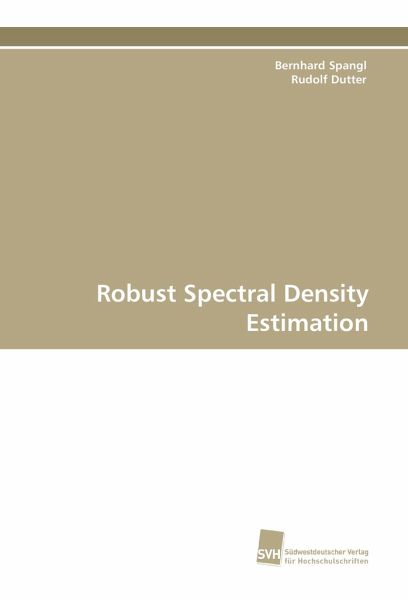
Robust Spectral Density Estimation
Versandkostenfrei!
Versandfertig in 6-10 Tagen
69,90 €
inkl. MwSt.
PAYBACK Punkte
0 °P sammeln!
We deal with robust spectral density estimation and its application to the analysis of heart rate variability. As classical spectral density estimators are sensitive to outlying observations, robustness is an issue. Hence, we focus on the problem of estimating the spectral density function robustly and present different methods, existing and new ones, that are resistant to outliers. In order to get a reliable estimate of the spectral density function, that is insensitive to outlying observations, it turned out that cleaning the time series in a robust way first and calculating the spectral den...
We deal with robust spectral density estimation and its application to the analysis of heart rate variability. As classical spectral density estimators are sensitive to outlying observations, robustness is an issue. Hence, we focus on the problem of estimating the spectral density function robustly and present different methods, existing and new ones, that are resistant to outliers. In order to get a reliable estimate of the spectral density function, that is insensitive to outlying observations, it turned out that cleaning the time series in a robust way first and calculating the spectral density function afterwards leads to encouraging results. The data-cleaning operation wherein the robustness is introduced, is accomplished by a robustified version of the Kalman filter. In addition a new multivariate approximate conditional-mean type filter for state-space models is proposed. All presented methods are implemented in the open source language R and compared by extensive simulation studies. The most competitive method is also applied to actual heart rate variability data of diabetic patients with different degrees of cardiovascular autonomic neuropathy.