Applied Statistics (eBook, ePUB)
Theory and Problem Solutions with R
Alle Infos zum eBook verschenken
Applied Statistics (eBook, ePUB)
Theory and Problem Solutions with R
- Format: ePub
- Merkliste
- Auf die Merkliste
- Bewerten Bewerten
- Teilen
- Produkt teilen
- Produkterinnerung
- Produkterinnerung
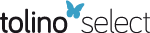
Hier können Sie sich einloggen
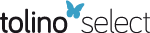
Bitte loggen Sie sich zunächst in Ihr Kundenkonto ein oder registrieren Sie sich bei bücher.de, um das eBook-Abo tolino select nutzen zu können.
Instructs readers on how to use methods of statistics and experimental design with R software Applied statistics covers both the theory and the application of modern statistical and mathematical modelling techniques to applied problems in industry, public services, commerce, and research. It proceeds from a strong theoretical background, but it is practically oriented to develop one's ability to tackle new and non-standard problems confidently. Taking a practical approach to applied statistics, this user-friendly guide teaches readers how to use methods of statistics and experimental design…mehr
- Geräte: eReader
- mit Kopierschutz
- eBook Hilfe
- Größe: 10.4MB
- P. C. G. VassiliouDiscrete-time Asset Pricing Models in Applied Stochastic Finance (eBook, ePUB)160,99 €
- Dieter RaschMathematical Statistics (eBook, ePUB)70,99 €
- N. W. GalweyIntroduction to Mixed Modelling (eBook, ePUB)78,99 €
- Joseph SchmullerR Projects For Dummies (eBook, ePUB)20,99 €
- Prakash GorroochurnClassic Problems of Probability (eBook, ePUB)57,99 €
- Anatoliy PogoruiRandom Motions in Markov and Semi-Markov Random Environments 1 (eBook, ePUB)139,99 €
- David W. HosmerApplied Survival Analysis (eBook, ePUB)139,99 €
-
-
-
Dieser Download kann aus rechtlichen Gründen nur mit Rechnungsadresse in A, B, BG, CY, CZ, D, DK, EW, E, FIN, F, GR, HR, H, IRL, I, LT, L, LR, M, NL, PL, P, R, S, SLO, SK ausgeliefert werden.
- Produktdetails
- Verlag: John Wiley & Sons
- Seitenzahl: 512
- Erscheinungstermin: 14. August 2019
- Englisch
- ISBN-13: 9781119551546
- Artikelnr.: 57625753
- Verlag: John Wiley & Sons
- Seitenzahl: 512
- Erscheinungstermin: 14. August 2019
- Englisch
- ISBN-13: 9781119551546
- Artikelnr.: 57625753
- Herstellerkennzeichnung Die Herstellerinformationen sind derzeit nicht verfügbar.
