Analysis of Poverty Data by Small Area Estimation (eBook, ePUB)
Redaktion: Pratesi, Monica
Alle Infos zum eBook verschenken
Analysis of Poverty Data by Small Area Estimation (eBook, ePUB)
Redaktion: Pratesi, Monica
- Format: ePub
- Merkliste
- Auf die Merkliste
- Bewerten Bewerten
- Teilen
- Produkt teilen
- Produkterinnerung
- Produkterinnerung
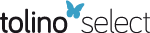
Hier können Sie sich einloggen
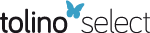
Bitte loggen Sie sich zunächst in Ihr Kundenkonto ein oder registrieren Sie sich bei bücher.de, um das eBook-Abo tolino select nutzen zu können.
A comprehensive guide to implementing SAE methods for poverty studies and poverty mapping There is an increasingly urgent demand for poverty and living conditions data, in relation to local areas and/or subpopulations. Policy makers and stakeholders need indicators and maps of poverty and living conditions in order to formulate and implement policies, (re)distribute resources, and measure the effect of local policy actions. Small Area Estimation (SAE) plays a crucial role in producing statistically sound estimates for poverty mapping. This book offers a comprehensive source of information…mehr
- Geräte: eReader
- mit Kopierschutz
- eBook Hilfe
- Größe: 28.85MB
- J. N. K. RaoSmall Area Estimation (eBook, ePUB)98,99 €
- Analysis of Poverty Data by Small Area Estimation (eBook, PDF)75,99 €
- Azizur RahmanSmall Area Estimation and Microsimulation Modeling (eBook, ePUB)50,95 €
- John Olusegun OgundareUnderstanding Least Squares Estimation and Geomatics Data Analysis (eBook, ePUB)107,99 €
- Peter J. HuberData Analysis (eBook, ePUB)116,99 €
- Kristin H. JarmanThe Art of Data Analysis (eBook, ePUB)56,99 €
- AgungPanel Data Analysis using EViews (eBook, ePUB)105,99 €
-
-
-
Dieser Download kann aus rechtlichen Gründen nur mit Rechnungsadresse in A, B, BG, CY, CZ, D, DK, EW, E, FIN, F, GR, HR, H, IRL, I, LT, L, LR, M, NL, PL, P, R, S, SLO, SK ausgeliefert werden.
- Produktdetails
- Verlag: John Wiley & Sons
- Seitenzahl: 472
- Erscheinungstermin: 29. Dezember 2015
- Englisch
- ISBN-13: 9781118815007
- Artikelnr.: 44449413
- Verlag: John Wiley & Sons
- Seitenzahl: 472
- Erscheinungstermin: 29. Dezember 2015
- Englisch
- ISBN-13: 9781118815007
- Artikelnr.: 44449413
- Herstellerkennzeichnung Die Herstellerinformationen sind derzeit nicht verfügbar.
