Algorithmic Learning Theory (eBook, PDF)
16th International Conference, ALT 2005, Singapore, October 8-11, 2005, Proceedings
Redaktion: Jain, Sanjay; Tomita, Etsuji; Simon, Hans Ulrich
40,95 €
40,95 €
inkl. MwSt.
Sofort per Download lieferbar
20 °P sammeln
40,95 €
Als Download kaufen
40,95 €
inkl. MwSt.
Sofort per Download lieferbar
20 °P sammeln
Jetzt verschenken
Alle Infos zum eBook verschenken
40,95 €
inkl. MwSt.
Sofort per Download lieferbar
Alle Infos zum eBook verschenken
20 °P sammeln
Algorithmic Learning Theory (eBook, PDF)
16th International Conference, ALT 2005, Singapore, October 8-11, 2005, Proceedings
Redaktion: Jain, Sanjay; Tomita, Etsuji; Simon, Hans Ulrich
- Format: PDF
- Merkliste
- Auf die Merkliste
- Bewerten Bewerten
- Teilen
- Produkt teilen
- Produkterinnerung
- Produkterinnerung
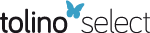
Bitte loggen Sie sich zunächst in Ihr Kundenkonto ein oder registrieren Sie sich bei
bücher.de, um das eBook-Abo tolino select nutzen zu können.
Hier können Sie sich einloggen
Hier können Sie sich einloggen
Sie sind bereits eingeloggt. Klicken Sie auf 2. tolino select Abo, um fortzufahren.
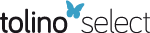
Bitte loggen Sie sich zunächst in Ihr Kundenkonto ein oder registrieren Sie sich bei bücher.de, um das eBook-Abo tolino select nutzen zu können.
- Geräte: PC
- ohne Kopierschutz
- eBook Hilfe
- Größe: 6.07MB
Andere Kunden interessierten sich auch für
- Learning Theory (eBook, PDF)73,95 €
- Machine Learning Challenges (eBook, PDF)40,95 €
- Learning Theory (eBook, PDF)73,95 €
- Algorithmic Learning Theory (eBook, PDF)40,95 €
- Computational Learning Theory (eBook, PDF)40,95 €
- Algorithmic Learning Theory (eBook, PDF)40,95 €
- Advances in Artificial Intelligence - IBERAMIA-SBIA 2006 (eBook, PDF)73,95 €
-
-
-
Produktdetails
- Verlag: Springer Berlin Heidelberg
- Seitenzahl: 491
- Erscheinungstermin: 11. Oktober 2005
- Englisch
- ISBN-13: 9783540316961
- Artikelnr.: 44132273
Dieser Download kann aus rechtlichen Gründen nur mit Rechnungsadresse in A, B, BG, CY, CZ, D, DK, EW, E, FIN, F, GR, HR, H, IRL, I, LT, L, LR, M, NL, PL, P, R, S, SLO, SK ausgeliefert werden.
Hinweis: Dieser Artikel kann nur an eine deutsche Lieferadresse ausgeliefert werden.
- Herstellerkennzeichnung Die Herstellerinformationen sind derzeit nicht verfügbar.
