Applications of Computational Intelligence in Concrete Technology (eBook, PDF)
Redaktion: Gupta, Sakshi; Kose, Utku; Thakur, Mohindra Singh; Sihag, Parveen
48,95 €
48,95 €
inkl. MwSt.
Sofort per Download lieferbar
24 °P sammeln
48,95 €
Als Download kaufen
48,95 €
inkl. MwSt.
Sofort per Download lieferbar
24 °P sammeln
Jetzt verschenken
Alle Infos zum eBook verschenken
48,95 €
inkl. MwSt.
Sofort per Download lieferbar
Alle Infos zum eBook verschenken
24 °P sammeln
Applications of Computational Intelligence in Concrete Technology (eBook, PDF)
Redaktion: Gupta, Sakshi; Kose, Utku; Thakur, Mohindra Singh; Sihag, Parveen
- Format: PDF
- Merkliste
- Auf die Merkliste
- Bewerten Bewerten
- Teilen
- Produkt teilen
- Produkterinnerung
- Produkterinnerung
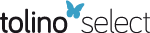
Bitte loggen Sie sich zunächst in Ihr Kundenkonto ein oder registrieren Sie sich bei
bücher.de, um das eBook-Abo tolino select nutzen zu können.
Hier können Sie sich einloggen
Hier können Sie sich einloggen
Sie sind bereits eingeloggt. Klicken Sie auf 2. tolino select Abo, um fortzufahren.
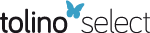
Bitte loggen Sie sich zunächst in Ihr Kundenkonto ein oder registrieren Sie sich bei bücher.de, um das eBook-Abo tolino select nutzen zu können.
This book discusses the selection and separation of data sets, performance evaluation parameters for different types of concrete and related materials, and sensitivity analysis related to various CI techniques. The technical contents included is beneficial for researchers as well as practicing engineers in concrete and construction industry.
- Geräte: PC
- mit Kopierschutz
- eBook Hilfe
Andere Kunden interessierten sich auch für
- Applications of Computational Intelligence in Concrete Technology (eBook, ePUB)48,95 €
- Karthikeyan H OblaImproving Concrete Quality (eBook, PDF)125,95 €
- Paul ChessDurability of Reinforced Concrete Structures (eBook, PDF)23,95 €
- Sprayed Concrete Technology (eBook, PDF)66,95 €
- Farzad HejaziSolving Complex Problems for Structures and Bridges using ABAQUS Finite Element Package (eBook, PDF)47,95 €
- George GarberDesign and Construction of Concrete Floors (eBook, PDF)49,95 €
- Lukasz SadowskiNon-Destructive Diagnostics of Concrete Floors (eBook, PDF)48,95 €
-
-
-
This book discusses the selection and separation of data sets, performance evaluation parameters for different types of concrete and related materials, and sensitivity analysis related to various CI techniques. The technical contents included is beneficial for researchers as well as practicing engineers in concrete and construction industry.
Dieser Download kann aus rechtlichen Gründen nur mit Rechnungsadresse in A, B, BG, CY, CZ, D, DK, EW, E, FIN, F, GR, HR, H, IRL, I, LT, L, LR, M, NL, PL, P, R, S, SLO, SK ausgeliefert werden.
Produktdetails
- Produktdetails
- Verlag: Taylor & Francis
- Seitenzahl: 320
- Erscheinungstermin: 23. Juni 2022
- Englisch
- ISBN-13: 9781000600544
- Artikelnr.: 63905967
- Verlag: Taylor & Francis
- Seitenzahl: 320
- Erscheinungstermin: 23. Juni 2022
- Englisch
- ISBN-13: 9781000600544
- Artikelnr.: 63905967
- Herstellerkennzeichnung Die Herstellerinformationen sind derzeit nicht verfügbar.
