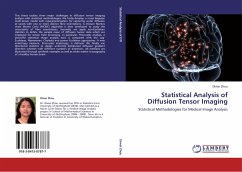
Statistical Analysis of Diffusion Tensor Imaging
Statistical Methodologies for Medical Image Analysis
Versandkostenfrei!
Versandfertig in 6-10 Tagen
44,99 €
inkl. MwSt.
PAYBACK Punkte
22 °P sammeln!
This thesis tackles three major challenges in diffusion tensor imaging analysis with statistical methodologies. We firstly develop a novel Bayesian multi-tensor model with reparameterisation for capturing water diffusion at voxels with one or more distinct fibre orientations. A mixture Markov chain Monte Carlo (MCMC) algorithm is then developed to study the uncertainty of fibre orientations. Secondly, we apply non-Euclidean statistics to define the sample mean of diffusion tensor data which are employed for tensor field processing. In particular, Procrustes analysis, a powerful statistical sha...
This thesis tackles three major challenges in diffusion tensor imaging analysis with statistical methodologies. We firstly develop a novel Bayesian multi-tensor model with reparameterisation for capturing water diffusion at voxels with one or more distinct fibre orientations. A mixture Markov chain Monte Carlo (MCMC) algorithm is then developed to study the uncertainty of fibre orientations. Secondly, we apply non-Euclidean statistics to define the sample mean of diffusion tensor data which are employed for tensor field processing. In particular, Procrustes analysis, a powerful statistical shape analysis tool, is compared with the Log-Euclidean, Riemannian, Cholesky and power Euclidean approaches. A new anisotropy measure, Procrustes anisotropy, is defined. We finally use directional statistics to design uniformly distributed diffusion gradient direction schemes with different numbers of directions. All methods are illustrated through synthetic examples as well as white matter tractography of a healthy human brain.