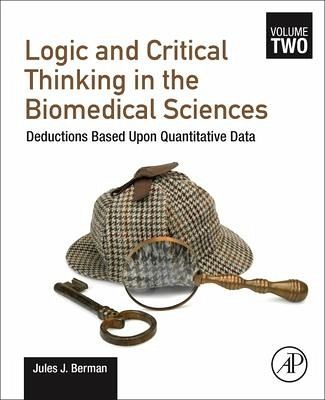
Logic and Critical Thinking in the Biomedical Sciences
Volume 2: Deductions Based Upon Quantitative Data
PAYBACK Punkte
47 °P sammeln!
All too often, individuals engaged in the biomedical sciences assume that numeric data must be left to the proper authorities (e.g., statisticians and data analysts) who are trained to apply sophisticated mathematical algorithms to sets of data. This is a terrible mistake. Individuals with keen observational skills, regardless of their mathematical training, are in the best position to draw correct inferences from their own data and to guide the subsequent implementation of robust, mathematical analyses. Volume 2 of Logic and Critical Thinking in the Biomedical Sciences provides readers with a...
All too often, individuals engaged in the biomedical sciences assume that numeric data must be left to the proper authorities (e.g., statisticians and data analysts) who are trained to apply sophisticated mathematical algorithms to sets of data. This is a terrible mistake. Individuals with keen observational skills, regardless of their mathematical training, are in the best position to draw correct inferences from their own data and to guide the subsequent implementation of robust, mathematical analyses. Volume 2 of Logic and Critical Thinking in the Biomedical Sciences provides readers with a repertoire of deductive non-mathematical methods that will help them draw useful inferences from their own data.Volumes 1 and 2 of Logic and Critical Thinking in the Biomedical Sciences are written for biomedical scientists and college-level students engaged in any of the life sciences, including bioinformatics and related data sciences.