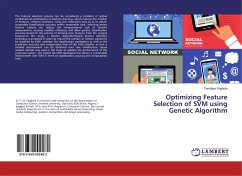
Optimizing Feature Selection of SVM using Genetic Algorithm
Versandkostenfrei!
Versandfertig in 6-10 Tagen
33,99 €
inkl. MwSt.
PAYBACK Punkte
17 °P sammeln!
The feature selection process can be considered a problem of global combinatorial optimization in machine learning, which reduces the number of features, removes irrelevant, noisy and redundant data so as to obtain acceptable classification accuracy within reasonable time. Selecting better feature subsets can reduce the computational cost of feature measurement, increase classifier efficiency, and allow greater classification accuracy based on the process of deriving new features from the original features.In this study, a Genetic Algorithm-based feature selection technique is proposed in orde...
The feature selection process can be considered a problem of global combinatorial optimization in machine learning, which reduces the number of features, removes irrelevant, noisy and redundant data so as to obtain acceptable classification accuracy within reasonable time. Selecting better feature subsets can reduce the computational cost of feature measurement, increase classifier efficiency, and allow greater classification accuracy based on the process of deriving new features from the original features.In this study, a Genetic Algorithm-based feature selection technique is proposed in order to reduce the number of feature subsets to be classified by SVM, optimize the classification parameters as well as the prediction accuracy and computation time of the SVM classifier so that a marked improvement can be obtained over raw classification. Spam assassin dataset was used in this study to validate the performance of the proposed system. The hybrid GA-SVM developed has shown a remarkable improvement over SVM in terms of classification accuracy and computation time.