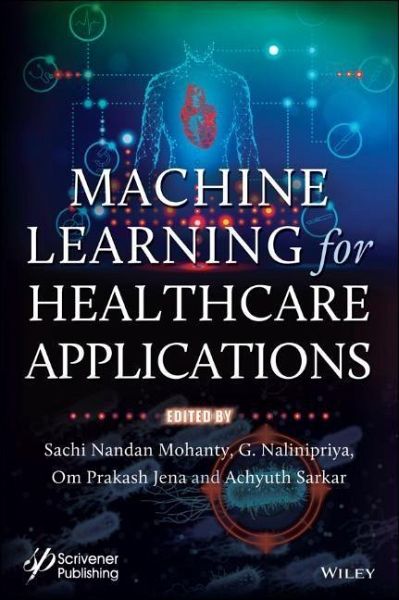
Machine Learning for Healthcare Applications
Versandkostenfrei!
Versandfertig in 2-4 Wochen
203,99 €
inkl. MwSt.
Weitere Ausgaben:
PAYBACK Punkte
102 °P sammeln!
When considering the idea of using machine learning in healthcare, it is a Herculean task to present the entire gamut of information in the field of intelligent systems. It is, therefore the objective of this book to keep the presentation narrow and intensive. This approach is distinct from others in that it presents detailed computer simulations for all models presented with explanations of the program code. It includes unique and distinctive chapters on disease diagnosis, telemedicine, medical imaging, smart health monitoring, social media healthcare, and machine learning for COVID-19. These...
When considering the idea of using machine learning in healthcare, it is a Herculean task to present the entire gamut of information in the field of intelligent systems. It is, therefore the objective of this book to keep the presentation narrow and intensive. This approach is distinct from others in that it presents detailed computer simulations for all models presented with explanations of the program code. It includes unique and distinctive chapters on disease diagnosis, telemedicine, medical imaging, smart health monitoring, social media healthcare, and machine learning for COVID-19. These chapters help develop a clear understanding of the working of an algorithm while strengthening logical thinking. In this environment, answering a single question may require accessing several data sources and calling on sophisticated analysis tools. While data integration is a dynamic research area in the database community, the specific needs of research have led to the development of numerous middleware systems that provide seamless data access in a result-driven environment.
Since this book is intended to be useful to a wide audience, students, researchers and scientists from both academia and industry may all benefit from this material. It contains a comprehensive description of issues for healthcare data management and an overview of existing systems, making it appropriate for introductory and instructional purposes. Prerequisites are minimal; the readers are expected to have basic knowledge of machine learning.
This book is divided into 22 real-time innovative chapters which provide a variety of application examples in different domains. These chapters illustrate why traditional approaches often fail to meet customers' needs. The presented approaches provide a comprehensive overview of current technology. Each of these chapters, which are written by the main inventors of the presented systems, specifies requirements and provides a description of both the chosen approach and its implementation. Because of the self-contained nature of these chapters, they may be read in any order. Each of the chapters use various technical terms which involve expertise in machine learning and computer science.
Since this book is intended to be useful to a wide audience, students, researchers and scientists from both academia and industry may all benefit from this material. It contains a comprehensive description of issues for healthcare data management and an overview of existing systems, making it appropriate for introductory and instructional purposes. Prerequisites are minimal; the readers are expected to have basic knowledge of machine learning.
This book is divided into 22 real-time innovative chapters which provide a variety of application examples in different domains. These chapters illustrate why traditional approaches often fail to meet customers' needs. The presented approaches provide a comprehensive overview of current technology. Each of these chapters, which are written by the main inventors of the presented systems, specifies requirements and provides a description of both the chosen approach and its implementation. Because of the self-contained nature of these chapters, they may be read in any order. Each of the chapters use various technical terms which involve expertise in machine learning and computer science.