Alle Infos zum eBook verschenken
- Format: PDF
- Merkliste
- Auf die Merkliste
- Bewerten Bewerten
- Teilen
- Produkt teilen
- Produkterinnerung
- Produkterinnerung
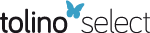
Hier können Sie sich einloggen
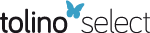
Bitte loggen Sie sich zunächst in Ihr Kundenkonto ein oder registrieren Sie sich bei bücher.de, um das eBook-Abo tolino select nutzen zu können.
Batch Effects and Noise in Microarray Experiments: Sources and Solutions looks at the issue of technical noise and batch effects in microarray studies and illustrates how to alleviate such factors whilst interpreting the relevant biological information. Each chapter focuses on sources of noise and batch effects before starting an experiment, with examples of statistical methods for detecting, measuring, and managing batch effects within and across datasets provided online. Throughout the book the importance of standardization and the value of standard operating procedures in the development of…mehr
- Geräte: PC
- mit Kopierschutz
- eBook Hilfe
- Größe: 4.95MB
- Geoffrey MclachlanAnalyzing Microarray Gene Expression Data (eBook, PDF)146,99 €
- Dhammika AmaratungaExploration and Analysis of DNA Microarray and Other High-Dimensional Data (eBook, PDF)112,99 €
- Michael StumpfHandbook of Statistical Systems Biology (eBook, PDF)158,99 €
- Handbook of Statistical Genetics (eBook, PDF)314,99 €
- Bendix CarstensenComparing Clinical Measurement Methods (eBook, PDF)74,99 €
- Shelemyahu ZacksStage-Wise Adaptive Designs (eBook, PDF)131,99 €
- Gerald Van BelleDesign and Analysis of Experiments in the Health Sciences (eBook, PDF)56,99 €
-
-
-
Dieser Download kann aus rechtlichen Gründen nur mit Rechnungsadresse in A, B, BG, CY, CZ, D, DK, EW, E, FIN, F, GR, HR, H, IRL, I, LT, L, LR, M, NL, PL, P, R, S, SLO, SK ausgeliefert werden.
- Produktdetails
- Verlag: John Wiley & Sons
- Seitenzahl: 272
- Erscheinungstermin: 13. Oktober 2009
- Englisch
- ISBN-13: 9780470685990
- Artikelnr.: 37298822
- Verlag: John Wiley & Sons
- Seitenzahl: 272
- Erscheinungstermin: 13. Oktober 2009
- Englisch
- ISBN-13: 9780470685990
- Artikelnr.: 37298822
- Herstellerkennzeichnung Die Herstellerinformationen sind derzeit nicht verfügbar.
Foreword xvii
Preface xix
1 Variation, Variability, Batches and Bias in Microarray Experiments: An
Introduction 1
Andreas Scherer
2 Microarray Platforms and Aspects of Experimental Variation 5
John A Coller Jr
2.1 Introduction 5
2.2 Microarray Platforms 6
2.2.1 Affymetrix 6
2.2.2 Agilent 7
2.2.3 Illumina 7
2.2.4 Nimblegen 8
2.2.5 Spotted Microarrays 8
2.3 Experimental Considerations 9
2.3.1 Experimental Design 9
2.3.2 Sample and RNA Extraction 9
2.3.3 Amplification 12
2.3.4 Labeling 13
2.3.5 Hybridization 13
2.3.6 Washing 14
2.3.7 Scanning 15
2.3.8 Image Analysis and Data Extraction 16
2.3.9 Clinical Diagnosis 17
2.3.10 Interpretation of the Data 17
2.4 Conclusions 17
3 Experimental Design 19
Peter Grass
3.1 Introduction 19
3.2 Principles of Experimental Design 20
3.2.1 Definitions 20
3.2.2 Technical Variation 21
3.2.3 Biological Variation 21
3.2.4 Systematic Variation 22
3.2.5 Population, Random Sample, Experimental and Observational Units 22
3.2.6 Experimental Factors 22
3.2.7 Statistical Errors 23
3.3 Measures to Increase Precision and Accuracy 24
3.3.1 Randomization 25
3.3.2 Blocking 25