) 221 11.A.2 Calculation of g2(
) 221 11.A.3 Calculation of g3(
) 222 Acknowledgements 224 References 224 12 Unit Level Spatio-temporal Models 227 Maria Chiara Pagliarella and Renato Salvatore 12.1 Unit Level Models 230 12.2 Spatio-temporal Time-varying Effects Models 232 12.3 State Space Models with Spatial Structure 234 12.4 The Italian EU-SILC Data: an Application with the Spatio-temporal Unit Level Models 236 12.5 Concluding Remarks 239 Appendix 12.A: Restricted Maximum Likelihood Estimation 240 Appendix 12.B: Mean Squared Error Estimation of the Unit Level State Space Model 241 References 242 13 Spatial Information and Geoadditive Small Area Models 245 Chiara Bocci and Alessandra Petrucci 13.1 Introduction 245 13.2 Geoadditive Models 247 13.3 Geoadditive Small Area Model for Skewed Data 248 13.4 Small Area Mean Estimators 250 13.5 Estimation of the Household Per-capita Consumption Expenditure in Albania 251 13.5.1 Data 251 13.5.2 Results 253 13.6 Concluding Remarks and Open Questions 258 Acknowledgement 259 References 259 Part V SMALL AREA ESTIMATION OF THE DISTRIBUTION FUNCTION OF INCOME AND INEQUALITIES 14 Model-based Direct Estimation of a Small Area Distribution Function 263 Hukum Chandra, Nicola Salvati and Ray Chambers 14.1 Introduction 263 14.2 Estimation of the Small Area Distribution Function 264 14.3 Model-based Direct Estimator for the Estimation of the Distribution Function of Equivalized Income in the Toscana, Lombardia and Campania Provinces of Italy 268 14.4 Final Remarks 275 References 276 15 Small Area Estimation for Lognormal Data 279 Emily Berg, Hukum Chandra and Ray Chambers 15.1 Introduction 279 15.2 Literature on Small Area Estimation for Skewed Data 280 15.3 Small Area Predictors for a Unit-Level Lognormal Model 282 15.3.1 The Linear Unit-Level Mixed Model 282 15.3.2 A Synthetic Estimator 283 15.3.3 A Model-Based Direct Estimator 285 15.3.4 An Empirical Bayes Predictor 286 15.4 Simulations 287 15.4.1 Comparison of Synthetic, TrMBDE, and EB Predictors 287 15.4.2 Bias and Robustness of the EB Predictor 291 15.4.3 Comparison of Lognormal and Gamma Distributions 291 15.5 Concluding Remarks 294 Appendix 15.A: Mean Squared Error Estimation for the Empirical Best Predictor 295 References 296 16 Bayesian Beta Regression Models for the Estimation of Poverty and Inequality Parameters in Small Areas 299 Enrico Fabrizi, Maria Rosaria Ferrante and Carlo Trivisano 16.1 Introduction 299 16.2 Direct Estimation 300 16.3 Small Area Estimation of the At-risk-of-poverty Rate 302 16.3.1 The Model 302 16.3.2 Data Analysis 304 16.4 Small Area Estimation of the Material Deprivation Rates 305 16.4.1 The Model 305 16.4.2 Data Analysis 306 16.5 Joint Estimation of the At-risk-of-poverty Rate and the Gini Coefficient 308 16.5.1 The Models 308 16.5.2 Data Analysis 310 16.6 A Short Description of Markov Chain Monte Carlo Algorithms and R Software Codes 312 16.7 Concluding Remarks 312 References 313 17 Empirical Bayes and Hierarchical Bayes Estimation of Poverty Measures for Small Areas 315 John N. K. Rao and Isabel Molina 17.1 Introduction 315 17.2 Poverty Measures 316 17.3 Fay-Herriot Area Level Model 317 17.4 Unit Level Nested Error Linear Regression Model 319 17.4.1 ELL/World Bank Method 319 17.4.2 Empirical Bayes Method 321 17.4.3 Hierarchical Bayes Method 322 17.5 Application 323 17.6 Concluding Remarks 324 References 324 Part VI DATA ANALYSIS AND APPLICATIONS 18 Small Area Estimation Using Both Survey and Census Unit Record Data 327 Stephen J. Haslett 18.1 Introduction 327 18.2 The ELL Implementation Process and Methodology 329 18.2.1 ELL: Implementation Process 329 18.2.2 ELL