Alle Infos zum eBook verschenken
- Format: ePub
- Merkliste
- Auf die Merkliste
- Bewerten Bewerten
- Teilen
- Produkt teilen
- Produkterinnerung
- Produkterinnerung
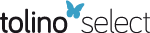
Hier können Sie sich einloggen
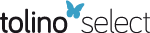
Bitte loggen Sie sich zunächst in Ihr Kundenkonto ein oder registrieren Sie sich bei bücher.de, um das eBook-Abo tolino select nutzen zu können.
A new edition of the definitive guide to logistic regression modeling for health science and other applications This thoroughly expanded Third Edition provides an easily accessible introduction to the logistic regression (LR) model and highlights the power of this model by examining the relationship between a dichotomous outcome and a set of covariables. Applied Logistic Regression, Third Edition emphasizes applications in the health sciences and handpicks topics that best suit the use of modern statistical software. The book provides readers with state-of-the-art techniques for building,…mehr
- Geräte: eReader
- mit Kopierschutz
- eBook Hilfe
- Größe: 21.63MB
- David W. HosmerApplied Logistic Regression (eBook, PDF)129,99 €
- Hossein RiazoshamsRobust Nonlinear Regression (eBook, ePUB)73,99 €
- Iain PardoeApplied Regression Modeling (eBook, ePUB)110,99 €
- Iain PardoeApplied Regression Modeling (eBook, ePUB)103,99 €
- Ali S. HadiRegression Analysis By Example Using R (eBook, ePUB)106,99 €
- Samprit ChatterjeeRegression Analysis by Example (eBook, ePUB)106,99 €
- Garrett M. FitzmauriceApplied Longitudinal Analysis (eBook, ePUB)124,99 €
-
-
-
Dieser Download kann aus rechtlichen Gründen nur mit Rechnungsadresse in A, B, BG, CY, CZ, D, DK, EW, E, FIN, F, GR, HR, H, IRL, I, LT, L, LR, M, NL, PL, P, R, S, SLO, SK ausgeliefert werden.
Hinweis: Dieser Artikel kann nur an eine deutsche Lieferadresse ausgeliefert werden.
- Produktdetails
- Verlag: Wiley
- Seitenzahl: 528
- Erscheinungstermin: 26. Februar 2013
- Englisch
- ISBN-13: 9781118548356
- Artikelnr.: 38244701
- Verlag: Wiley
- Seitenzahl: 528
- Erscheinungstermin: 26. Februar 2013
- Englisch
- ISBN-13: 9781118548356
- Artikelnr.: 38244701
- Herstellerkennzeichnung Die Herstellerinformationen sind derzeit nicht verfügbar.
1 Introduction to the Logistic Regression Model 1
1.1 Introduction 1
1.2 Fitting the Logistic Regression Model 8
1.3 Testing for the Significance of the Coefficients 10
1.4 Confidence Interval Estimation 15
1.5 Other Estimation Methods 20
1.6 Data Sets Used in Examples and Exercises 22
1.6.1 The ICU Study 22
1.6.2 The Low Birth Weight Study 24
1.6.3 The Global Longitudinal Study of Osteoporosis in Women 24
1.6.4 The Adolescent Placement Study 26
1.6.5 The Burn Injury Study 27
1.6.6 The Myopia Study 29
1.6.7 The NHANES Study 31
1.6.8 The Polypharmacy Study 31
Exercises 32
2 The Multiple Logistic Regression Model 35
2.1 Introduction 35
2.2 The Multiple Logistic Regression Model 35
2.3 Fitting the Multiple Logistic Regression Model 37
2.4 Testing for the Significance of the Model 39
2.5 Confidence Interval Estimation 42
2.6 Other Estimation Methods 45
Exercises 46
3 Interpretation of the Fitted Logistic Regression Model 49
3.1 Introduction 49
3.2 Dichotomous Independent Variable 50
3.3 Polychotomous Independent Variable 56
3.4 Continuous Independent Variable 62
3.5 Multivariable Models 64
