Building a Platform for Data-Driven Pandemic Prediction (eBook, PDF)
From Data Modelling to Visualisation - The CovidLP Project
Redaktion: Gamerman, Dani; Mayrink, Vinicius D.; Paiva, Thais; Prates, Marcos O.
62,95 €
62,95 €
inkl. MwSt.
Sofort per Download lieferbar
31 °P sammeln
62,95 €
Als Download kaufen
62,95 €
inkl. MwSt.
Sofort per Download lieferbar
31 °P sammeln
Jetzt verschenken
Alle Infos zum eBook verschenken
62,95 €
inkl. MwSt.
Sofort per Download lieferbar
Alle Infos zum eBook verschenken
31 °P sammeln
Building a Platform for Data-Driven Pandemic Prediction (eBook, PDF)
From Data Modelling to Visualisation - The CovidLP Project
Redaktion: Gamerman, Dani; Mayrink, Vinicius D.; Paiva, Thais; Prates, Marcos O.
- Format: PDF
- Merkliste
- Auf die Merkliste
- Bewerten Bewerten
- Teilen
- Produkt teilen
- Produkterinnerung
- Produkterinnerung
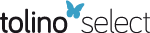
Bitte loggen Sie sich zunächst in Ihr Kundenkonto ein oder registrieren Sie sich bei
bücher.de, um das eBook-Abo tolino select nutzen zu können.
Hier können Sie sich einloggen
Hier können Sie sich einloggen
Sie sind bereits eingeloggt. Klicken Sie auf 2. tolino select Abo, um fortzufahren.
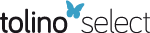
Bitte loggen Sie sich zunächst in Ihr Kundenkonto ein oder registrieren Sie sich bei bücher.de, um das eBook-Abo tolino select nutzen zu können.
This book is about building platforms for pandemic prediction. It provides an overview of probabilistic prediction for pandemic modeling based on a data-driven approach. It also provides guidance on building platforms with currently available technology using tools such as R, Shiny, and interactive plotting programs.
- Geräte: PC
- ohne Kopierschutz
- eBook Hilfe
- Größe: 7.22MB
Andere Kunden interessierten sich auch für
- Building a Platform for Data-Driven Pandemic Prediction (eBook, ePUB)62,95 €
- Anders AhlbomBiostatistics for Epidemiologists (eBook, PDF)241,95 €
- Mikel AickinCausal Analysis in Biomedicine and Epidemiology (eBook, PDF)66,95 €
- Thomas A. GerdsMedical Risk Prediction Models (eBook, PDF)52,95 €
- Textbook of Clinical Trials in Oncology (eBook, PDF)52,95 €
- Craig MallinckrodtAnalyzing Longitudinal Clinical Trial Data (eBook, PDF)48,95 €
- Brian K. NunnallySix Sigma in the Pharmaceutical Industry (eBook, PDF)126,95 €
-
-
-
This book is about building platforms for pandemic prediction. It provides an overview of probabilistic prediction for pandemic modeling based on a data-driven approach. It also provides guidance on building platforms with currently available technology using tools such as R, Shiny, and interactive plotting programs.
Dieser Download kann aus rechtlichen Gründen nur mit Rechnungsadresse in A, B, BG, CY, CZ, D, DK, EW, E, FIN, F, GR, HR, H, IRL, I, LT, L, LR, M, NL, PL, P, R, S, SLO, SK ausgeliefert werden.
Produktdetails
- Produktdetails
- Verlag: Taylor & Francis eBooks
- Seitenzahl: 382
- Erscheinungstermin: 13. September 2021
- Englisch
- ISBN-13: 9781000457193
- Artikelnr.: 62463222
- Verlag: Taylor & Francis eBooks
- Seitenzahl: 382
- Erscheinungstermin: 13. September 2021
- Englisch
- ISBN-13: 9781000457193
- Artikelnr.: 62463222
- Herstellerkennzeichnung Die Herstellerinformationen sind derzeit nicht verfügbar.
