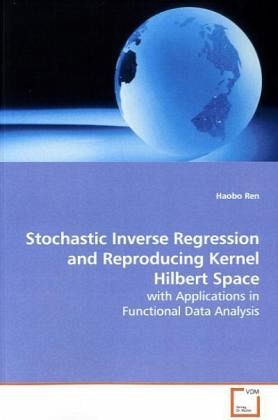
Stochastic Inverse Regression and Reproducing Kernel Hilbert Space
with Applications in Functional Data Analysis
Versandkostenfrei!
Versandfertig in 6-10 Tagen
32,99 €
inkl. MwSt.
PAYBACK Punkte
16 °P sammeln!
The basic philosophy of Functional Data Analysis (FDA) is to think of the observed data functions as elements of a possibly infinite-dimensional function space. Most of the current research topics on FDA focus on advancing theoretical tools and extending existing multivariate techniques to accommodate the infinite-dimensional nature of data. This monograph reports contributions on both fronts, where a unifying inverse regression theory for both themultivariate setting and functional data from a Reproducing Kernel Hilbert Space (RKHS) prospective is developed.We proposed a stochastic multiple-i...
The basic philosophy of Functional Data Analysis
(FDA) is to think of the observed data functions as
elements of a possibly infinite-dimensional function
space. Most of the current research topics on FDA
focus on advancing theoretical tools and extending
existing multivariate techniques to accommodate the
infinite-dimensional nature of data. This monograph
reports contributions on both fronts, where a
unifying inverse regression theory for both the
multivariate setting and functional data from a
Reproducing Kernel Hilbert Space (RKHS) prospective
is developed.
We proposed a stochastic multiple-index model, two
RKHS-related inverse regression procedures, a
``slicing'' approach and a kernel approach, as well
as an asymptotic theory were introduced to the
statistical framework. Some general computational
issues of FDA were discussed, Some
general computational issues of FDA were discussed,
which led to smoothed versions of the stochastic
inverse regression methods.
(FDA) is to think of the observed data functions as
elements of a possibly infinite-dimensional function
space. Most of the current research topics on FDA
focus on advancing theoretical tools and extending
existing multivariate techniques to accommodate the
infinite-dimensional nature of data. This monograph
reports contributions on both fronts, where a
unifying inverse regression theory for both the
multivariate setting and functional data from a
Reproducing Kernel Hilbert Space (RKHS) prospective
is developed.
We proposed a stochastic multiple-index model, two
RKHS-related inverse regression procedures, a
``slicing'' approach and a kernel approach, as well
as an asymptotic theory were introduced to the
statistical framework. Some general computational
issues of FDA were discussed, Some
general computational issues of FDA were discussed,
which led to smoothed versions of the stochastic
inverse regression methods.