47,95 €
47,95 €
inkl. MwSt.
Sofort per Download lieferbar
24 °P sammeln
47,95 €
Als Download kaufen
47,95 €
inkl. MwSt.
Sofort per Download lieferbar
24 °P sammeln
Jetzt verschenken
Alle Infos zum eBook verschenken
47,95 €
inkl. MwSt.
Sofort per Download lieferbar
Alle Infos zum eBook verschenken
24 °P sammeln
- Format: PDF
- Merkliste
- Auf die Merkliste
- Bewerten Bewerten
- Teilen
- Produkt teilen
- Produkterinnerung
- Produkterinnerung
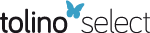
Bitte loggen Sie sich zunächst in Ihr Kundenkonto ein oder registrieren Sie sich bei
bücher.de, um das eBook-Abo tolino select nutzen zu können.
Hier können Sie sich einloggen
Hier können Sie sich einloggen
Sie sind bereits eingeloggt. Klicken Sie auf 2. tolino select Abo, um fortzufahren.
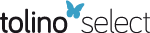
Bitte loggen Sie sich zunächst in Ihr Kundenkonto ein oder registrieren Sie sich bei bücher.de, um das eBook-Abo tolino select nutzen zu können.
What exactly is ML? How is it related to AI? Why is deep learning (DL) so popular these days? This book explains how traditional rule-based AI and ML work.
- Geräte: PC
- ohne Kopierschutz
- eBook Hilfe
- Größe: 9.06MB
Andere Kunden interessierten sich auch für
- Mark LiuAlphaGo Simplified (eBook, ePUB)47,95 €
- Brian McFeeDigital Signals Theory (eBook, PDF)45,95 €
- John Pile Jr.2D Graphics Programming for Games (eBook, PDF)63,95 €
- John M. QuickLearn to Code with Games (eBook, PDF)47,95 €
- Aaron LangilleA Gamer's Introduction to Programming in C (eBook, PDF)47,95 €
- Fletcher Dunn3D Math Primer for Graphics and Game Development (eBook, PDF)52,95 €
- Brian BeukenThe Fundamentals of C/C++ Game Programming (eBook, PDF)59,95 €
-
-
-
What exactly is ML? How is it related to AI? Why is deep learning (DL) so popular these days? This book explains how traditional rule-based AI and ML work.
Dieser Download kann aus rechtlichen Gründen nur mit Rechnungsadresse in A, B, BG, CY, CZ, D, DK, EW, E, FIN, F, GR, HR, H, IRL, I, LT, L, LR, M, NL, PL, P, R, S, SLO, SK ausgeliefert werden.
Produktdetails
- Produktdetails
- Verlag: Taylor & Francis
- Seitenzahl: 408
- Erscheinungstermin: 27. August 2024
- Englisch
- ISBN-13: 9781040103944
- Artikelnr.: 72285273
- Verlag: Taylor & Francis
- Seitenzahl: 408
- Erscheinungstermin: 27. August 2024
- Englisch
- ISBN-13: 9781040103944
- Artikelnr.: 72285273
- Herstellerkennzeichnung Die Herstellerinformationen sind derzeit nicht verfügbar.
Mark H. Liu is an Associate Professor of Finance, the (Founding) Director of the MS Finance Program at the University of Kentucky. He obtained his Ph.D. in finance from Boston College in 2004 and his M.A. in economics from Western University in Canada in 1998. Dr. Liu has more than 20 years of coding experience and is the author of two books: Make Python Talk (No Starch Press, 2021) and Machine Learning, Animated (CRC Press, 2023).
List of Figures
Preface
Acknowledgments
Section I Rule-Based A.I.
Chapter 1 Rule-Based AI in the Coin Game
Chapter 2 Look-Ahead Search in Tic Tac Toe
Chapter 3 Planning Three Steps Ahead in Connect Four
Chapter 4 Recursion and MiniMax Tree Search
Chapter 5 Depth Pruning in MiniMax
Chapter 6 Alpha-Beta Pruning
Chapter 7 Position Evaluation in MiniMax
Chapter 8 Monte Carlo Tree Search
Section II Deep Learning
Chapter 9 Deep Learning in the Coin Game
Chapter 10 Policy Networks in Tic Tac Toe
Chapter 11 A Policy Network in Connect Four
Section III Reinforcement Learning
Chapter 12 Tabular Q-Learning in the Coin Game
Chapter 13 Self-Play Deep Reinforcement Learning
Chapter 14 Vectorization to Speed Up Deep Reinforcement Learning
Chapter 15 A Value Network in Connect Four
Section IV AlphaGo Algorithms
Chapter 16 Implement AlphaGo in the Coin Game
Chapter 17 AlphaGo in Tic Tac Toe and Connect Four
Chapter 18 Hyperparameter Tuning in AlphaGo
Chapter 19 The Actor-Critic Method and AlphaZero
Chapter 20 Iterative Self-Play and AlphaZero in Tic Tac Toe
Chapter 21 AlphaZero in Unsolved Games
Bibliography
Preface
Acknowledgments
Section I Rule-Based A.I.
