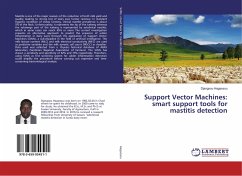
Support Vector Machines: smart support tools for mastitis detection
Versandkostenfrei!
Versandfertig in 6-10 Tagen
22,99 €
inkl. MwSt.
PAYBACK Punkte
11 °P sammeln!
Mastitis is one of the major reasons of the reduction of both milk yield and quality, leading to strong lost of dairy ewe farmer revenue. In standard hygienic condition of sheep farming, clinical mastitis prevalence is about 5% of the flock. Unfortunately, it represents the tip of the iceberg whereas the submerge part of the iceberg is represented by subclinical mastitis, which in some cases can reach 65% or more. The current investigation presents an alternative approach to predict the presence of udder inflammation in dairy ewes through the application of Support Vector Machines (SVMs), a su...
Mastitis is one of the major reasons of the reduction of both milk yield and quality, leading to strong lost of dairy ewe farmer revenue. In standard hygienic condition of sheep farming, clinical mastitis prevalence is about 5% of the flock. Unfortunately, it represents the tip of the iceberg whereas the submerge part of the iceberg is represented by subclinical mastitis, which in some cases can reach 65% or more. The current investigation presents an alternative approach to predict the presence of udder inflammation in dairy ewes through the application of Support Vector Machines (SVMs), a sub-discipline in the field of artificial intelligence. The milk lactose content (MLC) and milk electrical conductivity (MEC) are used as predictive variables and the milk somatic cell count (MSCC) as classifier. Data used was collected from a 10-years historical database of ARAS laboratory (Sardinian Regional Association of Farmers). The SVMs has shown a sensitivity and specificity of 62% and75% respectively. Therefore, using SVMs as first screening system for udder inflammation detection could simplify the procedure before carrying out expensive and time-consuming bacteriological analysis.