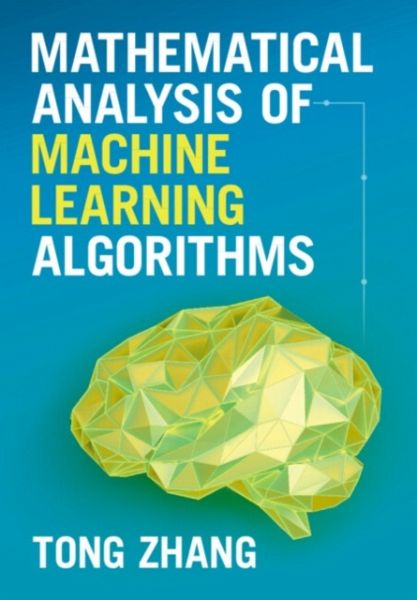
Mathematical Analysis of Machine Learning Algorithms
PAYBACK Punkte
21 °P sammeln!
This self-contained textbook introduces students and researchers of AI to the key mathematical concepts and techniques necessary to learn and analyze machine learning algorithms. Readers will gain the technical knowledge needed to understand research papers in theoretical machine learning, without much difficulty.