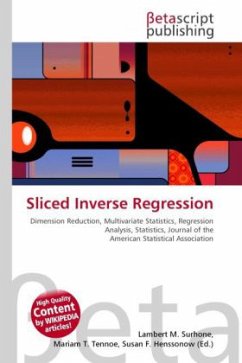
Sliced Inverse Regression
Versandkostenfrei!
Versandfertig in 6-10 Tagen
22,99 €
inkl. MwSt.
PAYBACK Punkte
11 °P sammeln!
High Quality Content by WIKIPEDIA articles! Sliced inverse regression (SIR) is a tool for dimension reduction in the field of multivariate statistics. In statistics, regression analysis is a popular way of studying the relationship between a response variable y and its explanatory variable underline{x}, which is a p-dimensional vector. There are several approaches which come under the term of regression. We know parametric methods, such as multiple linear regression, but also non-parametric techniques, such as local smoothing. If we have high-dimensional data, the number of observations needed...
High Quality Content by WIKIPEDIA articles! Sliced inverse regression (SIR) is a tool for dimension reduction in the field of multivariate statistics. In statistics, regression analysis is a popular way of studying the relationship between a response variable y and its explanatory variable underline{x}, which is a p-dimensional vector. There are several approaches which come under the term of regression. We know parametric methods, such as multiple linear regression, but also non-parametric techniques, such as local smoothing. If we have high-dimensional data, the number of observations needed to use local smoothing methods escalates exponentially. Therefore we need a tool for dimension reduction, which reveals us the most important directions of the data, on which it can be projected without losing, in the best case, any information. SIR uses the inverse regression curve, E(underline{x}, ,y), which falls into the effective dimension reducing space under certain conditions, to perform a weighted principal component analysis, with which one identifies the effective dimension reducing directions.