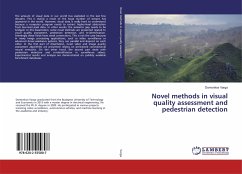
Novel methods in visual quality assessment and pedestrian detection
Versandkostenfrei!
Versandfertig in 6-10 Tagen
47,99 €
inkl. MwSt.
PAYBACK Punkte
24 °P sammeln!
The amount of visual data in our world has exploded in the last few decades. This is mainly a result of the huge number of sensors has appeared in the world. However, visual data is really hard to understand because a computer program needs to extract higher-level abstraction from low-level pixel data. In other words, the semantic gap needs to be bridged. In this dissertation, some novel methods are presented related to visual quality assessment, pedestrian detection, and re-identification. Seemingly these fields have weak connections. This is not the case because in many image processing appl...
The amount of visual data in our world has exploded in the last few decades. This is mainly a result of the huge number of sensors has appeared in the world. However, visual data is really hard to understand because a computer program needs to extract higher-level abstraction from low-level pixel data. In other words, the semantic gap needs to be bridged. In this dissertation, some novel methods are presented related to visual quality assessment, pedestrian detection, and re-identification. Seemingly these fields have weak connections. This is not the case because in many image processing applications, such as video surveillance or advanced driver-assistance systems, they run parallel and depend on each other. In the first part of dissertation, novel video and image quality assessment algorithms are presented relying on pretrained convolutional neural networks. On the other hand, the second part deals with pedestrian detection and re-identification in surveillance videos. Experimental results and analysis are demonstrated on publicly available benchmark databases.