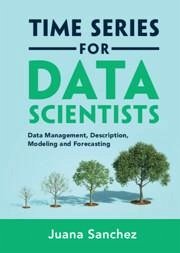
Time Series for Data Scientists
Data Management, Description, Modeling and Forecasting
PAYBACK Punkte
42 °P sammeln!
Learn by doing with this guide to classical and contemporary machine learning approaches to time series data analysis. With data sets, commented R programs, case studies and quizzes, this is an essential and accessible resource for undergraduate and graduate students in statistics and data science, and researchers in data-rich disciplines.