Dani Gamerman was Professor of Statistics at UFRJ from 1996 to 2019. He is currently Professor Emeritus at UFRJ since 2021 and Visiting Professor at UFMG since 2019. Director of the Graduate Program in Statistics at UFRJ (1999-2006 and 2015-2019). Published papers at JRSSB, Biometrika, Statistics and Computing, Bayesian Analysis, Journal of Multivariate Analysis, Applied Statistics and other journals. Author of books Monte Carlo Markov Chain: Stochastic Simulation for Bayesian Inference and Statistical Inference: an Integrated Approach, both with Chapman & Hall in their 2nd edition. Supervised 19 M.Sc. and 18 Ph.D. students. Visiting professor of a number of academic institution world-wide. Delivered seminars at many scientific meetings and universities world-wide, including plenary talks at a Valencia meeting and an ISBA world meeting. Editor of 5 statistical journals. Organized many statistical conferences in Brazil. Marcos Prates obtained his bachelor's in 2006 in the Computational Mathematics program at the Universidade Federal de Minas Gerais (UFMG) and a master's in Statistics in 2008 from the same institution. In 2011 he received his Ph.D. in Statistics from the University of Connecticut and was a Visiting Professor in the same institution from 2019 to 2020. Currently, he is an Associate Professor at UFMG. His main research areas are Bayesian Statistics, Generalised Linear Mixed Models, Machine Learning, and Spatial Statistics. He was Director of the Graduate Program in Statistics in UFMG (2016-2018), was the Secretary for ISBRA, the Brazilian chapter of ISBA (2015-2016), and currently is the President of the Brazilian Statistical Association (2020-2022). Thais Paiva obtained a bachelor degree in Actuarial Science from the Universidade Federal de Minas Gerais (UFMG) in 2008, and a Masters in Statistics from the same university in 2010. She earned a PhD degree in Statistics at Duke University in 2014. Since 2016, she has been an Assistant Professor in the Statistics Department at UFMG, working actively on the Actuarial Science undergraduate program and on the Statistics graduate program. Her main research interests are Bayesian Statistics, Imputation Methods for Missing Data, Data Confidentiality and Spatial Statistics. Vinicius Mayrink is an Associate Professor in the Department of Statistics at the Universidade Federal de Minas Gerais (UFMG) in Brazil. He received: his Ph.D. degree in the Department of Statistical Science at Duke University (USA, 2011), B.Sc. degree in Statistics from UFMG (2004), M.Sc. degree in Statistics from the Universidade Federal do Rio de Janeiro (2006) and a second M.Sc. degree in Statistics at Duke University (2009). Vinicius is currently the sub-Director of the Graduate Program in Statistics of the UFMG (2021-2023). He was a member (treasurer, 2015-2016) of the administrative board of ISBRA (the Brazilian chapter of ISBA). His research interests include: Bayesian Inference, Multivariate Analysis, Spatial Statistics, Survival Analysis and Statistical Modeling in Bioinformatics.
I Introduction
1. Overview of the book
2. Pandemic Data
II Modelling
3. Basic Epidemiological Features
4. Data Distributions
5. Modelling Specific Data Features
6. Review of Bayesian Inference
III Further Modelling
7. Modelling Misreported Data
8. Hierarchical Modelling
IV Implementation
9. Data Extraction/ETL
10. Automating Modelling and Inference
11. Building an Interactive App with Shiny
V Monitoring
12. Daily Evaluation of the Updated Data
13. Investigating Inference Results
14. Comparing Predictions
VI Software
15. PandemicLP Package: Basic Functionalities
16. Advanced Settings: The Pandemic Model Funtion
VII Conclusion
17. Future Directions
1. Overview of the book
2. Pandemic Data
II Modelling
3. Basic Epidemiological Features
4. Data Distributions
5. Modelling Specific Data Features
6. Review of Bayesian Inference
III Further Modelling
7. Modelling Misreported Data
8. Hierarchical Modelling
IV Implementation
9. Data Extraction/ETL
10. Automating Modelling and Inference
11. Building an Interactive App with Shiny
V Monitoring
12. Daily Evaluation of the Updated Data
13. Investigating Inference Results
14. Comparing Predictions
VI Software
15. PandemicLP Package: Basic Functionalities
16. Advanced Settings: The Pandemic Model Funtion
VII Conclusion
17. Future Directions
I Introduction
1. Overview of the book
2. Pandemic Data
II Modelling
3. Basic Epidemiological Features
4. Data Distributions
5. Modelling Specific Data Features
6. Review of Bayesian Inference
III Further Modelling
7. Modelling Misreported Data
8. Hierarchical Modelling
IV Implementation
9. Data Extraction/ETL
10. Automating Modelling and Inference
11. Building an Interactive App with Shiny
V Monitoring
12. Daily Evaluation of the Updated Data
13. Investigating Inference Results
14. Comparing Predictions
VI Software
15. PandemicLP Package: Basic Functionalities
16. Advanced Settings: The Pandemic Model Funtion
VII Conclusion
17. Future Directions
1. Overview of the book
2. Pandemic Data
II Modelling
3. Basic Epidemiological Features
4. Data Distributions
5. Modelling Specific Data Features
6. Review of Bayesian Inference
III Further Modelling
7. Modelling Misreported Data
8. Hierarchical Modelling
IV Implementation
9. Data Extraction/ETL
10. Automating Modelling and Inference
11. Building an Interactive App with Shiny
V Monitoring
12. Daily Evaluation of the Updated Data
13. Investigating Inference Results
14. Comparing Predictions
VI Software
15. PandemicLP Package: Basic Functionalities
16. Advanced Settings: The Pandemic Model Funtion
VII Conclusion
17. Future Directions