3.3.3 Replication 25
3.3.4 Further Measures to Optimize Study Design 26
3.4 Systematic Errors in Microarray Studies 28
3.4.1 Selection Bias 28
3.4.2 Observational Bias 28
3.4.3 Bias at Specimen/Tissue Collection 29
3.4.4 Bias at mRNA Extraction and Hybridization 30
3.5 Conclusion 30
4 Batches and Blocks, Sample Pools and Subsamples in the Design and
Analysis of Gene Expression Studies 33
Naomi Altman
4.1 Introduction 33
4.1.1 Batch Effects 35
4.2 A Statistical Linear Mixed Effects Model for Microarray Experiments 35
4.2.1 Using the Linear Model for Design 37
4.2.2 Examples of Design Guided by the Linear Model 37
4.3 Blocks and Batches 39
4.3.1 Complete Block Designs 39
4.3.2 Incomplete Block Designs 39
4.3.3 Multiple Batch Effects 40
4.4 Reducing Batch Effects by Normalization and Statistical Adjustment 41
4.4.1 Between and Within Batch Normalization with Multi-array Methods 43
4.4.2 Statistical Adjustment 46
4.5 Sample Pooling and Sample Splitting 47
4.5.1 Sample Pooling 47
4.5.2 Sample Splitting: Technical Replicates 48
4.6 Pilot Experiments 49
4.7 Conclusions 49
Acknowledgements 50
5 Aspects of Technical Bias 51
Martin Schumacher, Frank Staedtler, Wendell D Jones, and Andreas Scherer
5.1 Introduction 51
5.2 Observational Studies 52
5.2.1 Same Protocol, Different Times of Processing 52
5.2.2 Same Protocol, Different Sites (Study 1) 53
5.2.3 Same Protocol, Different Sites (Study 2) 55
5.2.4 Batch Effect Characteristics at the Probe Level 57
5.3 Conclusion 60
6 Bioinformatic Strategies for cDNA-Microarray Data Processing 61
Jessica Fahlén, Mattias Landfors, Eva Freyhult, Max Bylesjö, Johan Trygg,
Torgeir R Hvidsten, and Patrik Rydén
6.1 Introduction 61
6.1.1 Spike-in Experiments 62
6.1.2 Key Measures - Sensitivity and Bias 63
6.1.3 The IC Curve and MA Plot 63
6.2 Pre-processing 64
6.2.1 Scanning Procedures 65
6.2.2 Background Correction 65
6.2.3 Saturation 67
6.2.4 Normalization 68
6.2.5 Filtering 70
6.3 Downstream Analysis 71
6.3.1 Gene Selection 71
6.3.2 Cluster Analysis 71
6.4 Conclusion 73
7 Batch Effect Estimation of Microarray Platforms with Analysis of Variance
75
Nysia I George and James J Chen
7.1 Introduction 75
7.1.1 Microarray Gene Expression Data 76
7.1.2 Analysis of Variance in Gene Expression Data 77
7.2 Variance Component Analysis across Microarray Platforms 78
7.3 Methodology 78
7.3.1 Data Description 78
7.3.2 Normalization 79
7.3.3 Gene-Specific ANOVA Model 81
7.4 Application: The MAQC Project 81
7.5 Discussion and Conclusion 85
Acknowledgements 85
8 Variance due to Smooth Bias in Rat Liver and Kidney Baseline Gene
Expression in a Large Multi-laboratory Data Set 87
Michael J Boedigheimer, Jeff W Chou, J Christopher Corton, Jennifer Fostel,
Raegan O'Lone, P Scott Pine, John Quackenbush, Karol L Thompson, and
Russell D Wolfinger
8.1 Introduction 87
8.2 Methodology 89
8.3 Results 89
8.3.1 Assessment of Smooth Bias in Baseline Expression Data Sets 89
8.3.2 Relationship between Smooth Bias and Signal Detection 91
8.3.3 Effect of Smooth Bias Correction on Principal Components Analysis 92
8.3.4 Effect of Smooth Bias Correction on Estimates of Attributable
Variability 94
8.3.5 Effect of Smooth Bias Correction on Detection of Genes Differentially
Expressed by Fasting 95
8.3.6 Effect of Smooth Bias Correction on the Detection of Strain-Selective
Gene Expression 96
8.4 Discussion 97
