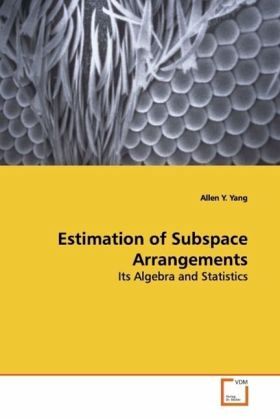
Estimation of Subspace Arrangements
Its Algebra and Statistics
Versandkostenfrei!
Versandfertig in 6-10 Tagen
32,99 €
inkl. MwSt.
PAYBACK Punkte
16 °P sammeln!
In the literature of computer vision and image processing, a fundamental problem in modeling visual data is that multivariate image or video data tend to be heterogeneous or multimodal. That is, subsets of the data may have significantly different geometric or statistical properties. Recently, subspace arrangements have become an increasingly popular class of mathematical objects to use for modeling multivariate mixed data that are (approximately) piecewise linear. A subspace arrangement is a union of multiple subspaces. Each subspace can be used to model a homogeneous subset of the data. In t...
In the literature of computer vision and image
processing, a fundamental problem in modeling visual
data is that multivariate image or video data tend to
be heterogeneous or multimodal. That is, subsets of
the data may have significantly different geometric
or statistical properties. Recently, subspace
arrangements have become an increasingly popular
class of mathematical objects to use for modeling
multivariate mixed data that are (approximately)
piecewise linear. A subspace arrangement is a union
of multiple subspaces. Each subspace can be used to
model a homogeneous subset of the data. In this work,
we study the problem of segmenting subspace
arrangements. Built on past extensive study of
subspace arrangements in algebraic geometry, we
propose a principled framework that summarizes
important algebraic properties and statistical facts
that are crucial for making the inference of subspace
arrangement models both efficient and robust, even
when the given data are corrupted with noise and/or
contaminated by outliers. The new solutions in many
ways improve and generalize extant methods for
modeling or clustering mixed data.
processing, a fundamental problem in modeling visual
data is that multivariate image or video data tend to
be heterogeneous or multimodal. That is, subsets of
the data may have significantly different geometric
or statistical properties. Recently, subspace
arrangements have become an increasingly popular
class of mathematical objects to use for modeling
multivariate mixed data that are (approximately)
piecewise linear. A subspace arrangement is a union
of multiple subspaces. Each subspace can be used to
model a homogeneous subset of the data. In this work,
we study the problem of segmenting subspace
arrangements. Built on past extensive study of
subspace arrangements in algebraic geometry, we
propose a principled framework that summarizes
important algebraic properties and statistical facts
that are crucial for making the inference of subspace
arrangement models both efficient and robust, even
when the given data are corrupted with noise and/or
contaminated by outliers. The new solutions in many
ways improve and generalize extant methods for
modeling or clustering mixed data.