Methodology: Survey Regression, Contextual Effects, Clustering, and the Bootstrap 331 18.2.3 Fitting Survey-based Models 334 18.2.4 Residuals and the Bootstrap 335 18.2.5 ELL: Linkages to Other Related Methods 338 18.3 ELL - Advantages, Criticisms and Disadvantages 339 18.4 Conclusions 344 References 346 19 An Overview of the U.S. Census Bureau's Small Area Income and Poverty Estimates Program 349 William R. Bell, Wesley W. Basel and Jerry J. Maples 19.1 Introduction 349 19.2 U.S. Poverty Measure and Poverty Data Sources 351 19.2.1 Poverty Measure and Survey Data Sources 351 19.2.2 Administrative Data Sources Used for Covariate Information 354 19.3 SAIPE Poverty Models and Estimation Procedures 356 19.3.1 State Poverty Models 357 19.3.2 County Poverty Models 363 19.3.3 School District Poverty Estimation 368 19.3.4 Major Changes Made in SAIPE Models and Estimation Procedures 372 19.4 Current Challenges and Recent SAIPE Research 374 19.5 Conclusions 375 References 376 20 Poverty Mapping for the Chilean Comunas 379 Carolina Casas-Cordero Valencia, Jenny Encina and Partha Lahiri 20.1 Introduction 379 20.2 Chilean Poverty Measures and Casen 381 20.2.1 The Poverty Measure Used in Chile 381 20.2.2 The Casen Survey 382 20.3 Data Preparation 383 20.3.1 Comuna Level Data Derived from Casen 2009 383 20.3.2 Comuna Level Administrative Data 387 20.4 Description of the Small Area Estimation Method Implemented in Chile 391 20.4.1 Modeling 394 20.4.2 Estimation of A and
395 20.4.3 Empirical Bayes Estimator of
i 395 20.4.4 Limited Translation Empirical Bayes Estimator of
i 395 20.4.5 Back-transformation and raking 396 20.4.6 Confidence intervals for the poverty rates 396 20.5 Data Analysis 397 20.6 Discussion 399 Acknowledgements 401 References 402 21 Appendix on Software and Codes Used in the Book 405 Antonella D'Agostino, Francesca Gagliardi and Laura Neri 21.1 Introduction 405 21.2 R and SAS Software: a Brief Note 406 21.3 Getting Started: EU-SILC Data 409 21.4 A Quick Guide to the Scripts 410 21.4.1 Basics of the Scripts 410 21.4.2 A Quick guide to Chapter 5 (Impact of Sampling Designs in Small Area Estimation with Applications to Poverty Measurement) 412 21.4.3 A Quick guide to Chapter 6 (Model-assisted Methods for Small Area Estimation of Poverty Indicators) 412 21.4.4 A Quick Guide to Chapter 7 (Variance Estimation for Cumulative and Longitudinal Poverty Indicators from Panel Data at Regional Level) 414 21.4.5 A Quick Guide to Chapter 8 (Models in Small Area Estimation when Covariates are Measured with Error) 415 21.4.6 A Quick Guide to Chapter 9 (Robust Domain Estimation of Income-based Inequality Indicators) 416 21.4.7 A Quick Guide to Chapter 10 (Nonparametric Regression Methods for Small Area Estimation) 417 21.4.8 A Quick Guide to Chapter 11 (Area-level Spatio-temporal Small Area Estimation Models) 418 21.4.9 A Quick Guide to Chapter 12 (Unit Level Spatio-temporal Models) 419 21.4.10 A Quick Guide to Chapter 13 (Spatial Information and Geoadditive Small Area Models) 420 21.4.11 A Quick guide to Chapter 14 (Model-based Direct Estimation of a Small Area Distribution Function) 422 21.4.12 A Quick Guide to Chapter 16 (Bayesian Beta Regression Models for the Estimation of Poverty and Inequality Parameters in Small Areas) 423 21.4.13 A Quick Guide to Chapter 17 (Empirical Bayes and Hierarchical Bayes Estimation of Poverty Measures for Small Areas) 424 21.4.14 A Quick Guide to Chapter 18 - (Small Area Estimation Using Both Survey and Census Unit Record Data: Links, Alternatives, and the Central Roles of Regression and Contextual Variables) 425 References 426 Author Index 427 Subject Index 431
