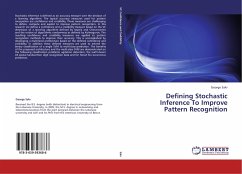
Defining Stochastic Inference To Improve Pattern Recognition
Versandkostenfrei!
Versandfertig in 6-10 Tagen
40,99 €
inkl. MwSt.
PAYBACK Punkte
20 °P sammeln!
Stochastic inference is defined as an accuracy measure over the decision of a learning algorithm. The typical accuracy measures used for pattern recognition are confidence and credibility. These measures are challenging to define, compute and exploit to improve pattern recognition. In this research we define a confidence and a credibility measure based on the VC dimension of a learning algorithm defined by Vapnik and Chervonenkis and the notion of algorithmic randomness as defined by Kolmogorov. The resulting confidence and credibility measures are applied to pattern recognition methods to imp...
Stochastic inference is defined as an accuracy measure over the decision of a learning algorithm. The typical accuracy measures used for pattern recognition are confidence and credibility. These measures are challenging to define, compute and exploit to improve pattern recognition. In this research we define a confidence and a credibility measure based on the VC dimension of a learning algorithm defined by Vapnik and Chervonenkis and the notion of algorithmic randomness as defined by Kolmogorov. The resulting confidence and credibility measures are applied to pattern recognition methods to improve their accuracy. This is accomplished by developing a multi-level architecture based on the defined confidence and credibility. In addition these defined measures are used to extend the binary classification of a single SVM to multi-class prediction. The benefits of the proposed architecture and the multi-class SVM are demonstrated on the following classification problems: agitation detection, the well known US postal handwritten digit recognition data and for forest fire occurrence prediction.