Victor Parada
Automatic Generation Of Algorithms (eBook, PDF)
201,95 €
201,95 €
inkl. MwSt.
Sofort per Download lieferbar
101 °P sammeln
201,95 €
Als Download kaufen
201,95 €
inkl. MwSt.
Sofort per Download lieferbar
101 °P sammeln
Jetzt verschenken
Alle Infos zum eBook verschenken
201,95 €
inkl. MwSt.
Sofort per Download lieferbar
Alle Infos zum eBook verschenken
101 °P sammeln
Victor Parada
Automatic Generation Of Algorithms (eBook, PDF)
- Format: PDF
- Merkliste
- Auf die Merkliste
- Bewerten Bewerten
- Teilen
- Produkt teilen
- Produkterinnerung
- Produkterinnerung
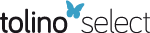
Bitte loggen Sie sich zunächst in Ihr Kundenkonto ein oder registrieren Sie sich bei
bücher.de, um das eBook-Abo tolino select nutzen zu können.
Hier können Sie sich einloggen
Hier können Sie sich einloggen
Sie sind bereits eingeloggt. Klicken Sie auf 2. tolino select Abo, um fortzufahren.
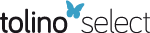
Bitte loggen Sie sich zunächst in Ihr Kundenkonto ein oder registrieren Sie sich bei bücher.de, um das eBook-Abo tolino select nutzen zu können.
This book is structured to guide readers through AGA's concepts, practical applications, and future directions.At its core, this book is an invitation to reimagine the boundaries of algorithmic design. It is crafted for researchers, practitioners, and enthusiasts in computer science, operations research, AI, and beyond.
- Geräte: PC
- mit Kopierschutz
- eBook Hilfe
Andere Kunden interessierten sich auch für
- Victor ParadaAutomatic Generation Of Algorithms (eBook, ePUB)201,95 €
- Handbook of Graph Theory (eBook, PDF)222,95 €
- Jitendra KumarMachine Learning for Cloud Management (eBook, PDF)59,95 €
- Antoine JouxAlgorithmic Cryptanalysis (eBook, PDF)140,95 €
- Vinod Kumar ChauhanStochastic Optimization for Large-scale Machine Learning (eBook, PDF)54,95 €
- Bozzano G LuisaHandbook of Combinatorics Volume 1 (eBook, PDF)139,95 €
- Roberto TogneriFundamentals of Information Theory and Coding Design (eBook, PDF)125,95 €
-
-
-
This book is structured to guide readers through AGA's concepts, practical applications, and future directions.At its core, this book is an invitation to reimagine the boundaries of algorithmic design. It is crafted for researchers, practitioners, and enthusiasts in computer science, operations research, AI, and beyond.
Hinweis: Dieser Artikel kann nur an eine deutsche Lieferadresse ausgeliefert werden.
Dieser Download kann aus rechtlichen Gründen nur mit Rechnungsadresse in A, B, BG, CY, CZ, D, DK, EW, E, FIN, F, GR, HR, H, IRL, I, LT, L, LR, M, NL, PL, P, R, S, SLO, SK ausgeliefert werden.
Hinweis: Dieser Artikel kann nur an eine deutsche Lieferadresse ausgeliefert werden.
Produktdetails
- Produktdetails
- Verlag: Taylor & Francis eBooks
- Seitenzahl: 214
- Erscheinungstermin: 10. Februar 2025
- Englisch
- ISBN-13: 9781040307069
- Artikelnr.: 72615846
- Verlag: Taylor & Francis eBooks
- Seitenzahl: 214
- Erscheinungstermin: 10. Februar 2025
- Englisch
- ISBN-13: 9781040307069
- Artikelnr.: 72615846
- Herstellerkennzeichnung Die Herstellerinformationen sind derzeit nicht verfügbar.
Victor Parada is Titular Professor in the Informatics Engineering Department at the University of Santiago, Chile.
