD, including auto-encoders, deep belief networks, and convolutional neural networks. Advanced topics of DL-enabled IFD are also explored, such as data augmentation, multi-sensor fusion, unsupervised deep transfer learning, neural architecture search, self-supervised learning, and reinforcement learning. Aiming to revolutionize the nature of IFD, Deep Neural Networks-Enabled...
The book aims to highlight the potential of deep learning (DL)-enabled methods in intelligent fault diagnosis (IFD), along with their benefits and contributions.
The authors first introduce basic applications of DL-enabled IFD, including auto-encoders, deep belief networks, and convolutional neural networks. Advanced topics of DL-enabled IFD are also explored, such as data augmentation, multi-sensor fusion, unsupervised deep transfer learning, neural architecture search, self-supervised learning, and reinforcement learning. Aiming to revolutionize the nature of IFD, Deep Neural Networks-Enabled Intelligent Fault Diangosis of Mechanical Systems contributes to improved efficiency, safety, and reliability of mechanical systems in various industrial domains.
The book will appeal to academic researchers, practitioners, and students in the fields of intelligent fault diagnosis, prognostics and health management, and deep learning.
The authors first introduce basic applications of DL-enabled IFD, including auto-encoders, deep belief networks, and convolutional neural networks. Advanced topics of DL-enabled IFD are also explored, such as data augmentation, multi-sensor fusion, unsupervised deep transfer learning, neural architecture search, self-supervised learning, and reinforcement learning. Aiming to revolutionize the nature of IFD, Deep Neural Networks-Enabled Intelligent Fault Diangosis of Mechanical Systems contributes to improved efficiency, safety, and reliability of mechanical systems in various industrial domains.
The book will appeal to academic researchers, practitioners, and students in the fields of intelligent fault diagnosis, prognostics and health management, and deep learning.