Chapter 1 Rule-Based AI in the Coin Game
Chapter 2 Look-Ahead Search in Tic Tac Toe
Chapter 3 Planning Three Steps Ahead in Connect Four
Chapter 4 Recursion and MiniMax Tree Search
Chapter 5 Depth Pruning in MiniMax
Chapter 6 Alpha-Beta Pruning
Chapter 7 Position Evaluation in MiniMax
Chapter 8 Monte Carlo Tree Search
Section II Deep Learning
Chapter 9 Deep Learning in the Coin Game
Chapter 10 Policy Networks in Tic Tac Toe
Chapter 11 A Policy Network in Connect Four
Section III Reinforcement Learning
Chapter 12 Tabular Q-Learning in the Coin Game
Chapter 13 Self-Play Deep Reinforcement Learning
Chapter 14 Vectorization to Speed Up Deep Reinforcement Learning
Chapter 15 A Value Network in Connect Four
Section IV AlphaGo Algorithms
Chapter 16 Implement AlphaGo in the Coin Game
Chapter 17 AlphaGo in Tic Tac Toe and Connect Four
Chapter 18 Hyperparameter Tuning in AlphaGo
Chapter 19 The Actor-Critic Method and AlphaZero
Chapter 20 Iterative Self-Play and AlphaZero in Tic Tac Toe
Chapter 21 AlphaZero in Unsolved Games
Bibliography
List of Figures
Preface
Acknowledgments
Section I Rule-Based A.I.
Chapter 1 Rule-Based AI in the Coin Game
Chapter 2 Look-Ahead Search in Tic Tac Toe
Chapter 3 Planning Three Steps Ahead in Connect Four
Chapter 4 Recursion and MiniMax Tree Search
Chapter 5 Depth Pruning in MiniMax
Chapter 6 Alpha-Beta Pruning
Chapter 7 Position Evaluation in MiniMax
Chapter 8 Monte Carlo Tree Search
Section II Deep Learning
Chapter 9 Deep Learning in the Coin Game
Chapter 10 Policy Networks in Tic Tac Toe
Chapter 11 A Policy Network in Connect Four
Section III Reinforcement Learning
Chapter 12 Tabular Q-Learning in the Coin Game
Chapter 13 Self-Play Deep Reinforcement Learning
Chapter 14 Vectorization to Speed Up Deep Reinforcement Learning
Chapter 15 A Value Network in Connect Four
Section IV AlphaGo Algorithms
Chapter 16 Implement AlphaGo in the Coin Game
Chapter 17 AlphaGo in Tic Tac Toe and Connect Four
Chapter 18 Hyperparameter Tuning in AlphaGo
Chapter 19 The Actor-Critic Method and AlphaZero
Chapter 20 Iterative Self-Play and AlphaZero in Tic Tac Toe
Chapter 21 AlphaZero in Unsolved Games
Bibliography
Preface
Acknowledgments
Section I Rule-Based A.I.
Chapter 1 Rule-Based AI in the Coin Game
Chapter 2 Look-Ahead Search in Tic Tac Toe
Chapter 3 Planning Three Steps Ahead in Connect Four
Chapter 4 Recursion and MiniMax Tree Search
Chapter 5 Depth Pruning in MiniMax
Chapter 6 Alpha-Beta Pruning
Chapter 7 Position Evaluation in MiniMax
Chapter 8 Monte Carlo Tree Search
Section II Deep Learning
Chapter 9 Deep Learning in the Coin Game
Chapter 10 Policy Networks in Tic Tac Toe
Chapter 11 A Policy Network in Connect Four
Section III Reinforcement Learning
Chapter 12 Tabular Q-Learning in the Coin Game
Chapter 13 Self-Play Deep Reinforcement Learning
Chapter 14 Vectorization to Speed Up Deep Reinforcement Learning
Chapter 15 A Value Network in Connect Four
Section IV AlphaGo Algorithms
Chapter 16 Implement AlphaGo in the Coin Game
Chapter 17 AlphaGo in Tic Tac Toe and Connect Four
Chapter 18 Hyperparameter Tuning in AlphaGo
Chapter 19 The Actor-Critic Method and AlphaZero
Chapter 20 Iterative Self-Play and AlphaZero in Tic Tac Toe
Chapter 21 AlphaZero in Unsolved Games
Bibliography