3.6 Presentation and Interpretation of the Fitted Values 77
3.7 A Comparison of Logistic Regression and Stratified Analysis for 2 × 2
Tables 82
Exercises 87
4 Model-Building Strategies and Methods for Logistic Regression 89
4.1 Introduction 89
4.2 Purposeful Selection of Covariates 89
4.2.1 Methods to Examine the Scale of a Continuous Covariate in the Logit
94
4.2.2 Examples of Purposeful Selection 107
4.3 Other Methods for Selecting Covariates 124
4.3.1 Stepwise Selection of Covariates 125
4.3.2 Best Subsets Logistic Regression 133
4.3.3 Selecting Covariates and Checking their Scale Using Multivariable
Fractional Polynomials 139
4.4 Numerical Problems 145
Exercises 150
5 Assessing the Fit of the Model 153
5.1 Introduction 153
5.2 Summary Measures of Goodness of Fit 154
5.2.1 Pearson Chi-Square Statistic, Deviance, and Sum-of-Squares 155
5.2.2 The Hosmer-Lemeshow Tests 157
5.2.3 Classification Tables 169
5.2.4 Area Under the Receiver Operating Characteristic Curve 173
5.2.5 Other Summary Measures 182
5.3 Logistic Regression Diagnostics 186
5.4 Assessment of Fit via External Validation 202
5.5 Interpretation and Presentation of the Results from a Fitted Logistic
Regression Model 212
Exercises 223
6 Application of Logistic Regression with Different Sampling Models 227
6.1 Introduction 227
6.2 Cohort Studies 227
6.3 Case-Control Studies 229
6.4 Fitting Logistic Regression Models to Data from Complex Sample Surveys
233
Exercises 242
7 Logistic Regression for Matched Case-Control Studies 243
7.1 Introduction 243
7.2 Methods For Assessment of Fit in a 1-M Matched Study 248
7.3 An Example Using the Logistic Regression Model in a 1-1 Matched Study
251
7.4 An Example Using the Logistic Regression Model in a 1-M Matched Study
260
Exercises 267
8 Logistic Regression Models for Multinomial and Ordinal Outcomes 269
8.1 The Multinomial Logistic Regression Model 269
8.1.1 Introduction to the Model and Estimation of Model Parameters 269
8.1.2 Interpreting and Assessing the Significance of the Estimated
Coefficients 272
8.1.3 Model-Building Strategies for Multinomial Logistic Regression 278
8.1.4 Assessment of Fit and Diagnostic Statistics for the Multinomial
Logistic Regression Model 283
8.2 Ordinal Logistic Regression Models 289
8.2.1 Introduction to the Models, Methods for Fitting, and Interpretation
of Model Parameters 289
8.2.2 Model Building Strategies for Ordinal Logistic Regression Models 305
Exercises 310
9 Logistic Regression Models for the Analysis of Correlated Data 313
9.1 Introduction 313
9.2 Logistic Regression Models for the Analysis of Correlated Data 315
9.3 Estimation Methods for Correlated Data Logistic Regression Models 318
9.4 Interpretation of Coefficients from Logistic Regression Models for the
Analysis of Correlated Data 323
9.4.1 Population Average Model 324
9.4.2 Cluster-Specific Model 326
9.4.3 Alternative Estimation Methods for the Cluster-Specific Model 333
9.4.4 Comparison of Population Average and Cluster-Specific Model 334
9.5 An Example of Logistic Regression Modeling with Correlated Data 337
9.5.1 Choice of Model for Correlated Data Analysis 338
9.5.2 Population Average Model 339
9.5.3 Cluster-Specific Model 344
9.5.4 Additional Points to Consider when Fitting Logistic Regression Models
to Correlated Data 351
9.6 Assessment of Model Fit 354
9.6.1 Assessment of Population Average Model Fit 354