Miss Sakshi Gupta is Assistant Professor in the Department of Civil Engineering, Amity School of Engineering & Technology, Amity University Haryana. She is pursuing PhD from Indian Institute of Technology Delhi, India and has completed M.Tech in Structural Engineering from National Institute of Technology, Kurukshetra, India in the year 2013 and B.Tech from MD University, Rohtak, Haryana, India in 2011. Her areas of interest include building information modeling, concrete technology, sustainable construction, construction technology & management, computation intelligence techniques, and soft computing techniques in civil engineering applications. She is the reviewer and editorial board member of many scientific journals. She has published more than 30 papers in reputed international and national journals and attended various conferences. She had also authored many books and book chapters in Elsevier & Springer. Dr. Parveen Sihag is presently working as Assistant Professor in Chandigarh University, India. He has completed B. Tech in Civil Engineering, M. Tech in Water Resources Engineering and Ph.D. in civil Engineering Department, National Institute of Technology, Kurukshetra, India. The area of interests is Hydrology, Infiltration, soft computing and optimization. He has published more than 70 research papers in conferences and journals. Dr. M.S.Thakur has been working as Professor in Department of Civil Engineering, Shoolini University, Solan HP India since 2015. He obtained his B.E..(Civil) from Punjab Engineering College Chandigarh (1980), M.Tech. (Structures) from IIT Bombay (1988) and PhD from Stevens Institute of Technology (2002), Hoboken, New Jersey USA. He has published papers in many international conferences and journals. He is not only an academician but is also an engineer having worked with last posting as Engineer-in Chief in HP Public Works Department in 2015. Dr. Utku Kose received the B.S. degree in 2008 from computer education of Gazi University, Turkey as a faculty valedictorian. He received M.S. degree in 2010 from Afyon Kocatepe University, Turkey in the field of computer and D.S. / Ph. D. degree in 2017 from Selcuk University, Turkey in the field of computer engineering. Currently, he is an Associate Professor in Suleyman Demirel University, Turkey. He has more than 100 publications including articles, authored and edited books, proceedings, and reports. He is also in editorial boards of many scientific journals and serves as one of the editors of the Biomedical and Robotics Healthcare book series by CRC Press. His research interest includes artificial intelligence, machine ethics, artificial intelligence safety, biomedical applications, optimization, the chaos theory, distance education, e-learning, computer education, and computer science.
1. Usage of Computational Intelligence Techniques in Concrete Technology,
2. Developing Random Forest Random Tree and Linear Regression Model to
Predict Compressive Strength of Concrete Using Glass Fiber, 3. Prediction
of Compressive Strength at Elevated Temperatures Using Machine Learning
Methods, 4. Implementation of Machine Learning Approaches to Evaluate
Flexural Strength of Concrete With Glass Fiber, 5. A Comparative Study
Using ANFIS and ANN for Determining the Compressive Strength of Concrete,
6. Prediction of Concrete Mix Compressive Strength Using Waste Marble
Powder: A Comparison of ANN, RF, RT, and LR models, 7. Using GA to Predict
the Compressive Strength of Concrete Containing Nano-Silica, 8. Evaluation
of Models by Soft Computing Techniques for the Prediction of Compressive
Strength of Concrete Using Steel Fibre, 9. Using Regression Model to
Estimate the Splitting Tensile Strength for the Concrete With Basalt Fiber
Reinforced Concrete, 10. Prediction of Compressive Strength of
Self-Compacting Concrete Containing Silica's Using Soft Computing
Techniques, 11. Using Soft Computing Techniques to Predict the Values of
Compressive Strength of Concrete with Basalt Fiber Reinforced Concrete, 12.
Soft Computing-Based Prediction of Compressive Strength of High Strength
Concrete, 13. Forecasting Compressive Strength of Concrete Containing
Nano-Silica Using Particle Swarm Optimization Algorithm and Genetic
Algorithm, 14. Prediction of Ultrasonic Pulse Velocity of Concrete, 15.
Evaluation of ANN and Tree-Based Techniques for Predicting the Compressive
Strength of Granite Powder Reinforced Concrete, 16. Predicting Recycled
Aggregates Compressive Strength in High-Performance Concrete Using
Artificial Neural Networks, 17. Compressive Strength Prediction and
Analysis of Concrete Using Hybrid Artificial Neural Networks
2. Developing Random Forest Random Tree and Linear Regression Model to
Predict Compressive Strength of Concrete Using Glass Fiber, 3. Prediction
of Compressive Strength at Elevated Temperatures Using Machine Learning
Methods, 4. Implementation of Machine Learning Approaches to Evaluate
Flexural Strength of Concrete With Glass Fiber, 5. A Comparative Study
Using ANFIS and ANN for Determining the Compressive Strength of Concrete,
6. Prediction of Concrete Mix Compressive Strength Using Waste Marble
Powder: A Comparison of ANN, RF, RT, and LR models, 7. Using GA to Predict
the Compressive Strength of Concrete Containing Nano-Silica, 8. Evaluation
of Models by Soft Computing Techniques for the Prediction of Compressive
Strength of Concrete Using Steel Fibre, 9. Using Regression Model to
Estimate the Splitting Tensile Strength for the Concrete With Basalt Fiber
Reinforced Concrete, 10. Prediction of Compressive Strength of
Self-Compacting Concrete Containing Silica's Using Soft Computing
Techniques, 11. Using Soft Computing Techniques to Predict the Values of
Compressive Strength of Concrete with Basalt Fiber Reinforced Concrete, 12.