1. Overview of Optimization
1.1 Introduction
1.2 Combinatorial Optimization
1.3 NP-hardness
1.4 NP-hardness in Combinatorial Optimization
1.5 General Framework of a Combinatorial Optimization Problem
1.6 Methods for Combinatorial Optimization Problems
1.7 A General Optimization Algorithm
1.8 Summary
2. The Master Problem
2.1 Introduction
2.2 The Problem Statement
2.3 The Space of Instances
2.4 The Space of Algorithms
2.5 The Space of Parameters
2.6 Simultaneous Parameter Optimization with AGA
2.7 Independent Parameter Optimization
2.8 The Algorithm Selection Problem
2.9 The No-Free-Lunch in AGA
2.10 Summary
3. Modeling Problems
3.1 The Modeling Process
3.2 Identifying Problems
3.3 Approaching Practical Problems in Operations Research
3.4 Fundamental Models in OR
3.5 Approaching Practical Problems via AI
3.6 Fundamental Models in AI
3.7 Approaching Practical Problems by AGA
3.8 A Fundamental AGA Model
3.9 Summary
4. AGA with Genetic Programming
4.1 Introduction
4.2 The Master Problem in AGA
4.3 Genetic Programming
4.3.1 The General Algorithmic Evolutionary Process
4.3.2 Genetic Operations in GP
4.4 GP as a Metaheuristic
4.5 Modeling the Master Problem with GP
4.5.1 Solution Representation as a Tree
4.5.2 The Fitness Function
4.6 The Evolution of Algorithms
4.7 Constructive and Refinement Algorithms
4.8 Robustness Versus Specialization
4.9 AGA for Population-Based Algorithms
4.10 Rediscovering Algorithms
4.11 AGA Specification Sheet
4.12 Summary
5. AGA and Machine Learning
5.1 Introduction
5.2 Schematic Overview of Machine Learning
5.2.1 Modeling a Practical Problem
5.2.2 Meaning of the Dataset
5.2.3 Hypothetical Model
5.2.4 The Optimization Problem
5.2.5 Algorithms for the Optimization Problem
5.3 Types of Problems in Machine Learning
5.4 Schematic Overview of the Automatic Generation of Algorithms
5.4.1 Problem Instances
5.4.2 Algorithmic Components
5.4.3 The Master Problem
5.4.4 The Resulting Algorithm
5.5 Symbolic Regression
5.6 Summary
6. Producing Metaheuristics Automatically
6.1 Metaheuristics and AGA
6.2 Types of Metaheuristics
6.3 Key Concepts in Metaheuristics
6.4 Solution Container Definition
6.5 Terminals Definition
6.6 Defining Terminals for AGM
6.7 Possible Combinations
6.8 Summary
7. AGA with Reinforcement Learning
7.1 Introduction
7.2 Dynamic Programming
7.3 Bellman's Principle of Optimality
7.4 Dynamic Programming Algorithms
7.5 Dynamic Programming Approaches
7.6 Deciding Agent Problem
7.7 AGA with Reinforcement Learning
7.7.1 Introduction
7.7.2 RL Algorithms
7.7.3 Modeling the Automatic Generation of Algorithms as a DAP
7.8 Summary
8.Conclusions and Future Trends
8.1 Introduction
8.2 Future Directions and Research Paths
8.3 Closing Thoughts
1.1 Introduction
1.2 Combinatorial Optimization
1.3 NP-hardness
1.4 NP-hardness in Combinatorial Optimization
1.5 General Framework of a Combinatorial Optimization Problem
1.6 Methods for Combinatorial Optimization Problems
1.7 A General Optimization Algorithm
1.8 Summary
2. The Master Problem
2.1 Introduction
2.2 The Problem Statement
2.3 The Space of Instances
2.4 The Space of Algorithms
2.5 The Space of Parameters
2.6 Simultaneous Parameter Optimization with AGA
2.7 Independent Parameter Optimization
2.8 The Algorithm Selection Problem
2.9 The No-Free-Lunch in AGA
2.10 Summary
3. Modeling Problems
3.1 The Modeling Process
3.2 Identifying Problems
3.3 Approaching Practical Problems in Operations Research
3.4 Fundamental Models in OR
3.5 Approaching Practical Problems via AI
3.6 Fundamental Models in AI
3.7 Approaching Practical Problems by AGA
3.8 A Fundamental AGA Model