Acknowledgements 99
9 Microarray Gene Expression: The Effects of Varying Certain Measurement
Conditions 101
Walter Liggett, Jean Lozach, Anne Bergstrom Lucas, Ron L Peterson, Marc L
Salit, Danielle Thierry-Mieg, Jean Thierry-Mieg, and Russell D Wolfinger
9.1 Introduction 101
9.2 Input Mass Effect on the Amount of Normalization Applied 103
9.3 Probe-by-Probe Modeling of the Input Mass Effect 103
9.4 Further Evidence of Batch Effects 108
9.5 Conclusions 110
10 Adjusting Batch Effects in Microarray Experiments with Small Sample Size
Using Empirical Bayes Methods 113
W Evan Johnson and Cheng li
10.1 Introduction 113
10.1.1 Bayesian and Empirical Bayes Applications in Microarrays 114
10.2 Existing Methods for Adjusting Batch Effect 115
10.2.1 Microarray Data Normalization 115
10.2.2 Batch Effect Adjustment Methods for Large Sample Size 115
10.2.3 Model-Based Location and Scale Adjustments 116
10.3 Empirical Bayes Method for Adjusting Batch Effect 117
10.3.1 Parametric Shrinkage Adjustment 117
10.3.2 Empirical Bayes Batch Effect Parameter Estimates using Nonparametric
Empirical Priors 120
10.4 Data Examples, Results and Robustness of the Empirical Bayes Method
121
10.4.1 Microarray Data with Batch Effects 121
10.4.2 Results for Data Set 1 124
10.4.3 Results for Data Set 2 124
10.4.4 Robustness of the Empirical Bayes Method 126
10.4.5 Software Implementation 127
10.5 Discussion 128
11 Identical Reference Samples and Empirical Bayes Method for Cross-Batch
Gene Expression Analysis 131
Wynn L Walker and Frank R Sharp
11.1 Introduction 131
11.2 Methodology 133
11.2.1 Data Description 133
11.2.2 Empirical Bayes Method for Batch Adjustment 134
11.2.3 Naïve t-test Batch Adjustment 135
11.3 Application: Expression Profiling of Blood from Muscular Dystrophy
Patients 135
11.3.1 Removal of Cross-Experimental Batch Effects 135
11.3.2 Removal of Within-Experimental Batch Effects 136
11.3.3 Removal of Batch Effects: Empirical Bayes Method versus t-Test
Filter 137
11.4 Discussion and Conclusion 138
11.4.1 Methods for Batch Adjustment Within and Across Experiments 138
11.4.2 Bayesian Approach is Well Suited for Modeling Cross-Experimental
Batch Effects 139
11.4.3 Implications of Cross-Experimental Batch Corrections for Clinical
Studies 139
12 Principal Variance Components Analysis: Estimating Batch Effects in
Microarray Gene Expression Data 141
Jianying Li, Pierre R Bushel, Tzu-Ming Chu, and Russell D Wolfinger
12.1 Introduction 141
12.2 Methods 143
12.2.1 Principal Components Analysis 143
12.2.2 Variance Components Analysis and Mixed Models 145
12.2.3 Principal Variance Components Analysis 145
12.3 Experimental Data 146
12.3.1 A Transcription Inhibition Study 146
12.3.2 A Lung Cancer Toxicity Study 147
12.3.3 A Hepato-toxicant Toxicity Study 147
12.4 Application of the PVCA Procedure to the Three Example Data Sets 148
12.4.1 PVCA Provides Detailed Estimates of Batch Effects 148
12.4.2 Visualizing the Sources of Batch Effects 149
12.4.3 Selecting the Principal Components in the Modeling 150
12.5 Discussion 153
13 Batch Profile Estimation, Correction, and Scoring 155
Tzu-Ming Chu, Wenjun Bao, Russell S Thomas, and Russell D Wolfinger
13.1 Introduction 155
13.2 Mouse Lung Tumorigenicity Data Set with Batch Effects 157
13.2.1 Batch Profile Estimation 159
13.2.2 Batch Profile Correction 160
13.2.3 Batch Profile Scoring 161
