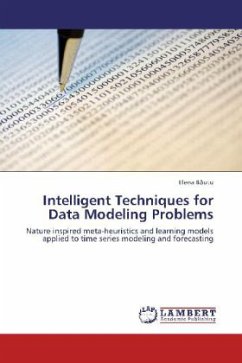
Intelligent Techniques for Data Modeling Problems
Nature inspired meta-heuristics and learning models applied to time series modeling and forecasting
Versandkostenfrei!
Versandfertig in 6-10 Tagen
51,99 €
inkl. MwSt.
PAYBACK Punkte
26 °P sammeln!
Supervised learning deals with the problem of discovering models from data as relationships between input and output attributes. Two types of models are distinguished: regression models (for continuous output attributes) and classification models (for discrete output attributes). This thesis addresses both regression and classification problems with an emphasis on new applications and on presenting improved evolutionary techniques. Such techniques include Gene Expression Programming (classical and its adaptive version), Genetic Programming, and the hypernetwork model of learning (classical and...
Supervised learning deals with the problem of discovering models from data as relationships between input and output attributes. Two types of models are distinguished: regression models (for continuous output attributes) and classification models (for discrete output attributes). This thesis addresses both regression and classification problems with an emphasis on new applications and on presenting improved evolutionary techniques. Such techniques include Gene Expression Programming (classical and its adaptive version), Genetic Programming, and the hypernetwork model of learning (classical and its evolutionary version). Such methods can be successfully applied to many problems from various domains. This thesis presents applications for symbolic regression for inverse problems, quantum circuit design, modeling of dynamic processes, and forecasting price movement.