AI, Machine Learning and Deep Learning (eBook, ePUB)
A Security Perspective
Redaktion: Hu, Fei; Hei, Xiali
52,95 €
52,95 €
inkl. MwSt.
Sofort per Download lieferbar
26 °P sammeln
52,95 €
Als Download kaufen
52,95 €
inkl. MwSt.
Sofort per Download lieferbar
26 °P sammeln
Jetzt verschenken
Alle Infos zum eBook verschenken
52,95 €
inkl. MwSt.
Sofort per Download lieferbar
Alle Infos zum eBook verschenken
26 °P sammeln
AI, Machine Learning and Deep Learning (eBook, ePUB)
A Security Perspective
Redaktion: Hu, Fei; Hei, Xiali
- Format: ePub
- Merkliste
- Auf die Merkliste
- Bewerten Bewerten
- Teilen
- Produkt teilen
- Produkterinnerung
- Produkterinnerung
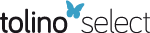
Bitte loggen Sie sich zunächst in Ihr Kundenkonto ein oder registrieren Sie sich bei
bücher.de, um das eBook-Abo tolino select nutzen zu können.
Hier können Sie sich einloggen
Hier können Sie sich einloggen
Sie sind bereits eingeloggt. Klicken Sie auf 2. tolino select Abo, um fortzufahren.
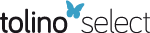
Bitte loggen Sie sich zunächst in Ihr Kundenkonto ein oder registrieren Sie sich bei bücher.de, um das eBook-Abo tolino select nutzen zu können.
Today AI and Machine/Deep Learning have become the hottest areas in the information technology. This book aims to provide a complete picture on the challenges and solutions to the security issues in various applications. It explains how different attacks can occur in advanced AI tools and the challenges of overcoming those attacks.
- Geräte: eReader
- ohne Kopierschutz
- eBook Hilfe
- Größe: 10.28MB
Today AI and Machine/Deep Learning have become the hottest areas in the information technology. This book aims to provide a complete picture on the challenges and solutions to the security issues in various applications. It explains how different attacks can occur in advanced AI tools and the challenges of overcoming those attacks.
Dieser Download kann aus rechtlichen Gründen nur mit Rechnungsadresse in A, B, BG, CY, CZ, D, DK, EW, E, FIN, F, GR, HR, H, IRL, I, LT, L, LR, M, NL, PL, P, R, S, SLO, SK ausgeliefert werden.
Produktdetails
- Produktdetails
- Verlag: Taylor & Francis
- Seitenzahl: 346
- Erscheinungstermin: 5. Juni 2023
- Englisch
- ISBN-13: 9781000878899
- Artikelnr.: 67694800
- Verlag: Taylor & Francis
- Seitenzahl: 346
- Erscheinungstermin: 5. Juni 2023
- Englisch
- ISBN-13: 9781000878899
- Artikelnr.: 67694800
- Herstellerkennzeichnung Die Herstellerinformationen sind derzeit nicht verfügbar.
Dr. Fei Hu is a professor in the department of Electrical and Computer Engineering at the University of Alabama. He has published over 10 technical books with CRC press. His research focus includes cyber security and networking. He obtained his Ph.D. degrees at Tongji University (Shanghai, China) in the field of Signal Processing (in 1999), and at Clarkson University (New York, USA) in Electrical and Computer Engineering (in 2002). He has published over 200 journal/conference papers and books. Dr. Hu's research has been supported by U.S. National Science Foundation, Cisco, Sprint, and other sources. He won the school's President's Faculty Research Award (<1% faculty were awarded each year) in 2020. Dr. Xiali (Sharon) Hei is an assistant professor in the School of Computing and Informatics at the University of Louisiana at Lafayette. Her research focus is cyber and physical security. Prior to joining the University of Louisiana at Lafayette, she was an assistant professor at Delaware State University from 2015-2017 and Frostburg State University 2014-2015. Sharon received his Ph.D. in computer science from Temple University in 2014, focusing on computer security.
Part I. Secure AI/ML Systems: Attack Models
1. Machine Learning Attack Models, 2. Adversarial Machine Learning: A New Threat Paradigm for Next-generation Wireless Communications, 3. Threat of Adversarial Attacks to Deep Learning: A Survey, 4. Attack Models for Collaborative Deep Learning, 5. Attacks on Deep Reinforcement Learning Systems: A Tutorial, 6. Trust and Security of Deep Reinforcement Learning, 7. IoT Threat Modeling using Bayesian Networks
Part II. Secure AI/ML Systems: Defenses
8. Survey of Machine Learning Defense Strategies, 9. Defenses Against Deep Learning Attacks, 10. Defensive Schemes for Cyber Security of Deep Reinforcement Learning, 11. Adversarial Attacks on Machine Learning Models in Cyber-Physical Systems, 12. Federated Learning and Blockchain: An Opportunity for Artificial Intelligence with Data Regulation
Part III. Using AI/ML Algorithms for Cyber Security
13. Using Machine Learning for Cyber Security: Overview, 14. Performance of Machine Learning and Big Data Analytics Paradigms in Cyber Security, 15. Using ML and DL Algorithms for Intrusion Detection in Industrial Internet of Things.
