47,95 €
47,95 €
inkl. MwSt.
Sofort per Download lieferbar
24 °P sammeln
47,95 €
Als Download kaufen
47,95 €
inkl. MwSt.
Sofort per Download lieferbar
24 °P sammeln
Jetzt verschenken
Alle Infos zum eBook verschenken
47,95 €
inkl. MwSt.
Sofort per Download lieferbar
Alle Infos zum eBook verschenken
24 °P sammeln
- Format: ePub
- Merkliste
- Auf die Merkliste
- Bewerten Bewerten
- Teilen
- Produkt teilen
- Produkterinnerung
- Produkterinnerung
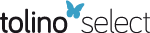
Bitte loggen Sie sich zunächst in Ihr Kundenkonto ein oder registrieren Sie sich bei
bücher.de, um das eBook-Abo tolino select nutzen zu können.
Hier können Sie sich einloggen
Hier können Sie sich einloggen
Sie sind bereits eingeloggt. Klicken Sie auf 2. tolino select Abo, um fortzufahren.
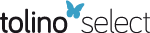
Bitte loggen Sie sich zunächst in Ihr Kundenkonto ein oder registrieren Sie sich bei bücher.de, um das eBook-Abo tolino select nutzen zu können.
Currently, there are a lack of intermediate /advanced level textbooks which introduce students and practicing statisticians to the updated methods on correlated data inference. T
- Geräte: eReader
- ohne Kopierschutz
- eBook Hilfe
- Größe: 2.28MB
Andere Kunden interessierten sich auch für
- You-Gan WangAnalysis of Longitudinal Data with Examples (eBook, PDF)47,95 €
- Peter H. WestfallUnderstanding Regression Analysis (eBook, ePUB)42,95 €
- Handbook of Statistical Methods for Randomized Controlled Trials (eBook, ePUB)65,95 €
- Modeling and Analysis of Longitudinal Data (eBook, ePUB)198,95 €
- Kevin J. KeenGraphics for Statistics and Data Analysis with R (eBook, ePUB)48,95 €
- Richard . H. JonesLongitudinal Data with Serial Correlation (eBook, ePUB)65,95 €
- Christopher D. DesjardinsHandbook of Educational Measurement and Psychometrics Using R (eBook, ePUB)48,95 €
-
-
-
Currently, there are a lack of intermediate /advanced level textbooks which introduce students and practicing statisticians to the updated methods on correlated data inference. T
Dieser Download kann aus rechtlichen Gründen nur mit Rechnungsadresse in A, B, BG, CY, CZ, D, DK, EW, E, FIN, F, GR, HR, H, IRL, I, LT, L, LR, M, NL, PL, P, R, S, SLO, SK ausgeliefert werden.
Produktdetails
- Produktdetails
- Verlag: Taylor & Francis
- Seitenzahl: 252
- Erscheinungstermin: 16. Februar 2022
- Englisch
- ISBN-13: 9781351649674
- Artikelnr.: 63104422
- Verlag: Taylor & Francis
- Seitenzahl: 252
- Erscheinungstermin: 16. Februar 2022
- Englisch
- ISBN-13: 9781351649674
- Artikelnr.: 63104422
- Herstellerkennzeichnung Die Herstellerinformationen sind derzeit nicht verfügbar.
Professor Wang obtained his Ph.D. on dynamic optimization in 1991 (University of Oxford) and worked for CSIRO (2005-2010). Before returning to Australia, Professor Wang worked for the National University of Singapore (2001-2005) and Harvard University as Assistant Professor and Associate Professor (1998-2000) in biostatistics. He joined the University of Queensland in April 2010 as Chair Professor of Applied Statistics to lead the Centre for Applications in Natural Resource Mathematics and to promote applied statistics and mathematics. Currently, he is Capacity Building Professor in Data Science at Queensland University of Technology, Australia. Professor Wang has developed a number of novel statistical methodologies in longitudinal data analysis published by top statistical journals (Biometrika, Biometrics, Statistics in Medicine, Journal of the American Statistician Association, Annals of Statistics). His recent interests and successes include (1) 'working' likelihood approach for hyperparameter estimation and model selection, (2) integrating statistical learning and machine learning for dependent data analysis and (3) data-driven approach for robust estimation. More recently, he advocates 'working' likelihood approaches to parameter estimation but recognizing possibly a different likelihood that generating the observed data in inferencing. This has been found very useful in finding datadependent tuning parameters in robust estimation and hyper-parameters in machine learning algorithms.
