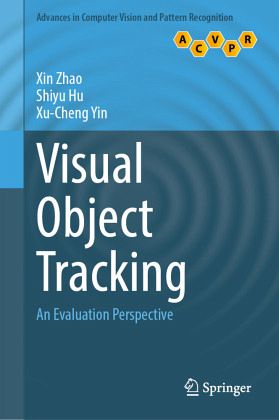
Visual Object Tracking
An Evaluation Perspective
PAYBACK Punkte
69 °P sammeln!
This book delves into visual object tracking (VOT), a fundamental aspect of computer vision crucial for replicating human dynamic vision, with applications ranging from self-driving vehicles to surveillance systems. Despite significant strides propelled by deep learning, challenges such as target deformation and motion persist, exposing a disparity between cutting-edge VOT systems and human performance. This observation underscores the necessity to thoroughly scrutinize and enhance evaluation methodologies within VOT research.Hence, the primary objective of this book is to equip readers with e...
This book delves into visual object tracking (VOT), a fundamental aspect of computer vision crucial for replicating human dynamic vision, with applications ranging from self-driving vehicles to surveillance systems. Despite significant strides propelled by deep learning, challenges such as target deformation and motion persist, exposing a disparity between cutting-edge VOT systems and human performance. This observation underscores the necessity to thoroughly scrutinize and enhance evaluation methodologies within VOT research.
Hence, the primary objective of this book is to equip readers with essential insights into dynamic visual tasks encapsulated by VOT. Beginning with the elucidation of task definitions, it integrates interdisciplinary perspectives on evaluation techniques. The book is organized into five parts, tracing the evolution of VOT from perceptual to cognitive intelligence, exploring the experimental frameworks utilized in assessments, analyzing the various agents involved, including tracking algorithms and human visual tracking, and dissecting evaluation mechanisms through both machine machine and human machine comparisons. Furthermore, it examines the trend toward crafting more human-like task definitions and comprehensive evaluation frameworks to effectively gauge machine intelligence.
This book serves as a roadmap for researchers aiming to grasp the bottlenecks in VOT capabilities and comprehend the gaps between current methodologies and human abilities, all geared toward advancing algorithmic intelligence. It also delves into the realm of data-centric AI, emphasizing the pivotal role of high-quality datasets and evaluation systems in the age of large language models (LLMs). Such systems are indispensable for training AI models while ensuring their safety and reliability. Utilizing VOT as a case study, the book offers detailed insights into these facets of data-centric AI research. Designed to cater to readers with foundational knowledge in computer vision, it employs diagrams and examples to facilitate comprehension, providing essential groundwork for understanding key technical components.
Hence, the primary objective of this book is to equip readers with essential insights into dynamic visual tasks encapsulated by VOT. Beginning with the elucidation of task definitions, it integrates interdisciplinary perspectives on evaluation techniques. The book is organized into five parts, tracing the evolution of VOT from perceptual to cognitive intelligence, exploring the experimental frameworks utilized in assessments, analyzing the various agents involved, including tracking algorithms and human visual tracking, and dissecting evaluation mechanisms through both machine machine and human machine comparisons. Furthermore, it examines the trend toward crafting more human-like task definitions and comprehensive evaluation frameworks to effectively gauge machine intelligence.
This book serves as a roadmap for researchers aiming to grasp the bottlenecks in VOT capabilities and comprehend the gaps between current methodologies and human abilities, all geared toward advancing algorithmic intelligence. It also delves into the realm of data-centric AI, emphasizing the pivotal role of high-quality datasets and evaluation systems in the age of large language models (LLMs). Such systems are indispensable for training AI models while ensuring their safety and reliability. Utilizing VOT as a case study, the book offers detailed insights into these facets of data-centric AI research. Designed to cater to readers with foundational knowledge in computer vision, it employs diagrams and examples to facilitate comprehension, providing essential groundwork for understanding key technical components.