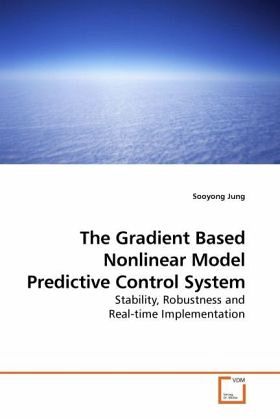
The Gradient Based Nonlinear Model Predictive Control System
Stability, Robustness and Real-time Implementation
Versandkostenfrei!
Versandfertig in 6-10 Tagen
38,99 €
inkl. MwSt.
PAYBACK Punkte
19 °P sammeln!
A class of nonlinear model predictive control(NMPC) law based on gradient-based iteration is analyzed and implemented real-time in this book. This NMPC law takes only a finite number of Newton steps in each sampling period instead of solving the complete optimal control problem. The key attribute of the NMPC algorithmused here is that it only seeks to reduce the error at the end of the prediction horizon rather than tries to find the optimal solution. This reduces the computation load and allows for real-time implementation. The stability is shown for a class of discrete-time control-affine sy...
A class of nonlinear model predictive control(NMPC) law based on gradient-based iteration is analyzed and implemented real-time in this book. This NMPC law takes only a finite number of Newton steps in each sampling period instead of solving the complete optimal control problem. The key attribute of the NMPC algorithmused here is that it only seeks to reduce the error at the end of the prediction horizon rather than tries to find the optimal solution. This reduces the computation load and allows for real-time implementation. The stability is shown for a class of discrete-time control-affine system that the NMPC has some inherent robustness property with respect to external disturbances and model error. This property follows from the exponential convergence of the predicted state error. The robustness of this NMPC is analyzed and quantified with uncertainties. In addition to simulation examples, the NMPC algorithm is also applied to the swing-up control experiment of a rotary inverted pendulum. We also discuss the implementation strategy, state estimation issue, and experimental results.