9.6.2 Assessment of Cluster-Specific Model Fit 365
9.6.3 Conclusions 374
Exercises 375
10 Special Topics 377
10.1 Introduction 377
10.2 Application of Propensity Score Methods in Logistic Regression
Modeling 377
10.3 Exact Methods for Logistic Regression Models 387
10.4 Missing Data 395
10.5 Sample Size Issues when Fitting Logistic Regression Models 401
10.6 Bayesian Methods for Logistic Regression 408
10.6.1 The Bayesian Logistic Regression Model 410
10.6.2 MCMC Simulation 411
10.6.3 An Example of a Bayesian Analysis and Its Interpretation 419
10.7 Other Link Functions for Binary Regression Models 434
10.8 Mediation 441
10.8.1 Distinguishing Mediators from Confounders 441
10.8.2 Implications for the Interpretation of an Adjusted Logistic
Regression Coefficient 443
10.8.3 Why Adjust for a Mediator? 444
10.8.4 Using Logistic Regression to Assess Mediation: Assumptions 445
10.9 More About Statistical Interaction 448
10.9.1 Additive versus Multiplicative Scale-Risk Difference versus Odds
Ratios 448
10.9.2 Estimating and Testing Additive Interaction 451
Exercises 456
References 459
Index 479
1 Introduction to the Logistic Regression Model 1
1.1 Introduction 1
1.2 Fitting the Logistic Regression Model 8
1.3 Testing for the Significance of the Coefficients 10
1.4 Confidence Interval Estimation 15
1.5 Other Estimation Methods 20
1.6 Data Sets Used in Examples and Exercises 22
1.6.1 The ICU Study 22
1.6.2 The Low Birth Weight Study 24
1.6.3 The Global Longitudinal Study of Osteoporosis in Women 24
1.6.4 The Adolescent Placement Study 26
1.6.5 The Burn Injury Study 27
1.6.6 The Myopia Study 29
1.6.7 The NHANES Study 31
1.6.8 The Polypharmacy Study 31
Exercises 32
2 The Multiple Logistic Regression Model 35
2.1 Introduction 35
2.2 The Multiple Logistic Regression Model 35
2.3 Fitting the Multiple Logistic Regression Model 37
2.4 Testing for the Significance of the Model 39
2.5 Confidence Interval Estimation 42
2.6 Other Estimation Methods 45
Exercises 46
3 Interpretation of the Fitted Logistic Regression Model 49
3.1 Introduction 49
3.2 Dichotomous Independent Variable 50
3.3 Polychotomous Independent Variable 56
3.4 Continuous Independent Variable 62
3.5 Multivariable Models 64
3.6 Presentation and Interpretation of the Fitted Values 77
3.7 A Comparison of Logistic Regression and Stratified Analysis for 2 × 2
Tables 82
Exercises 87
4 Model-Building Strategies and Methods for Logistic Regression 89
4.1 Introduction 89
4.2 Purposeful Selection of Covariates 89
4.2.1 Methods to Examine the Scale of a Continuous Covariate in the Logit
94
4.2.2 Examples of Purposeful Selection 107
4.3 Other Methods for Selecting Covariates 124
4.3.1 Stepwise Selection of Covariates 125
4.3.2 Best Subsets Logistic Regression 133
4.3.3 Selecting Covariates and Checking their Scale Using Multivariable
Fractional Polynomials 139
4.4 Numerical Problems 145
Exercises 150
5 Assessing the Fit of the Model 153
5.1 Introduction 153
5.2 Summary Measures of Goodness of Fit 154
5.2.1 Pearson Chi-Square Statistic, Deviance, and Sum-of-Squares 155
5.2.2 The Hosmer-Lemeshow Tests 157
5.2.3 Classification Tables 169
5.2.4 Area Under the Receiver Operating Characteristic Curve 173
5.2.5 Other Summary Measures 182
5.3 Logistic Regression Diagnostics 186
5.4 Assessment of Fit via External Validation 202