3.9 Summary
4. AGA with Genetic Programming
4.1 Introduction
4.2 The Master Problem in AGA
4.3 Genetic Programming
4.3.1 The General Algorithmic Evolutionary Process
4.3.2 Genetic Operations in GP
4.4 GP as a Metaheuristic
4.5 Modeling the Master Problem with GP
4.5.1 Solution Representation as a Tree
4.5.2 The Fitness Function
4.6 The Evolution of Algorithms
4.7 Constructive and Refinement Algorithms
4.8 Robustness Versus Specialization
4.9 AGA for Population-Based Algorithms
4.10 Rediscovering Algorithms
4.11 AGA Specification Sheet
4.12 Summary
5. AGA and Machine Learning
5.1 Introduction
5.2 Schematic Overview of Machine Learning
5.2.1 Modeling a Practical Problem
5.2.2 Meaning of the Dataset
5.2.3 Hypothetical Model
5.2.4 The Optimization Problem
5.2.5 Algorithms for the Optimization Problem
5.3 Types of Problems in Machine Learning
5.4 Schematic Overview of the Automatic Generation of Algorithms
5.4.1 Problem Instances
5.4.2 Algorithmic Components
5.4.3 The Master Problem
5.4.4 The Resulting Algorithm
5.5 Symbolic Regression
5.6 Summary
6. Producing Metaheuristics Automatically
6.1 Metaheuristics and AGA
6.2 Types of Metaheuristics
6.3 Key Concepts in Metaheuristics
6.4 Solution Container Definition
6.5 Terminals Definition
6.6 Defining Terminals for AGM
6.7 Possible Combinations
6.8 Summary
7. AGA with Reinforcement Learning
7.1 Introduction
7.2 Dynamic Programming
7.3 Bellman's Principle of Optimality
7.4 Dynamic Programming Algorithms
7.5 Dynamic Programming Approaches
7.6 Deciding Agent Problem
7.7 AGA with Reinforcement Learning
7.7.1 Introduction
7.7.2 RL Algorithms
7.7.3 Modeling the Automatic Generation of Algorithms as a DAP
7.8 Summary
8.Conclusions and Future Trends
8.1 Introduction
8.2 Future Directions and Research Paths
8.3 Closing Thoughts
1. Overview of Optimization
1.1 Introduction
1.2 Combinatorial Optimization
1.3 NP-hardness
1.4 NP-hardness in Combinatorial Optimization
1.5 General Framework of a Combinatorial Optimization Problem
1.6 Methods for Combinatorial Optimization Problems
1.7 A General Optimization Algorithm
1.8 Summary
2. The Master Problem
2.1 Introduction
2.2 The Problem Statement
2.3 The Space of Instances
2.4 The Space of Algorithms
2.5 The Space of Parameters
2.6 Simultaneous Parameter Optimization with AGA
2.7 Independent Parameter Optimization
2.8 The Algorithm Selection Problem
2.9 The No-Free-Lunch in AGA
2.10 Summary
3. Modeling Problems
3.1 The Modeling Process
3.2 Identifying Problems
3.3 Approaching Practical Problems in Operations Research
3.4 Fundamental Models in OR
3.5 Approaching Practical Problems via AI
3.6 Fundamental Models in AI
3.7 Approaching Practical Problems by AGA
3.8 A Fundamental AGA Model
3.9 Summary
4. AGA with Genetic Programming
4.1 Introduction
4.2 The Master Problem in AGA
4.3 Genetic Programming
4.3.1 The General Algorithmic Evolutionary Process
4.3.2 Genetic Operations in GP
4.4 GP as a Metaheuristic
4.5 Modeling the Master Problem with GP
4.5.1 Solution Representation as a Tree
4.5.2 The Fitness Function
4.6 The Evolution of Algorithms
4.7 Constructive and Refinement Algorithms
4.8 Robustness Versus Specialization
4.9 AGA for Population-Based Algorithms
4.10 Rediscovering Algorithms
4.11 AGA Specification Sheet
4.12 Summary
5. AGA and Machine Learning
5.1 Introduction
5.2 Schematic Overview of Machine Learning
5.2.1 Modeling a Practical Problem
5.2.2 Meaning of the Dataset
5.2.3 Hypothetical Model
5.2.4 The Optimization Problem
5.2.5 Algorithms for the Optimization Problem
5.3 Types of Problems in Machine Learning
