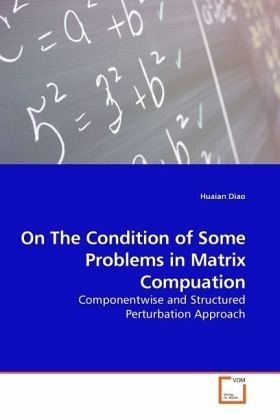
On The Condition of Some Problems in Matrix Compuation
Componentwise and Structured Perturbation Approach
Versandkostenfrei!
Versandfertig in 6-10 Tagen
32,99 €
inkl. MwSt.
PAYBACK Punkte
16 °P sammeln!
In numerical analysis, the condition number associated with a problem is a measure of that problem's amenability to digital computation, that is, how numerically well-posed the problem is. A problem with a low condition number is said to be well-conditioned, while a problem with a high condition number is said to be ill-conditioned. Classical condition numbers are normwise: they measure the size of both input perturbations and output errors using some norms. To take into account the relative of each data component, and, in particular, a possible data sparseness, componentwise condition numbers...
In numerical analysis, the condition number associated with a problem is a measure of that problem's amenability to digital computation, that is, how numerically well-posed the problem is. A problem with a low condition number is said to be well-conditioned, while a problem with a high condition number is said to be ill-conditioned. Classical condition numbers are normwise: they measure the size of both input perturbations and output errors using some norms. To take into account the relative of each data component, and, in particular, a possible data sparseness, componentwise condition numbers have been increasingly considered. These are mostly of two kinds: mixed and componentwise. In this book, we give explicit expressions, computable from the data, for the mixed and componentwise condition numbers for some problems in matrix computation, such as Moore- Penrose inverse, structured and unstructured linear least squares problems, structured and unstructured eigenvalue problems and smoothed analysis of some normwise condition numbers.