Part IV. Applications
16. On Detecting Interest Flooding Attacks in Named Data Networking (NDN)-based IoT Searches, 17. Attack on Fraud Detection Systems in Online Banking Using Generative Adversarial Networks, 18. An Artificial Intelligence-assisted Security Analysis of Smart Healthcare Systems, 19. A User-centric Focus for Detecting Phishing Emails
1. Machine Learning Attack Models, 2. Adversarial Machine Learning: A New Threat Paradigm for Next-generation Wireless Communications, 3. Threat of Adversarial Attacks to Deep Learning: A Survey, 4. Attack Models for Collaborative Deep Learning, 5. Attacks on Deep Reinforcement Learning Systems: A Tutorial, 6. Trust and Security of Deep Reinforcement Learning, 7. IoT Threat Modeling using Bayesian Networks
Part II. Secure AI/ML Systems: Defenses
8. Survey of Machine Learning Defense Strategies, 9. Defenses Against Deep Learning Attacks, 10. Defensive Schemes for Cyber Security of Deep Reinforcement Learning, 11. Adversarial Attacks on Machine Learning Models in Cyber-Physical Systems, 12. Federated Learning and Blockchain: An Opportunity for Artificial Intelligence with Data Regulation
Part III. Using AI/ML Algorithms for Cyber Security
13. Using Machine Learning for Cyber Security: Overview, 14. Performance of Machine Learning and Big Data Analytics Paradigms in Cyber Security, 15. Using ML and DL Algorithms for Intrusion Detection in Industrial Internet of Things.
Part IV. Applications
16. On Detecting Interest Flooding Attacks in Named Data Networking (NDN)-based IoT Searches, 17. Attack on Fraud Detection Systems in Online Banking Using Generative Adversarial Networks, 18. An Artificial Intelligence-assisted Security Analysis of Smart Healthcare Systems, 19. A User-centric Focus for Detecting Phishing Emails
Part I. Secure AI/ML Systems: Attack Models
1. Machine Learning Attack Models, 2. Adversarial Machine Learning: A New Threat Paradigm for Next-generation Wireless Communications, 3. Threat of Adversarial Attacks to Deep Learning: A Survey, 4. Attack Models for Collaborative Deep Learning, 5. Attacks on Deep Reinforcement Learning Systems: A Tutorial, 6. Trust and Security of Deep Reinforcement Learning, 7. IoT Threat Modeling using Bayesian Networks
Part II. Secure AI/ML Systems: Defenses
8. Survey of Machine Learning Defense Strategies, 9. Defenses Against Deep Learning Attacks, 10. Defensive Schemes for Cyber Security of Deep Reinforcement Learning, 11. Adversarial Attacks on Machine Learning Models in Cyber-Physical Systems, 12. Federated Learning and Blockchain: An Opportunity for Artificial Intelligence with Data Regulation
Part III. Using AI/ML Algorithms for Cyber Security
13. Using Machine Learning for Cyber Security: Overview, 14. Performance of Machine Learning and Big Data Analytics Paradigms in Cyber Security, 15. Using ML and DL Algorithms for Intrusion Detection in Industrial Internet of Things.
Part IV. Applications
16. On Detecting Interest Flooding Attacks in Named Data Networking (NDN)-based IoT Searches, 17. Attack on Fraud Detection Systems in Online Banking Using Generative Adversarial Networks, 18. An Artificial Intelligence-assisted Security Analysis of Smart Healthcare Systems, 19. A User-centric Focus for Detecting Phishing Emails
1. Machine Learning Attack Models, 2. Adversarial Machine Learning: A New Threat Paradigm for Next-generation Wireless Communications, 3. Threat of Adversarial Attacks to Deep Learning: A Survey, 4. Attack Models for Collaborative Deep Learning, 5. Attacks on Deep Reinforcement Learning Systems: A Tutorial, 6. Trust and Security of Deep Reinforcement Learning, 7. IoT Threat Modeling using Bayesian Networks
Part II. Secure AI/ML Systems: Defenses
8. Survey of Machine Learning Defense Strategies, 9. Defenses Against Deep Learning Attacks, 10. Defensive Schemes for Cyber Security of Deep Reinforcement Learning, 11. Adversarial Attacks on Machine Learning Models in Cyber-Physical Systems, 12. Federated Learning and Blockchain: An Opportunity for Artificial Intelligence with Data Regulation
Part III. Using AI/ML Algorithms for Cyber Security
13. Using Machine Learning for Cyber Security: Overview, 14. Performance of Machine Learning and Big Data Analytics Paradigms in Cyber Security, 15. Using ML and DL Algorithms for Intrusion Detection in Industrial Internet of Things.
Part IV. Applications
16. On Detecting Interest Flooding Attacks in Named Data Networking (NDN)-based IoT Searches, 17. Attack on Fraud Detection Systems in Online Banking Using Generative Adversarial Networks, 18. An Artificial Intelligence-assisted Security Analysis of Smart Healthcare Systems, 19. A User-centric Focus for Detecting Phishing Emails