13.2.4 Cross-Validation Results 162
13.3 Discussion 164
Acknowledgements 165
14 Visualization of Cross-Platform Microarray Normalization 167
Xuxin Liu, Joel Parker, Cheng Fan, Charles M Perou, and J S Marron
14.1 Introduction 167
14.2 Analysis of the NCI 60 Data 169
14.3 Improved Statistical Power 174
14.4 Gene-by-Gene versus Multivariate Views 178
14.5 Conclusion 181
15 Toward Integration of Biological Noise: Aggregation Effect in Microarray
Data Analysis 183
Lev Klebanov and Andreas Scherer
15.1 Introduction 183
15.2 Aggregated Expression Intensities 185
15.3 Covariance between Log-Expressions 186
15.4 Conclusion 189
Acknowledgements 190
16 Potential Sources of Spurious Associations and Batch Effects in
Genome-Wide Association Studies 191
Huixiao Hong, Leming Shi, James C Fuscoe, Federico Goodsaid, Donna
Mendrick, and Weida Tong
16.1 Introduction 191
16.2 Potential Sources of Spurious Associations 192
16.2.1 Spurious Associations Related to Study Design 194
16.2.2 Spurious Associations Caused in Genotyping Experiments 195
16.2.3 Spurious Associations Caused by Genotype Calling Errors 195
16.3 Batch Effects 196
16.3.1 Batch Effect in Genotyping Experiment 196
16.3.2 Batch Effect in Genotype Calling 197
16.4 Conclusion 201
Disclaimer 201
17 Standard Operating Procedures in Clinical Gene Expression Biomarker
Panel Development 203
Khurram Shahzad, Anshu Sinha, Farhana Latif, and Mario C Deng
17.1 Introduction 203
17.2 Theoretical Framework 204
17.3 Systems-Biological Concepts in Medicine 204
17.4 General Conceptual Challenges 205
17.5 Strategies for Gene Expression Biomarker Development 205
17.5.1 Phase 1: Clinical Phenotype Consensus Definition 206
17.5.2 Phase 2: Gene Discovery 207
17.5.3 Phase 3: Internal Differential Gene List Confirmation 209
17.5.4 Phase 4: Diagnostic Classifier Development 209
17.5.5 Phase 5: External Clinical Validation 210
17.5.6 Phase 6: Clinical Implementation 211
17.5.7 Phase 7: Post-Clinical Implementation Studies 212
17.6 Conclusions 213
18 Data, Analysis, and Standardization 215
Gabriella Rustici, Andreas Scherer, and John Quackenbush
18.1 Introduction 215
18.2 Reporting Standards 216
18.3 Computational Standards: From Microarray to Omic Sciences 219
18.3.1 The Microarray Gene Expression Data Society 219
18.3.2 The Proteomics Standards Initiative 220
18.3.3 The Metabolomics Standards Initiative 220
18.3.4 The Genomic Standards Consortium 220
18.3.5 Systems Biology Initiatives 221
18.3.6 Data Standards in Biopharmaceutical and Clinical Research 221
18.3.7 Standards Integration Initiatives 222
18.3.8 The MIBBI project 223
18.3.9 OBO Foundry 223
18.3.10 FuGE and ISA-TAB 223
18.4 Experimental Standards: Developing Quality Metrics and a Consensus on
Data Analysis Methods 226
18.5 Conclusions and Future Perspective 228
References 231
Index 245
Foreword xvii
Preface xix
1 Variation, Variability, Batches and Bias in Microarray Experiments: An
Introduction 1
Andreas Scherer
2 Microarray Platforms and Aspects of Experimental Variation 5
John A Coller Jr
2.1 Introduction 5
2.2 Microarray Platforms 6
2.2.1 Affymetrix 6
2.2.2 Agilent 7
2.2.3 Illumina 7
2.2.4 Nimblegen 8
2.2.5 Spotted Microarrays 8
2.3 Experimental Considerations 9
2.3.1 Experimental Design 9
2.3.2 Sample and RNA Extraction 9
2.3.3 Amplification 12
2.3.4 Labeling 13
2.3.5 Hybridization 13
2.3.6 Washing 14
2.3.7 Scanning 15
