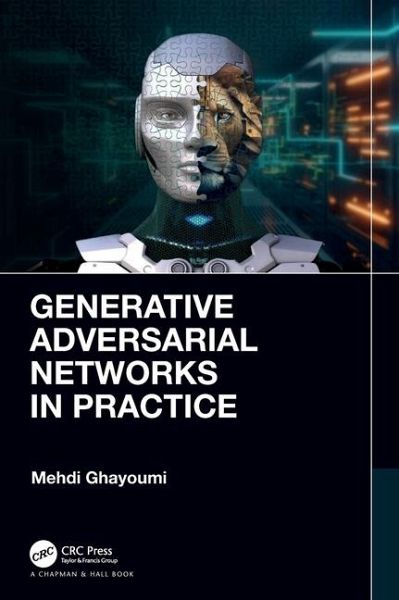
Generative Adversarial Networks in Practice
Versandkostenfrei!
Versandfertig in 1-2 Wochen
96,99 €
inkl. MwSt.
Weitere Ausgaben:
PAYBACK Punkte
48 °P sammeln!
Generative Adversarial Networks (GANs) in Practice is an all-inclusive resource that provides a solid foundation on GAN methodologies, their application to real-world projects, and their underlying mathematical and theoretical concepts.