B) 131 5.5 Three-Way Classification 134 5.5.1 Complete Cross-Classification (A×B ×C) 135 5.5.2 Nested Classification (C
B
A) 144 5.5.3 Mixed Classifications 147 5.5.3.1 Cross-Classification between Two Factors where One of Them Is Sub-Ordinated to a Third Factor ((B
A)xC) 148 5.5.3.2 Cross-Classification of Two Factors, in which a Third Factor is Nested (C
(A× B)) 153 References 157 6 Analysis of Variance -Models with Random Effects 159 6.1 Introduction 159 6.2 One-Way Classification 159 6.2.1 Estimation of the Variance Components 160 6.2.1.1 ANOVA Method 160 6.2.1.2 Maximum Likelihood Method 164 6.2.1.3 REML - Estimation 166 6.2.2 Tests of Hypotheses and Confidence Intervals 169 6.2.3 Expectation and Variances of the ANOVA Estimators 174 6.3 Two-Way Classification 176 6.3.1 Two-Way Cross Classification 176 6.3.2 Two-Way Nested Classification 182 6.4 Three-Way Classification 186 6.4.1 Three-Way Cross-Classification with Equal Sub-Class Numbers 186 6.4.2 Three-Way Nested Classification 192 6.4.3 Three-Way Mixed Classifications 195 6.4.3.1 Cross-Classification Between Two Factors Where One of Them is Sub-Ordinated to a Third Factor ((B
A)×C) 195 6.4.3.2 Cross-Classification of Two Factors in Which a Third Factor is Nested (C
(A×B)) 197 References 199 7 Analysis of Variance -Mixed Models 201 7.1 Introduction 201 7.2 Two-Way Classification 201 7.2.1 Balanced Two-Way Cross-Classification 201 7.2.2 Two-Way Nested Classification 214 7.3 Three-Way Layout 223 7.3.1 Three-Way Analysis of Variance - Cross-Classification A × B × C 223 7.3.2 Three-Way Analysis of Variance - Nested Classification A
B
C 230 7.3.2.1 Three-Way Analysis of Variance - Nested Classification - Model III - Balanced Case 230 7.3.2.2 Three-Way Analysis of Variance - Nested Classification - Model IV - Balanced Case 232 7.3.2.3 Three-Way Analysis of Variance - Nested Classification - Model V - Balanced Case 234 7.3.2.4 Three-Way Analysis of Variance - Nested Classification - Model VI - Balanced Case 236 7.3.2.5 Three-Way Analysis of Variance - Nested Classification - Model VII - Balanced Case 237 7.3.2.6 Three-Way Analysis of Variance - Nested Classification - Model VIII - Balanced Case 238 7.3.3 Three-Way Analysis of Variance - Mixed Classification - (A× B)
C 239 7.3.3.1 Three-Way Analysis of Variance - Mixed Classification - (A× B)
C Model III 239 7.3.3.2 Three-Way Analysis of Variance - Mixed Classification - (A× B)
C Model IV 242 7.3.3.3 Three-Way Analysis of Variance - Mixed Classification - (A× B)
C Model V 243 7.3.3.4 Three-Way Analysis of Variance - Mixed Classification - (A× B)
C Model VI 245 7.3.4 Three-Way Analysis of Variance - Mixed Classification - (A
B) ×C 247 7.3.4.1 Three-Way Analysis of Variance - Mixed Classification - (A
B) ×C Model III 247 7.3.4.2 Three-Way Analysis of Variance - Mixed Classification - (A
B) ×C Model IV 249 7.3.4.3 Three-Way Analysis of Variance - Mixed Classification - (A
B) ×C Model V 251 7.3.4.4 Three-Way Analysis of Variance - Mixed Classification - (A
B) ×C Model VI 253 7.3.4.5 Three-Way Analysis of Variance - Mixed Classification - (A
B) ×C model VII 254 7.3.4.6 Three-Way Analysis of Variance - Mixed Classification - (A