) 221 11.A.2 Calculation of g2(
) 221 11.A.3 Calculation of g3(
) 222 Acknowledgements 224 References 224 12 Unit Level Spatio-temporal Models 227 Maria Chiara Pagliarella and Renato Salvatore 12.1 Unit Level Models 230 12.2 Spatio-temporal Time-varying Effects Models 232 12.3 State Space Models with Spatial Structure 234 12.4 The Italian EU-SILC Data: an Application with the Spatio-temporal Unit Level Models 236 12.5 Concluding Remarks 239 Appendix 12.A: Restricted Maximum Likelihood Estimation 240 Appendix 12.B: Mean Squared Error Estimation of the Unit Level State Space Model 241 References 242 13 Spatial Information and Geoadditive Small Area Models 245 Chiara Bocci and Alessandra Petrucci 13.1 Introduction 245 13.2 Geoadditive Models 247 13.3 Geoadditive Small Area Model for Skewed Data 248 13.4 Small Area Mean Estimators 250 13.5 Estimation of the Household Per-capita Consumption Expenditure in Albania 251 13.5.1 Data 251 13.5.2 Results 253 13.6 Concluding Remarks and Open Questions 258 Acknowledgement 259 References 259 Part V SMALL AREA ESTIMATION OF THE DISTRIBUTION FUNCTION OF INCOME AND INEQUALITIES 14 Model-based Direct Estimation of a Small Area Distribution Function 263 Hukum Chandra, Nicola Salvati and Ray Chambers 14.1 Introduction 263 14.2 Estimation of the Small Area Distribution Function 264 14.3 Model-based Direct Estimator for the Estimation of the Distribution Function of Equivalized Income in the Toscana, Lombardia and Campania Provinces of Italy 268 14.4 Final Remarks 275 References 276 15 Small Area Estimation for Lognormal Data 279 Emily Berg, Hukum Chandra and Ray Chambers 15.1 Introduction 279 15.2 Literature on Small Area Estimation for Skewed Data 280 15.3 Small Area Predictors for a Unit-Level Lognormal Model 282 15.3.1 The Linear Unit-Level Mixed Model 282 15.3.2 A Synthetic Estimator 283 15.3.3 A Model-Based Direct Estimator 285 15.3.4 An Empirical Bayes Predictor 286 15.4 Simulations 287 15.4.1 Comparison of Synthetic, TrMBDE, and EB Predictors 287 15.4.2 Bias and Robustness of the EB Predictor 291 15.4.3 Comparison of Lognormal and Gamma Distributions 291 15.5 Concluding Remarks 294 Appendix 15.A: Mean Squared Error Estimation for the Empirical Best Predictor 295 References 296 16 Bayesian Beta Regression Models for the Estimation of Poverty and Inequality Parameters in Small Areas 299 Enrico Fabrizi, Maria Rosaria Ferrante and Carlo Trivisano 16.1 Introduction 299 16.2 Direct Estimation 300 16.3 Small Area Estimation of the At-risk-of-poverty Rate 302 16.3.1 The Model 302 16.3.2 Data Analysis 304 16.4 Small Area Estimation of the Material Deprivation Rates 305 16.4.1 The Model 305 16.4.2 Data Analysis 306 16.5 Joint Estimation of the At-risk-of-poverty Rate and the Gini Coefficient 308 16.5.1 The Models 308 16.5.2 Data Analysis 310 16.6 A Short Description of Markov Chain Monte Carlo Algorithms and R Software Codes 312 16.7 Concluding Remarks 312 References 313 17 Empirical Bayes and Hierarchical Bayes Estimation of Poverty Measures for Small Areas 315 John N. K. Rao and Isabel Molina 17.1 Introduction 315 17.2 Poverty Measures 316 17.3 Fay-Herriot Area Level Model 317 17.4 Unit Level Nested Error Linear Regression Model 319 17.4.1 ELL/World Bank Method 319 17.4.2 Empirical Bayes Method 321 17.4.3 Hierarchical Bayes Method 322 17.5 Application 323 17.6 Concluding Remarks 324 References 324 Part VI DATA ANALYSIS AND APPLICATIONS 18 Small Area Estimation Using Both Survey and Census Unit Record Data 327 Stephen J. Haslett 18.1 Introduction 327 18.2 The ELL Implementation Process and Methodology 329 18.2.1 ELL: Implementation Process 329 18.2.2 ELL