2.3.8 Image Analysis and Data Extraction 16
2.3.9 Clinical Diagnosis 17
2.3.10 Interpretation of the Data 17
2.4 Conclusions 17
3 Experimental Design 19
Peter Grass
3.1 Introduction 19
3.2 Principles of Experimental Design 20
3.2.1 Definitions 20
3.2.2 Technical Variation 21
3.2.3 Biological Variation 21
3.2.4 Systematic Variation 22
3.2.5 Population, Random Sample, Experimental and Observational Units 22
3.2.6 Experimental Factors 22
3.2.7 Statistical Errors 23
3.3 Measures to Increase Precision and Accuracy 24
3.3.1 Randomization 25
3.3.2 Blocking 25
3.3.3 Replication 25
3.3.4 Further Measures to Optimize Study Design 26
3.4 Systematic Errors in Microarray Studies 28
3.4.1 Selection Bias 28
3.4.2 Observational Bias 28
3.4.3 Bias at Specimen/Tissue Collection 29
3.4.4 Bias at mRNA Extraction and Hybridization 30
3.5 Conclusion 30
4 Batches and Blocks, Sample Pools and Subsamples in the Design and
Analysis of Gene Expression Studies 33
Naomi Altman
4.1 Introduction 33
4.1.1 Batch Effects 35
4.2 A Statistical Linear Mixed Effects Model for Microarray Experiments 35
4.2.1 Using the Linear Model for Design 37
4.2.2 Examples of Design Guided by the Linear Model 37
4.3 Blocks and Batches 39
4.3.1 Complete Block Designs 39
4.3.2 Incomplete Block Designs 39
4.3.3 Multiple Batch Effects 40
4.4 Reducing Batch Effects by Normalization and Statistical Adjustment 41
4.4.1 Between and Within Batch Normalization with Multi-array Methods 43
4.4.2 Statistical Adjustment 46
4.5 Sample Pooling and Sample Splitting 47
4.5.1 Sample Pooling 47
4.5.2 Sample Splitting: Technical Replicates 48
4.6 Pilot Experiments 49
4.7 Conclusions 49
Acknowledgements 50
5 Aspects of Technical Bias 51
Martin Schumacher, Frank Staedtler, Wendell D Jones, and Andreas Scherer
5.1 Introduction 51
5.2 Observational Studies 52
5.2.1 Same Protocol, Different Times of Processing 52
5.2.2 Same Protocol, Different Sites (Study 1) 53
5.2.3 Same Protocol, Different Sites (Study 2) 55
5.2.4 Batch Effect Characteristics at the Probe Level 57
5.3 Conclusion 60
6 Bioinformatic Strategies for cDNA-Microarray Data Processing 61
Jessica Fahlén, Mattias Landfors, Eva Freyhult, Max Bylesjö, Johan Trygg,
Torgeir R Hvidsten, and Patrik Rydén
6.1 Introduction 61
6.1.1 Spike-in Experiments 62
6.1.2 Key Measures - Sensitivity and Bias 63
6.1.3 The IC Curve and MA Plot 63
6.2 Pre-processing 64
6.2.1 Scanning Procedures 65
6.2.2 Background Correction 65
6.2.3 Saturation 67
6.2.4 Normalization 68
6.2.5 Filtering 70
6.3 Downstream Analysis 71
6.3.1 Gene Selection 71
6.3.2 Cluster Analysis 71
6.4 Conclusion 73
7 Batch Effect Estimation of Microarray Platforms with Analysis of Variance
75
Nysia I George and James J Chen
7.1 Introduction 75
7.1.1 Microarray Gene Expression Data 76
7.1.2 Analysis of Variance in Gene Expression Data 77
7.2 Variance Component Analysis across Microarray Platforms 78
7.3 Methodology 78
7.3.1 Data Description 78
7.3.2 Normalization 79
7.3.3 Gene-Specific ANOVA Model 81
7.4 Application: The MAQC Project 81
7.5 Discussion and Conclusion 85
Acknowledgements 85
8 Variance due to Smooth Bias in Rat Liver and Kidney Baseline Gene
Expression in a Large Multi-laboratory Data Set 87
Michael J Boedigheimer, Jeff W Chou, J Christopher Corton, Jennifer Fostel,
Raegan O'Lone, P Scott Pine, John Quackenbush, Karol L Thompson, and
Russell D Wolfinger
8.1 Introduction 87