B) ×C Model VIII 255 References 256 8 Regression Analysis 257 8.1 Introduction 257 8.2 Regression with Non-Random Regressors - Model I of Regression 262 8.2.1 Linear and Quasilinear Regression 262 8.2.1.1 Parameter Estimation 263 8.2.1.2 Confidence Intervals and Hypotheses Testing 274 8.2.2 Intrinsically Non-Linear Regression 282 8.2.2.1 The Asymptotic Distribution of the Least Squares Estimators 283 8.2.2.2 The Michaelis-Menten Regression 285 8.2.2.3 Exponential Regression 290 8.2.2.4 The Logistic Regression 298 8.2.2.5 The Bertalanffy Function 306 8.2.2.6 The Gompertz Function 312 8.2.3 Optimal Experimental Designs 315 8.2.3.1 Simple Linear and Quasilinear Regression 316 8.2.3.2 Intrinsically Non-linear Regression 317 8.2.3.3 The Michaelis-Menten Regression 319 8.2.3.4 Exponential Regression 319 8.2.3.5 The Logistic Regression 320 8.2.3.6 The Bertalanffy Function 321 8.2.3.7 The Gompertz Function 321 8.3 Models with Random Regressors 322 8.3.1 The Simple Linear Case 322 8.3.2 The Multiple Linear Case and the Quasilinear Case 330 8.3.2.1 Hypotheses Testing - General 333 8.3.2.2 Confidence Estimation 333 8.3.3 The Allometric Model 334 8.3.4 Experimental Designs 335 References 335 9 Analysis of Covariance (ANCOVA) 339 9.1 Introduction 339 9.2 Completely Randomised Design with Covariate 340 9.2.1 Balanced Completely Randomised Design 340 9.2.2 Unbalanced Completely Randomised Design 350 9.3 Randomised Complete Block Design with Covariate 358 9.4 Concluding Remarks 365 References 366 10 Multiple Decision Problems 367 10.1 Introduction 367 10.2 Selection Procedures 367 10.2.1 The Indifference Zone Formulation for Selecting Expectations 368 10.2.1.1 Indifference Zone Selection,
2 Known 368 10.2.1.2 Indifference Zone Selection,
2 Unknown 371 10.3 The Subset Selection Procedure for Expectations 371 10.4 Optimal Combination of the Indifference Zone and the Subset Selection Procedure 372 10.5 Selection of the Normal Distribution with the Smallest Variance 375 10.6 Multiple Comparisons 375 10.6.1 The Solution of MC Problem 10.1 377 10.6.1.1 The F-test for MC Problem 10.1 377 10.6.1.2 Scheffé's Method for MC Problem 10.1 378 10.6.1.3 Bonferroni's Method for MC Problem 10.1 379 10.6.1.4 Tukey's Method for MC Problem 10.1 for ni = n 382 10.6.1.5 Generalised Tukey's Method for MC Problem 10.1 for ni
n 383 10.6.2 The Solution of MC Problem 10.2 - the Multiple t-Test 384 10.6.3 The Solution of MC Problem 10.3 - Pairwise and Simultaneous Comparisons with a Control 385 10.6.3.1 Pairwise Comparisons - The Multiple t-Test 385 10.6.3.2 Simultaneous Comparisons -The Dunnett Method 387 References 390 11 Generalised Linear Models 393 11.1 Introduction 393 11.2 Exponential Families of Distributions 394 11.3 Generalised Linear Models - An Overview 396 11.4 Analysis - Fitting a GLM - The Linear Case 398 11.5 Binary Logistic Regression 399 11.5.1 Analysis 400 11.5.2 Overdispersion 408 11.6 Poisson Regression 411 11.6.1 Analysis 411 11.6.2 Overdispersion 417 11.7 The Gamma Regression 417 11.8 GLM for Gamma Regression 418 11.9 GLM for the Multinomial Distribution 425 References 428 12 Spatial Statistics 429 12.1 Introduction 429 12.2 Geostatistics 431 12.2.1 Semi-variogram Function 432 12.2.2 Semi-variogram Parameter Estimation 439 12.2.3 Kriging 440 12.2.4 Trans-Gaussian Kriging 446 12.3 Special Problems and Outlook 450 12.3.1 Generalised Linear Models in Geostatistics 450 12.3.2 Copula Based Geostatistical Prediction 451 References 451 Appendix A List of Problems 455 Appendix B Symbolism 483 Appendix C Abbreviations 485 Appendix D Probability and Density Functions 487 Index 489
