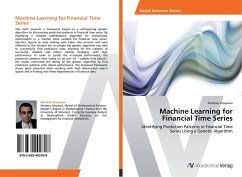
Machine Learning for Financial Time Series
Identifying Prediction Patterns in Financial Time Series Using a Genetic Algorithm
Versandkostenfrei!
Versandfertig in 6-10 Tagen
24,99 €
inkl. MwSt.
PAYBACK Punkte
12 °P sammeln!
This work presents a framework based on a self-learning genetic algorithm for discovering prediction patterns in financial time series. By modifying a complex mathematical algorithm for evolutionary optimization in a manner more suitable for financial time series, specifics typical to asset trading were taken into account and were reflected in the solution set. In-sample the genetic algorithm was able to successfully find prediction rules allowing for the creation of successful, feasible and robust trading strategies with high performance. In order to justify the in-sample performance, the pre...
This work presents a framework based on a self-learning genetic algorithm for discovering prediction patterns in financial time series. By modifying a complex mathematical algorithm for evolutionary optimization in a manner more suitable for financial time series, specifics typical to asset trading were taken into account and were reflected in the solution set. In-sample the genetic algorithm was able to successfully find prediction rules allowing for the creation of successful, feasible and robust trading strategies with high performance. In order to justify the in-sample performance, the prediction patterns were tested on an out of sample time period : the results confirmed the ability of the genetic algorithm to find prediction patterns with robust performance. The proposed framework shows great potential when working with high dimensional search spaces and in finding non-linear dependencies in financial data.