8.2 Methodology 89
8.3 Results 89
8.3.1 Assessment of Smooth Bias in Baseline Expression Data Sets 89
8.3.2 Relationship between Smooth Bias and Signal Detection 91
8.3.3 Effect of Smooth Bias Correction on Principal Components Analysis 92
8.3.4 Effect of Smooth Bias Correction on Estimates of Attributable
Variability 94
8.3.5 Effect of Smooth Bias Correction on Detection of Genes Differentially
Expressed by Fasting 95
8.3.6 Effect of Smooth Bias Correction on the Detection of Strain-Selective
Gene Expression 96
8.4 Discussion 97
Acknowledgements 99
9 Microarray Gene Expression: The Effects of Varying Certain Measurement
Conditions 101
Walter Liggett, Jean Lozach, Anne Bergstrom Lucas, Ron L Peterson, Marc L
Salit, Danielle Thierry-Mieg, Jean Thierry-Mieg, and Russell D Wolfinger
9.1 Introduction 101
9.2 Input Mass Effect on the Amount of Normalization Applied 103
9.3 Probe-by-Probe Modeling of the Input Mass Effect 103
9.4 Further Evidence of Batch Effects 108
9.5 Conclusions 110
10 Adjusting Batch Effects in Microarray Experiments with Small Sample Size
Using Empirical Bayes Methods 113
W Evan Johnson and Cheng li
10.1 Introduction 113
10.1.1 Bayesian and Empirical Bayes Applications in Microarrays 114
10.2 Existing Methods for Adjusting Batch Effect 115
10.2.1 Microarray Data Normalization 115
10.2.2 Batch Effect Adjustment Methods for Large Sample Size 115
10.2.3 Model-Based Location and Scale Adjustments 116
10.3 Empirical Bayes Method for Adjusting Batch Effect 117
10.3.1 Parametric Shrinkage Adjustment 117
10.3.2 Empirical Bayes Batch Effect Parameter Estimates using Nonparametric
Empirical Priors 120
10.4 Data Examples, Results and Robustness of the Empirical Bayes Method
121
10.4.1 Microarray Data with Batch Effects 121
10.4.2 Results for Data Set 1 124
10.4.3 Results for Data Set 2 124
10.4.4 Robustness of the Empirical Bayes Method 126
10.4.5 Software Implementation 127
10.5 Discussion 128
11 Identical Reference Samples and Empirical Bayes Method for Cross-Batch
Gene Expression Analysis 131
Wynn L Walker and Frank R Sharp
11.1 Introduction 131
11.2 Methodology 133
11.2.1 Data Description 133
11.2.2 Empirical Bayes Method for Batch Adjustment 134
11.2.3 Naïve t-test Batch Adjustment 135
11.3 Application: Expression Profiling of Blood from Muscular Dystrophy
Patients 135
11.3.1 Removal of Cross-Experimental Batch Effects 135
11.3.2 Removal of Within-Experimental Batch Effects 136
11.3.3 Removal of Batch Effects: Empirical Bayes Method versus t-Test
Filter 137
11.4 Discussion and Conclusion 138
11.4.1 Methods for Batch Adjustment Within and Across Experiments 138
11.4.2 Bayesian Approach is Well Suited for Modeling Cross-Experimental
Batch Effects 139
11.4.3 Implications of Cross-Experimental Batch Corrections for Clinical
Studies 139
12 Principal Variance Components Analysis: Estimating Batch Effects in
Microarray Gene Expression Data 141
Jianying Li, Pierre R Bushel, Tzu-Ming Chu, and Russell D Wolfinger
12.1 Introduction 141
12.2 Methods 143
12.2.1 Principal Components Analysis 143
12.2.2 Variance Components Analysis and Mixed Models 145
12.2.3 Principal Variance Components Analysis 145
12.3 Experimental Data 146
12.3.1 A Transcription Inhibition Study 146
12.3.2 A Lung Cancer Toxicity Study 147
12.3.3 A Hepato-toxicant Toxicity Study 147
12.4 Application of the PVCA Procedure to the Three Example Data Sets 148