B) 131 5.5 Three-Way Classification 134 5.5.1 Complete Cross-Classification (A×B ×C) 135 5.5.2 Nested Classification (C
B
A) 144 5.5.3 Mixed Classifications 147 5.5.3.1 Cross-Classification between Two Factors where One of Them Is Sub-Ordinated to a Third Factor ((B
A)xC) 148 5.5.3.2 Cross-Classification of Two Factors, in which a Third Factor is Nested (C
(A× B)) 153 References 157 6 Analysis of Variance -Models with Random Effects 159 6.1 Introduction 159 6.2 One-Way Classification 159 6.2.1 Estimation of the Variance Components 160 6.2.1.1 ANOVA Method 160 6.2.1.2 Maximum Likelihood Method 164 6.2.1.3 REML - Estimation 166 6.2.2 Tests of Hypotheses and Confidence Intervals 169 6.2.3 Expectation and Variances of the ANOVA Estimators 174 6.3 Two-Way Classification 176 6.3.1 Two-Way Cross Classification 176 6.3.2 Two-Way Nested Classification 182 6.4 Three-Way Classification 186 6.4.1 Three-Way Cross-Classification with Equal Sub-Class Numbers 186 6.4.2 Three-Way Nested Classification 192 6.4.3 Three-Way Mixed Classifications 195 6.4.3.1 Cross-Classification Between Two Factors Where One of Them is Sub-Ordinated to a Third Factor ((B
A)×C) 195 6.4.3.2 Cross-Classification of Two Factors in Which a Third Factor is Nested (C
(A×B)) 197 References 199 7 Analysis of Variance -Mixed Models 201 7.1 Introduction 201 7.2 Two-Way Classification 201 7.2.1 Balanced Two-Way Cross-Classification 201 7.2.2 Two-Way Nested Classification 214 7.3 Three-Way Layout 223 7.3.1 Three-Way Analysis of Variance - Cross-Classification A × B × C 223 7.3.2 Three-Way Analysis of Variance - Nested Classification A
B
C 230 7.3.2.1 Three-Way Analysis of Variance - Nested Classification - Model III - Balanced Case 230 7.3.2.2 Three-Way Analysis of Variance - Nested Classification - Model IV - Balanced Case 232 7.3.2.3 Three-Way Analysis of Variance - Nested Classification - Model V - Balanced Case 234 7.3.2.4 Three-Way Analysis of Variance - Nested Classification - Model VI - Balanced Case 236 7.3.2.5 Three-Way Analysis of Variance - Nested Classification - Model VII - Balanced Case 237 7.3.2.6 Three-Way Analysis of Variance - Nested Classification - Model VIII - Balanced Case 238 7.3.3 Three-Way Analysis of Variance - Mixed Classification - (A× B)
C 239 7.3.3.1 Three-Way Analysis of Variance - Mixed Classification - (A× B)
C Model III 239 7.3.3.2 Three-Way Analysis of Variance - Mixed Classification - (A× B)
C Model IV 242 7.3.3.3 Three-Way Analysis of Variance - Mixed Classification - (A× B)
C Model V 243 7.3.3.4 Three-Way Analysis of Variance - Mixed Classification - (A× B)
C Model VI 245 7.3.4 Three-Way Analysis of Variance - Mixed Classification - (A
B) ×C 247 7.3.4.1 Three-Way Analysis of Variance - Mixed Classification - (A
B) ×C Model III 247 7.3.4.2 Three-Way Analysis of Variance - Mixed Classification - (A
B) ×C Model IV 249 7.3.4.3 Three-Way Analysis of Variance - Mixed Classification - (A
B) ×C Model V 251 7.3.4.4 Three-Way Analysis of Variance - Mixed Classification - (A
B) ×C Model VI 253 7.3.4.5 Three-Way Analysis of Variance - Mixed Classification - (A
B) ×C model VII 254 7.3.4.6 Three-Way Analysis of Variance - Mixed Classification - (A