Liya Fu obtained her Ph.D. in 2010 from Northeast Normal University. Currently she is Associate Professor of Statistics at Xi'an Jiaotong University. She worked briefly as a Postdoctoral Fellow at the University of Queensland after after two-years visiting student at CSIRO (2008-010), Australia. Dr. Fu mainly focuses on the methodologies for the analysis of longitudinal data and has published about 20 papers in international journals, including Biometrics, Statistics in Medicine, Journal of Multivariate Analysis. Professor Sudhir Paul obtained his PhD in 1976 (University of Wales). He worked as a Postdoctoral Fellow (University of Newcastle Upon Tyne, 1976- 1978) and a Lecturer (University of Kent at Canterbury, 1978-1982) before moving to Canada in 1982. He started as Assistant Professor at the University of Windsor and moved through all professorial ranks and finally in 2005 became distinguished University Professor. He became Fellow of the Royal Statistical Society in 1982 and Fellow of the American Statistical Association in 1986.
Professor Paul has developed many methodologies for the analyses of overdispersed and zero-inflated count data, longitudinal data, and familial data and published in most of the top-tier journals in statistics (Journal of the Royal Statistical Society, Biometrika, Biometrics, Journal of the American Statistician Association, Technometrics). Professor Paul supervised over 50 graduate students including 16 PhD students and has published over 100 papers.
Liya Fu obtained her Ph.D. in 2010 from Northeast Normal University. Currently she is Associate Professor of Statistics at Xi'an Jiaotong University. She worked briefly as a Postdoctoral Fellow at the University of Queensland after after two-years visiting student at CSIRO (2008-010), Australia. Dr. Fu mainly focuses on the methodologies for the analysis of longitudinal data and has published about 20 papers in international journals, including Biometrics, Statistics in Medicine, Journal of Multivariate Analysis. Professor Sudhir Paul obtained his PhD in 1976 (University of Wales). He worked as a Postdoctoral Fellow (University of Newcastle Upon Tyne, 1976- 1978) and a Lecturer (University of Kent at Canterbury, 1978-1982) before moving to Canada in 1982. He started as Assistant Professor at the University of Windsor and moved through all professorial ranks and finally in 2005 became distinguished University Professor. He became Fellow of the Royal Statistical Society in 1982 and Fellow of the American Statistical Association in 1986.
Professor Paul has developed many methodologies for the analyses of overdispersed and zero-inflated count data, longitudinal data, and familial data and published in most of the top-tier journals in statistics (Journal of the Royal Statistical Society, Biometrika, Biometrics, Journal of the American Statistician Association, Technometrics). Professor Paul supervised over 50 graduate students including 16 PhD students and has published over 100 papers.
Chapter 1 Introduction Chapter 2 Examples and Organization of The Book Chapter 3 Model Framework and Its Components Chapter 4 Parameter Estimation Chapter 5 Model Selection Chapter 6 Robust Approaches Chapter 7 Clustered Data Analysis Chapter 8 Missing Data Analysis Chapter 9 Random Effects and Transitional Models Chapter 10 Handing High Dimensional Longitudinal Data
Chapter 1 Introduction Chapter 2 Examples and Organization of The Book
Chapter 3 Model Framework and Its Components Chapter 4 Parameter Estimation
Chapter 5 Model Selection Chapter 6 Robust Approaches Chapter 7 Clustered
Data Analysis Chapter 8 Missing Data Analysis Chapter 9 Random Effects and
Transitional Models Chapter 10 Handing High Dimensional Longitudinal Data
Chapter 3 Model Framework and Its Components Chapter 4 Parameter Estimation
Chapter 5 Model Selection Chapter 6 Robust Approaches Chapter 7 Clustered
Data Analysis Chapter 8 Missing Data Analysis Chapter 9 Random Effects and
Transitional Models Chapter 10 Handing High Dimensional Longitudinal Data
Chapter 1 Introduction Chapter 2 Examples and Organization of The Book Chapter 3 Model Framework and Its Components Chapter 4 Parameter Estimation Chapter 5 Model Selection Chapter 6 Robust Approaches Chapter 7 Clustered Data Analysis Chapter 8 Missing Data Analysis Chapter 9 Random Effects and Transitional Models Chapter 10 Handing High Dimensional Longitudinal Data
Chapter 1 Introduction Chapter 2 Examples and Organization of The Book
Chapter 3 Model Framework and Its Components Chapter 4 Parameter Estimation
Chapter 5 Model Selection Chapter 6 Robust Approaches Chapter 7 Clustered
Data Analysis Chapter 8 Missing Data Analysis Chapter 9 Random Effects and
Transitional Models Chapter 10 Handing High Dimensional Longitudinal Data
Chapter 3 Model Framework and Its Components Chapter 4 Parameter Estimation
Chapter 5 Model Selection Chapter 6 Robust Approaches Chapter 7 Clustered
Data Analysis Chapter 8 Missing Data Analysis Chapter 9 Random Effects and
Transitional Models Chapter 10 Handing High Dimensional Longitudinal Data