Sanjay Jain, National University of Singapore / Hans Ulrich Simon, Ruhr-Universität Bochum, Germany / Etsuji Tomita, The University of Electro-Communications, Tokyo, Japan
Editors' Introduction.- Editors' Introduction.- Invited Papers.- Invention and Artificial Intelligence.- The Arrowsmith Project: 2005 Status Report.- The Robot Scientist Project.- Algorithms and Software for Collaborative Discovery from Autonomous, Semantically Heterogeneous, Distributed Information Sources.- Training Support Vector Machines via SMO-Type Decomposition Methods.- Kernel-Based Learning.- Measuring Statistical Dependence with Hilbert-Schmidt Norms.- An Analysis of the Anti-learning Phenomenon for the Class Symmetric Polyhedron.- Learning Causal Structures Based on Markov Equivalence Class.- Stochastic Complexity for Mixture of Exponential Families in Variational Bayes.- ACME: An Associative Classifier Based on Maximum Entropy Principle.- Constructing Multiclass Learners from Binary Learners: A Simple Black-Box Analysis of the Generalization Errors.- On Computability of Pattern Recognition Problems.- PAC-Learnability of Probabilistic Deterministic Finite State Automata in Terms of Variation Distance.- Learnability of Probabilistic Automata via Oracles.- Learning Attribute-Efficiently with Corrupt Oracles.- Learning DNF by Statistical and Proper Distance Queries Under the Uniform Distribution.- Learning of Elementary Formal Systems with Two Clauses Using Queries.- Gold-Style and Query Learning Under Various Constraints on the Target Class.- Non U-Shaped Vacillatory and Team Learning.- Learning Multiple Languages in Groups.- Inferring Unions of the Pattern Languages by the Most Fitting Covers.- Identification in the Limit of Substitutable Context-Free Languages.- Algorithms for Learning Regular Expressions.- A Class of Prolog Programs with Non-linear Outputs Inferable from Positive Data.- Absolute Versus Probabilistic Classification in a Logical Setting.-Online Allocation with Risk Information.- Defensive Universal Learning with Experts.- On Following the Perturbed Leader in the Bandit Setting.- Mixture of Vector Experts.- On-line Learning with Delayed Label Feedback.- Monotone Conditional Complexity Bounds on Future Prediction Errors.- Non-asymptotic Calibration and Resolution.- Defensive Prediction with Expert Advice.- Defensive Forecasting for Linear Protocols.- Teaching Learners with Restricted Mind Changes.
Editors' Introduction.- Editors' Introduction.- Invited Papers.- Invention and Artificial Intelligence.- The Arrowsmith Project: 2005 Status Report.- The Robot Scientist Project.- Algorithms and Software for Collaborative Discovery from Autonomous, Semantically Heterogeneous, Distributed Information Sources.- Training Support Vector Machines via SMO-Type Decomposition Methods.- Kernel-Based Learning.- Measuring Statistical Dependence with Hilbert-Schmidt Norms.- An Analysis of the Anti-learning Phenomenon for the Class Symmetric Polyhedron.- Learning Causal Structures Based on Markov Equivalence Class.- Stochastic Complexity for Mixture of Exponential Families in Variational Bayes.- ACME: An Associative Classifier Based on Maximum Entropy Principle.- Constructing Multiclass Learners from Binary Learners: A Simple Black-Box Analysis of the Generalization Errors.- On Computability of Pattern Recognition Problems.- PAC-Learnability of Probabilistic Deterministic Finite State Automata in Terms of Variation Distance.- Learnability of Probabilistic Automata via Oracles.- Learning Attribute-Efficiently with Corrupt Oracles.- Learning DNF by Statistical and Proper Distance Queries Under the Uniform Distribution.- Learning of Elementary Formal Systems with Two Clauses Using Queries.- Gold-Style and Query Learning Under Various Constraints on the Target Class.- Non U-Shaped Vacillatory and Team Learning.- Learning Multiple Languages in Groups.- Inferring Unions of the Pattern Languages by the Most Fitting Covers.- Identification in the Limit of Substitutable Context-Free Languages.- Algorithms for Learning Regular Expressions.- A Class of Prolog Programs with Non-linear Outputs Inferable from Positive Data.- Absolute Versus Probabilistic Classification in a Logical Setting.-Online Allocation with Risk Information.- Defensive Universal Learning with Experts.- On Following the Perturbed Leader in the Bandit Setting.- Mixture of Vector Experts.- On-line Learning with Delayed Label Feedback.- Monotone Conditional Complexity Bounds on Future Prediction Errors.- Non-asymptotic Calibration and Resolution.- Defensive Prediction with Expert Advice.- Defensive Forecasting for Linear Protocols.- Teaching Learners with Restricted Mind Changes.