B) ×C Model VIII 255 References 256 8 Regression Analysis 257 8.1 Introduction 257 8.2 Regression with Non-Random Regressors - Model I of Regression 262 8.2.1 Linear and Quasilinear Regression 262 8.2.1.1 Parameter Estimation 263 8.2.1.2 Confidence Intervals and Hypotheses Testing 274 8.2.2 Intrinsically Non-Linear Regression 282 8.2.2.1 The Asymptotic Distribution of the Least Squares Estimators 283 8.2.2.2 The Michaelis-Menten Regression 285 8.2.2.3 Exponential Regression 290 8.2.2.4 The Logistic Regression 298 8.2.2.5 The Bertalanffy Function 306 8.2.2.6 The Gompertz Function 312 8.2.3 Optimal Experimental Designs 315 8.2.3.1 Simple Linear and Quasilinear Regression 316 8.2.3.2 Intrinsically Non-linear Regression 317 8.2.3.3 The Michaelis-Menten Regression 319 8.2.3.4 Exponential Regression 319 8.2.3.5 The Logistic Regression 320 8.2.3.6 The Bertalanffy Function 321 8.2.3.7 The Gompertz Function 321 8.3 Models with Random Regressors 322 8.3.1 The Simple Linear Case 322 8.3.2 The Multiple Linear Case and the Quasilinear Case 330 8.3.2.1 Hypotheses Testing - General 333 8.3.2.2 Confidence Estimation 333 8.3.3 The Allometric Model 334 8.3.4 Experimental Designs 335 References 335 9 Analysis of Covariance (ANCOVA) 339 9.1 Introduction 339 9.2 Completely Randomised Design with Covariate 340 9.2.1 Balanced Completely Randomised Design 340 9.2.2 Unbalanced Completely Randomised Design 350 9.3 Randomised Complete Block Design with Covariate 358 9.4 Concluding Remarks 365 References 366 10 Multiple Decision Problems 367 10.1 Introduction 367 10.2 Selection Procedures 367 10.2.1 The Indifference Zone Formulation for Selecting Expectations 368 10.2.1.1 Indifference Zone Selection,
2 Known 368 10.2.1.2 Indifference Zone Selection,
2 Unknown 371 10.3 The Subset Selection Procedure for Expectations 371 10.4 Optimal Combination of the Indifference Zone and the Subset Selection Procedure 372 10.5 Selection of the Normal Distribution with the Smallest Variance 375 10.6 Multiple Comparisons 375 10.6.1 The Solution of MC Problem 10.1 377 10.6.1.1 The F-test for MC Problem 10.1 377 10.6.1.2 Scheffé's Method for MC Problem 10.1 378 10.6.1.3 Bonferroni's Method for MC Problem 10.1 379 10.6.1.4 Tukey's Method for MC Problem 10.1 for ni = n 382 10.6.1.5 Generalised Tukey's Method for MC Problem 10.1 for ni
n 383 10.6.2 The Solution of MC Problem 10.2 - the Multiple t-Test 384 10.6.3 The Solution of MC Problem 10.3 - Pairwise and Simultaneous Comparisons with a Control 385 10.6.3.1 Pairwise Comparisons - The Multiple t-Test 385 10.6.3.2 Simultaneous Comparisons -The Dunnett Method 387 References 390 11 Generalised Linear Models 393 11.1 Introduction 393 11.2 Exponential Families of Distributions 394 11.3 Generalised Linear Models - An Overview 396 11.4 Analysis - Fitting a GLM - The Linear Case 398 11.5 Binary Logistic Regression 399 11.5.1 Analysis 400 11.5.2 Overdispersion 408 11.6 Poisson Regression 411 11.6.1 Analysis 411 11.6.2 Overdispersion 417 11.7 The Gamma Regression 417 11.8 GLM for Gamma Regression 418 11.9 GLM for the Multinomial Distribution 425 References 428 12 Spatial Statistics 429 12.1 Introduction 429 12.2 Geostatistics 431 12.2.1 Semi-variogram Function 432 12.2.2 Semi-variogram Parameter Estimation 439 12.2.3 Kriging 440 12.2.4 Trans-Gaussian Kriging 446 12.3 Special Problems and Outlook 450 12.3.1 Generalised Linear Models in Geostatistics 450 12.3.2 Copula Based Geostatistical Prediction 451 References 451 Appendix A List of Problems 455 Appendix B Symbolism 483 Appendix C Abbreviations 485 Appendix D Probability and Density Functions 487 Index 489