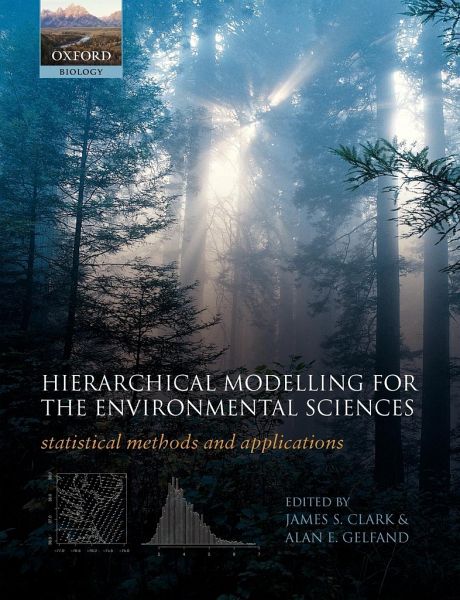
Hierarchical Modelling for the Environmental Sciences
Statistical Methods and Applications
Herausgegeben von Clark, James S.; Gelfand, Alan E.
Versandkostenfrei!
Versandfertig in 1-2 Wochen
97,99 €
inkl. MwSt.
PAYBACK Punkte
49 °P sammeln!
New statistical tools are changing the ways in which scientists analyze and interpret data and models. Many of these are emerging as a result of the wide availability of inexpensive, high speed computational power. In particular, hierarchical Bayes and Markov Chain Monte Carlo methods for analysis provide consistent framework for inference and prediction where information is heterogeneous and uncertain, processes are complex, and responses depend on scale. Nowhere are thesemethods more promising than in the environmental sciences. Models have developed rapidly, and there is now a requirement f...
New statistical tools are changing the ways in which scientists analyze and interpret data and models. Many of these are emerging as a result of the wide availability of inexpensive, high speed computational power. In particular, hierarchical Bayes and Markov Chain Monte Carlo methods for analysis provide consistent framework for inference and prediction where information is heterogeneous and uncertain, processes are complex, and responses depend on scale. Nowhere are these
methods more promising than in the environmental sciences. Models have developed rapidly, and there is now a requirement for a clear exposition of the methodology through to application for a range of environmental challenges.
methods more promising than in the environmental sciences. Models have developed rapidly, and there is now a requirement for a clear exposition of the methodology through to application for a range of environmental challenges.