Soft Computing-Based Prediction of Compressive Strength of High Strength
Concrete, 13. Forecasting Compressive Strength of Concrete Containing
Nano-Silica Using Particle Swarm Optimization Algorithm and Genetic
Algorithm, 14. Prediction of Ultrasonic Pulse Velocity of Concrete, 15.
Evaluation of ANN and Tree-Based Techniques for Predicting the Compressive
Strength of Granite Powder Reinforced Concrete, 16. Predicting Recycled
Aggregates Compressive Strength in High-Performance Concrete Using
Artificial Neural Networks, 17. Compressive Strength Prediction and
Analysis of Concrete Using Hybrid Artificial Neural Networks
1. Usage of Computational Intelligence Techniques in Concrete Technology,
2. Developing Random Forest Random Tree and Linear Regression Model to
Predict Compressive Strength of Concrete Using Glass Fiber, 3. Prediction
of Compressive Strength at Elevated Temperatures Using Machine Learning
Methods, 4. Implementation of Machine Learning Approaches to Evaluate
Flexural Strength of Concrete With Glass Fiber, 5. A Comparative Study
Using ANFIS and ANN for Determining the Compressive Strength of Concrete,
6. Prediction of Concrete Mix Compressive Strength Using Waste Marble
Powder: A Comparison of ANN, RF, RT, and LR models, 7. Using GA to Predict
the Compressive Strength of Concrete Containing Nano-Silica, 8. Evaluation
of Models by Soft Computing Techniques for the Prediction of Compressive
Strength of Concrete Using Steel Fibre, 9. Using Regression Model to
Estimate the Splitting Tensile Strength for the Concrete With Basalt Fiber
Reinforced Concrete, 10. Prediction of Compressive Strength of
Self-Compacting Concrete Containing Silica's Using Soft Computing
Techniques, 11. Using Soft Computing Techniques to Predict the Values of
Compressive Strength of Concrete with Basalt Fiber Reinforced Concrete, 12.
Soft Computing-Based Prediction of Compressive Strength of High Strength
Concrete, 13. Forecasting Compressive Strength of Concrete Containing
Nano-Silica Using Particle Swarm Optimization Algorithm and Genetic
Algorithm, 14. Prediction of Ultrasonic Pulse Velocity of Concrete, 15.
Evaluation of ANN and Tree-Based Techniques for Predicting the Compressive
Strength of Granite Powder Reinforced Concrete, 16. Predicting Recycled
Aggregates Compressive Strength in High-Performance Concrete Using
Artificial Neural Networks, 17. Compressive Strength Prediction and
Analysis of Concrete Using Hybrid Artificial Neural Networks
2. Developing Random Forest Random Tree and Linear Regression Model to
Predict Compressive Strength of Concrete Using Glass Fiber, 3. Prediction
of Compressive Strength at Elevated Temperatures Using Machine Learning
Methods, 4. Implementation of Machine Learning Approaches to Evaluate
Flexural Strength of Concrete With Glass Fiber, 5. A Comparative Study
Using ANFIS and ANN for Determining the Compressive Strength of Concrete,
6. Prediction of Concrete Mix Compressive Strength Using Waste Marble
Powder: A Comparison of ANN, RF, RT, and LR models, 7. Using GA to Predict
the Compressive Strength of Concrete Containing Nano-Silica, 8. Evaluation
of Models by Soft Computing Techniques for the Prediction of Compressive
Strength of Concrete Using Steel Fibre, 9. Using Regression Model to
Estimate the Splitting Tensile Strength for the Concrete With Basalt Fiber
Reinforced Concrete, 10. Prediction of Compressive Strength of
Self-Compacting Concrete Containing Silica's Using Soft Computing
Techniques, 11. Using Soft Computing Techniques to Predict the Values of
Compressive Strength of Concrete with Basalt Fiber Reinforced Concrete, 12.
Soft Computing-Based Prediction of Compressive Strength of High Strength
Concrete, 13. Forecasting Compressive Strength of Concrete Containing
Nano-Silica Using Particle Swarm Optimization Algorithm and Genetic
Algorithm, 14. Prediction of Ultrasonic Pulse Velocity of Concrete, 15.
Evaluation of ANN and Tree-Based Techniques for Predicting the Compressive
Strength of Granite Powder Reinforced Concrete, 16. Predicting Recycled
Aggregates Compressive Strength in High-Performance Concrete Using
Artificial Neural Networks, 17. Compressive Strength Prediction and
Analysis of Concrete Using Hybrid Artificial Neural Networks