5.4 Schematic Overview of the Automatic Generation of Algorithms
5.4.1 Problem Instances
5.4.2 Algorithmic Components
5.4.3 The Master Problem
5.4.4 The Resulting Algorithm
5.5 Symbolic Regression
5.6 Summary
6. Producing Metaheuristics Automatically
6.1 Metaheuristics and AGA
6.2 Types of Metaheuristics
6.3 Key Concepts in Metaheuristics
6.4 Solution Container Definition
6.5 Terminals Definition
6.6 Defining Terminals for AGM
6.7 Possible Combinations
6.8 Summary
7. AGA with Reinforcement Learning
7.1 Introduction
7.2 Dynamic Programming
7.3 Bellman's Principle of Optimality
7.4 Dynamic Programming Algorithms
7.5 Dynamic Programming Approaches
7.6 Deciding Agent Problem
7.7 AGA with Reinforcement Learning
7.7.1 Introduction
7.7.2 RL Algorithms
7.7.3 Modeling the Automatic Generation of Algorithms as a DAP
7.8 Summary
8.Conclusions and Future Trends
8.1 Introduction
8.2 Future Directions and Research Paths
8.3 Closing Thoughts
1.1 Introduction
1.2 Combinatorial Optimization
1.3 NP-hardness
1.4 NP-hardness in Combinatorial Optimization
1.5 General Framework of a Combinatorial Optimization Problem
1.6 Methods for Combinatorial Optimization Problems
1.7 A General Optimization Algorithm
1.8 Summary
2. The Master Problem
2.1 Introduction
2.2 The Problem Statement
2.3 The Space of Instances
2.4 The Space of Algorithms
2.5 The Space of Parameters
2.6 Simultaneous Parameter Optimization with AGA
2.7 Independent Parameter Optimization
2.8 The Algorithm Selection Problem
2.9 The No-Free-Lunch in AGA
2.10 Summary
3. Modeling Problems
3.1 The Modeling Process
3.2 Identifying Problems
3.3 Approaching Practical Problems in Operations Research
3.4 Fundamental Models in OR
3.5 Approaching Practical Problems via AI
3.6 Fundamental Models in AI
3.7 Approaching Practical Problems by AGA
3.8 A Fundamental AGA Model
3.9 Summary
4. AGA with Genetic Programming
4.1 Introduction
4.2 The Master Problem in AGA
4.3 Genetic Programming
4.3.1 The General Algorithmic Evolutionary Process
4.3.2 Genetic Operations in GP
4.4 GP as a Metaheuristic
4.5 Modeling the Master Problem with GP
4.5.1 Solution Representation as a Tree
4.5.2 The Fitness Function
4.6 The Evolution of Algorithms
4.7 Constructive and Refinement Algorithms
4.8 Robustness Versus Specialization
4.9 AGA for Population-Based Algorithms
4.10 Rediscovering Algorithms
4.11 AGA Specification Sheet
4.12 Summary
5. AGA and Machine Learning
5.1 Introduction
5.2 Schematic Overview of Machine Learning
5.2.1 Modeling a Practical Problem
5.2.2 Meaning of the Dataset
5.2.3 Hypothetical Model
5.2.4 The Optimization Problem
5.2.5 Algorithms for the Optimization Problem
5.3 Types of Problems in Machine Learning
5.4 Schematic Overview of the Automatic Generation of Algorithms
5.4.1 Problem Instances
5.4.2 Algorithmic Components
5.4.3 The Master Problem
5.4.4 The Resulting Algorithm
5.5 Symbolic Regression
5.6 Summary
6. Producing Metaheuristics Automatically
6.1 Metaheuristics and AGA
6.2 Types of Metaheuristics
6.3 Key Concepts in Metaheuristics
6.4 Solution Container Definition
6.5 Terminals Definition
6.6 Defining Terminals for AGM
6.7 Possible Combinations
6.8 Summary
7. AGA with Reinforcement Learning
7.1 Introduction
7.2 Dynamic Programming
7.3 Bellman's Principle of Optimality
7.4 Dynamic Programming Algorithms
7.5 Dynamic Programming Approaches
7.6 Deciding Agent Problem
7.7 AGA with Reinforcement Learning
7.7.1 Introduction
7.7.2 RL Algorithms
7.7.3 Modeling the Automatic Generation of Algorithms as a DAP
7.8 Summary
8.Conclusions and Future Trends
8.1 Introduction
8.2 Future Directions and Research Paths
8.3 Closing Thoughts