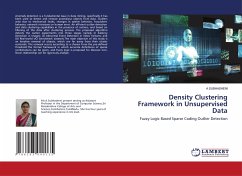
Density Clustering Framework in Unsupervised Data
Fuzzy Logic Based Sparse Coding Outlier Detection
Versandkostenfrei!
Versandfertig in 6-10 Tagen
26,99 €
inkl. MwSt.
PAYBACK Punkte
13 °P sammeln!
Anomaly detection is a fundamental issue in data mining, specifically it has been used to detect and remove anomalous objects from data. Outliers arise due to mechanical faults, changes in system behavior, fraudulent behavior, network intrusions or human error. An efficient outlier detection and data clustering capabilities in the presence of outliers, and based on filtering of the data after clustering process. The proposed algorithm detects the outlier experiments into three stages namely (i) Saliency Detection in Images; (ii) Abnormal Event Detection in Video Streams; and (iii) Real-world U...
Anomaly detection is a fundamental issue in data mining, specifically it has been used to detect and remove anomalous objects from data. Outliers arise due to mechanical faults, changes in system behavior, fraudulent behavior, network intrusions or human error. An efficient outlier detection and data clustering capabilities in the presence of outliers, and based on filtering of the data after clustering process. The proposed algorithm detects the outlier experiments into three stages namely (i) Saliency Detection in Images; (ii) Abnormal Event Detection in Video Streams; and (iii) Real-world UCI benchmark datasets.The main objective of this study is an iterative removal of objects, which are far away from their cluster centroids. The removal occurs according to a chosen from the pre-defined threshold.The formal framework in which accurate definitions of sparse combinations can be given, and Fuzzy logic is proposed for discover non-linear relationship can be rigorously analyze.