12.4.1 PVCA Provides Detailed Estimates of Batch Effects 148
12.4.2 Visualizing the Sources of Batch Effects 149
12.4.3 Selecting the Principal Components in the Modeling 150
12.5 Discussion 153
13 Batch Profile Estimation, Correction, and Scoring 155
Tzu-Ming Chu, Wenjun Bao, Russell S Thomas, and Russell D Wolfinger
13.1 Introduction 155
13.2 Mouse Lung Tumorigenicity Data Set with Batch Effects 157
13.2.1 Batch Profile Estimation 159
13.2.2 Batch Profile Correction 160
13.2.3 Batch Profile Scoring 161
13.2.4 Cross-Validation Results 162
13.3 Discussion 164
Acknowledgements 165
14 Visualization of Cross-Platform Microarray Normalization 167
Xuxin Liu, Joel Parker, Cheng Fan, Charles M Perou, and J S Marron
14.1 Introduction 167
14.2 Analysis of the NCI 60 Data 169
14.3 Improved Statistical Power 174
14.4 Gene-by-Gene versus Multivariate Views 178
14.5 Conclusion 181
15 Toward Integration of Biological Noise: Aggregation Effect in Microarray
Data Analysis 183
Lev Klebanov and Andreas Scherer
15.1 Introduction 183
15.2 Aggregated Expression Intensities 185
15.3 Covariance between Log-Expressions 186
15.4 Conclusion 189
Acknowledgements 190
16 Potential Sources of Spurious Associations and Batch Effects in
Genome-Wide Association Studies 191
Huixiao Hong, Leming Shi, James C Fuscoe, Federico Goodsaid, Donna
Mendrick, and Weida Tong
16.1 Introduction 191
16.2 Potential Sources of Spurious Associations 192
16.2.1 Spurious Associations Related to Study Design 194
16.2.2 Spurious Associations Caused in Genotyping Experiments 195
16.2.3 Spurious Associations Caused by Genotype Calling Errors 195
16.3 Batch Effects 196
16.3.1 Batch Effect in Genotyping Experiment 196
16.3.2 Batch Effect in Genotype Calling 197
16.4 Conclusion 201
Disclaimer 201
17 Standard Operating Procedures in Clinical Gene Expression Biomarker
Panel Development 203
Khurram Shahzad, Anshu Sinha, Farhana Latif, and Mario C Deng
17.1 Introduction 203
17.2 Theoretical Framework 204
17.3 Systems-Biological Concepts in Medicine 204
17.4 General Conceptual Challenges 205
17.5 Strategies for Gene Expression Biomarker Development 205
17.5.1 Phase 1: Clinical Phenotype Consensus Definition 206
17.5.2 Phase 2: Gene Discovery 207
17.5.3 Phase 3: Internal Differential Gene List Confirmation 209
17.5.4 Phase 4: Diagnostic Classifier Development 209
17.5.5 Phase 5: External Clinical Validation 210
17.5.6 Phase 6: Clinical Implementation 211
17.5.7 Phase 7: Post-Clinical Implementation Studies 212
17.6 Conclusions 213
18 Data, Analysis, and Standardization 215
Gabriella Rustici, Andreas Scherer, and John Quackenbush
18.1 Introduction 215
18.2 Reporting Standards 216
18.3 Computational Standards: From Microarray to Omic Sciences 219
18.3.1 The Microarray Gene Expression Data Society 219
18.3.2 The Proteomics Standards Initiative 220
18.3.3 The Metabolomics Standards Initiative 220
18.3.4 The Genomic Standards Consortium 220
18.3.5 Systems Biology Initiatives 221
18.3.6 Data Standards in Biopharmaceutical and Clinical Research 221
18.3.7 Standards Integration Initiatives 222
18.3.8 The MIBBI project 223
18.3.9 OBO Foundry 223
18.3.10 FuGE and ISA-TAB 223
18.4 Experimental Standards: Developing Quality Metrics and a Consensus on
Data Analysis Methods 226
18.5 Conclusions and Future Perspective 228
References 231
Index 245