Methodology: Survey Regression, Contextual Effects, Clustering, and the Bootstrap 331 18.2.3 Fitting Survey-based Models 334 18.2.4 Residuals and the Bootstrap 335 18.2.5 ELL: Linkages to Other Related Methods 338 18.3 ELL - Advantages, Criticisms and Disadvantages 339 18.4 Conclusions 344 References 346 19 An Overview of the U.S. Census Bureau's Small Area Income and Poverty Estimates Program 349 William R. Bell, Wesley W. Basel and Jerry J. Maples 19.1 Introduction 349 19.2 U.S. Poverty Measure and Poverty Data Sources 351 19.2.1 Poverty Measure and Survey Data Sources 351 19.2.2 Administrative Data Sources Used for Covariate Information 354 19.3 SAIPE Poverty Models and Estimation Procedures 356 19.3.1 State Poverty Models 357 19.3.2 County Poverty Models 363 19.3.3 School District Poverty Estimation 368 19.3.4 Major Changes Made in SAIPE Models and Estimation Procedures 372 19.4 Current Challenges and Recent SAIPE Research 374 19.5 Conclusions 375 References 376 20 Poverty Mapping for the Chilean Comunas 379 Carolina Casas-Cordero Valencia, Jenny Encina and Partha Lahiri 20.1 Introduction 379 20.2 Chilean Poverty Measures and Casen 381 20.2.1 The Poverty Measure Used in Chile 381 20.2.2 The Casen Survey 382 20.3 Data Preparation 383 20.3.1 Comuna Level Data Derived from Casen 2009 383 20.3.2 Comuna Level Administrative Data 387 20.4 Description of the Small Area Estimation Method Implemented in Chile 391 20.4.1 Modeling 394 20.4.2 Estimation of A and
395 20.4.3 Empirical Bayes Estimator of
i 395 20.4.4 Limited Translation Empirical Bayes Estimator of
i 395 20.4.5 Back-transformation and raking 396 20.4.6 Confidence intervals for the poverty rates 396 20.5 Data Analysis 397 20.6 Discussion 399 Acknowledgements 401 References 402 21 Appendix on Software and Codes Used in the Book 405 Antonella D'Agostino, Francesca Gagliardi and Laura Neri 21.1 Introduction 405 21.2 R and SAS Software: a Brief Note 406 21.3 Getting Started: EU-SILC Data 409 21.4 A Quick Guide to the Scripts 410 21.4.1 Basics of the Scripts 410 21.4.2 A Quick guide to Chapter 5 (Impact of Sampling Designs in Small Area Estimation with Applications to Poverty Measurement) 412 21.4.3 A Quick guide to Chapter 6 (Model-assisted Methods for Small Area Estimation of Poverty Indicators) 412 21.4.4 A Quick Guide to Chapter 7 (Variance Estimation for Cumulative and Longitudinal Poverty Indicators from Panel Data at Regional Level) 414 21.4.5 A Quick Guide to Chapter 8 (Models in Small Area Estimation when Covariates are Measured with Error) 415 21.4.6 A Quick Guide to Chapter 9 (Robust Domain Estimation of Income-based Inequality Indicators) 416 21.4.7 A Quick Guide to Chapter 10 (Nonparametric Regression Methods for Small Area Estimation) 417 21.4.8 A Quick Guide to Chapter 11 (Area-level Spatio-temporal Small Area Estimation Models) 418 21.4.9 A Quick Guide to Chapter 12 (Unit Level Spatio-temporal Models) 419 21.4.10 A Quick Guide to Chapter 13 (Spatial Information and Geoadditive Small Area Models) 420 21.4.11 A Quick guide to Chapter 14 (Model-based Direct Estimation of a Small Area Distribution Function) 422 21.4.12 A Quick Guide to Chapter 16 (Bayesian Beta Regression Models for the Estimation of Poverty and Inequality Parameters in Small Areas) 423 21.4.13 A Quick Guide to Chapter 17 (Empirical Bayes and Hierarchical Bayes Estimation of Poverty Measures for Small Areas) 424 21.4.14 A Quick Guide to Chapter 18 - (Small Area Estimation Using Both Survey and Census Unit Record Data: Links, Alternatives, and the Central Roles of Regression and Contextual Variables) 425 References 426 Author Index 427 Subject Index 431