5.5 Interpretation and Presentation of the Results from a Fitted Logistic
Regression Model 212
Exercises 223
6 Application of Logistic Regression with Different Sampling Models 227
6.1 Introduction 227
6.2 Cohort Studies 227
6.3 Case-Control Studies 229
6.4 Fitting Logistic Regression Models to Data from Complex Sample Surveys
233
Exercises 242
7 Logistic Regression for Matched Case-Control Studies 243
7.1 Introduction 243
7.2 Methods For Assessment of Fit in a 1-M Matched Study 248
7.3 An Example Using the Logistic Regression Model in a 1-1 Matched Study
251
7.4 An Example Using the Logistic Regression Model in a 1-M Matched Study
260
Exercises 267
8 Logistic Regression Models for Multinomial and Ordinal Outcomes 269
8.1 The Multinomial Logistic Regression Model 269
8.1.1 Introduction to the Model and Estimation of Model Parameters 269
8.1.2 Interpreting and Assessing the Significance of the Estimated
Coefficients 272
8.1.3 Model-Building Strategies for Multinomial Logistic Regression 278
8.1.4 Assessment of Fit and Diagnostic Statistics for the Multinomial
Logistic Regression Model 283
8.2 Ordinal Logistic Regression Models 289
8.2.1 Introduction to the Models, Methods for Fitting, and Interpretation
of Model Parameters 289
8.2.2 Model Building Strategies for Ordinal Logistic Regression Models 305
Exercises 310
9 Logistic Regression Models for the Analysis of Correlated Data 313
9.1 Introduction 313
9.2 Logistic Regression Models for the Analysis of Correlated Data 315
9.3 Estimation Methods for Correlated Data Logistic Regression Models 318
9.4 Interpretation of Coefficients from Logistic Regression Models for the
Analysis of Correlated Data 323
9.4.1 Population Average Model 324
9.4.2 Cluster-Specific Model 326
9.4.3 Alternative Estimation Methods for the Cluster-Specific Model 333
9.4.4 Comparison of Population Average and Cluster-Specific Model 334
9.5 An Example of Logistic Regression Modeling with Correlated Data 337
9.5.1 Choice of Model for Correlated Data Analysis 338
9.5.2 Population Average Model 339
9.5.3 Cluster-Specific Model 344
9.5.4 Additional Points to Consider when Fitting Logistic Regression Models
to Correlated Data 351
9.6 Assessment of Model Fit 354
9.6.1 Assessment of Population Average Model Fit 354
9.6.2 Assessment of Cluster-Specific Model Fit 365
9.6.3 Conclusions 374
Exercises 375
10 Special Topics 377
10.1 Introduction 377
10.2 Application of Propensity Score Methods in Logistic Regression
Modeling 377
10.3 Exact Methods for Logistic Regression Models 387
10.4 Missing Data 395
10.5 Sample Size Issues when Fitting Logistic Regression Models 401
10.6 Bayesian Methods for Logistic Regression 408
10.6.1 The Bayesian Logistic Regression Model 410
10.6.2 MCMC Simulation 411
10.6.3 An Example of a Bayesian Analysis and Its Interpretation 419
10.7 Other Link Functions for Binary Regression Models 434
10.8 Mediation 441
10.8.1 Distinguishing Mediators from Confounders 441
10.8.2 Implications for the Interpretation of an Adjusted Logistic
Regression Coefficient 443
10.8.3 Why Adjust for a Mediator? 444
10.8.4 Using Logistic Regression to Assess Mediation: Assumptions 445
10.9 More About Statistical Interaction 448
10.9.1 Additive versus Multiplicative Scale-Risk Difference versus Odds
Ratios 448
10.9.2 Estimating and Testing Additive Interaction 451
Exercises 456
References 459
Index 479