Case Studies in Bayesian Statistical Modelling and Analysis (eBook, ePUB)
Redaktion: Alston, Clair L.; Pettitt, Anthony N.; Mengersen, Kerrie
Alle Infos zum eBook verschenken
Case Studies in Bayesian Statistical Modelling and Analysis (eBook, ePUB)
Redaktion: Alston, Clair L.; Pettitt, Anthony N.; Mengersen, Kerrie
- Format: ePub
- Merkliste
- Auf die Merkliste
- Bewerten Bewerten
- Teilen
- Produkt teilen
- Produkterinnerung
- Produkterinnerung
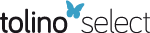
Hier können Sie sich einloggen
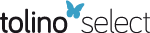
Bitte loggen Sie sich zunächst in Ihr Kundenkonto ein oder registrieren Sie sich bei bücher.de, um das eBook-Abo tolino select nutzen zu können.
Provides an accessible foundation to Bayesian analysis using real world models This book aims to present an introduction to Bayesian modelling and computation, by considering real case studies drawn from diverse fields spanning ecology, health, genetics and finance. Each chapter comprises a description of the problem, the corresponding model, the computational method, results and inferences as well as the issues that arise in the implementation of these approaches. Case Studies in Bayesian Statistical Modelling and Analysis: * Illustrates how to do Bayesian analysis in a clear and concise…mehr
- Geräte: eReader
- mit Kopierschutz
- eBook Hilfe
- Größe: 6.32MB
- Case Studies in Bayesian Statistical Modelling and Analysis (eBook, PDF)75,99 €
- Ioannis NtzoufrasBayesian Modeling Using WinBUGS (eBook, ePUB)142,99 €
- Sik-Yum LeeBasic and Advanced Bayesian Structural Equation Modeling (eBook, ePUB)83,99 €
- Causality (eBook, ePUB)73,99 €
- Stephen Winters-HiltInformatics and Machine Learning (eBook, ePUB)109,99 €
- Charles E. MccullochGeneralized, Linear, and Mixed Models (eBook, ePUB)171,99 €
- Linda M. CollinsLatent Class and Latent Transition Analysis (eBook, ePUB)118,99 €
-
-
-
Dieser Download kann aus rechtlichen Gründen nur mit Rechnungsadresse in A, B, BG, CY, CZ, D, DK, EW, E, FIN, F, GR, HR, H, IRL, I, LT, L, LR, M, NL, PL, P, R, S, SLO, SK ausgeliefert werden.
- Produktdetails
- Verlag: John Wiley & Sons
- Seitenzahl: 504
- Erscheinungstermin: 12. Oktober 2012
- Englisch
- ISBN-13: 9781118394328
- Artikelnr.: 37351308
- Verlag: John Wiley & Sons
- Seitenzahl: 504
- Erscheinungstermin: 12. Oktober 2012
- Englisch
- ISBN-13: 9781118394328
- Artikelnr.: 37351308
- Herstellerkennzeichnung Die Herstellerinformationen sind derzeit nicht verfügbar.
257 15.4.3 Sampling ß 1:n 257 15.4.4 Sampling
259 15.4.5 Likelihood calculation 260 15.5 Analysis 261 15.5.1 Prior elicitation 261 15.5.2 Estimation output 261 15.6 Conclusion 264 References 265 16 Bayesian mixture models: When the thing you need to know is the thing you cannot measure 267 Clair L. Alston, Kerrie L. Mengersen and Graham E. Gardner 16.1 Introduction 267 16.2 Case study: CT scan images of sheep 268 16.3 Models and methods 270 16.3.1 Bayesian mixture models 270 16.3.2 Parameter estimation using the Gibbs sampler 273 16.3.3 Extending the model to incorporate spatial information 274 16.4 Data analysis and results 276 16.4.1 Normal Bayesian mixture model 276 16.4.2 Spatial mixture model 278 16.4.3 Carcase volume calculation 281 16.5 Discussion 284 References 284 17 Latent class models in medicine 287 Margaret Rolfe, Nicole M. White and Carla Chen 17.1 Introduction 287 17.2 Case studies 288 17.2.1 Case study 1: Parkinson's disease 288 17.2.2 Case study 2: Cognition in breast cancer 288 17.3 Models and methods 289 17.3.1 Finite mixture models 290 17.3.2 Trajectory mixture models 292 17.3.3 Goodness of fit 296 17.3.4 Label switching 297 17.3.5 Model computation 298 17.4 Data analysis and results 300 17.4.1 Case study 1: Phenotype identification in PD 300 17.4.2 Case study 2: Trajectory groups for verbal memory 302 17.5 Discussion 306 References 307 18 Hidden Markov models for complex stochastic processes: A case study in electrophysiology 310 Nicole M. White, Helen Johnson, Peter Silburn, Judith Rousseau and Kerrie L. Mengersen 18.1 Introduction 310 18.2 Case study: Spike identification and sorting of extracellular recordings 311 18.3 Models and methods 312 18.3.1 What is an HMM? 312 18.3.2 Modelling a single AP: Application of a simple HMM 313 18.3.3 Multiple neurons: An application of a factorial HMM 315 18.3.4 Model estimation and inference 317 18.4 Data analysis and results 320 18.4.1 Simulation study 320 18.4.2 Case study: Extracellular recordings collected during deep brain stimulation 323 18.5 Discussion 326 References 327 19 Bayesian classification and regression trees 330 Rebecca A. O'Leary, Samantha Low Choy, Wenbiao Hu and Kerrie L. Mengersen 19.1 Introduction 330 19.2 Case studies 332 19.2.1 Case study 1: Kyphosis 332 19.2.2 Case study 2: Cryptosporidium 332 19.3 Models and methods 334 19.3.1 CARTs 334 19.3.2 Bayesian CARTs 335 19.4 Computation 337 19.4.1 Building the BCART model - stochastic search 337 19.4.2 Model diagnostics and identifying good trees 339 19.5 Case studies - results 341 19.5.1 Case study 1: Kyphosis 341 19.5.2 Case study 2: Cryptosporidium 343 19.6 Discussion 345 References 346 20 Tangled webs: Using Bayesian networks in the fight against infection 348 Mary Waterhouse and Sandra Johnson 20.1 Introduction to Bayesian network modelling 348 20.1.1 Building a BN 349 20.2 Introduction to case study 351 20.3 Model 352 20.4 Methods 354 20.5 Results 355 20.6 Discussion 357 References 359 21 Implementing adaptive dose finding studies using sequential Monte Carlo 361 James M. McGree, Christopher C. Drovandi and Anthony N. Pettitt 21.1 Introduction 361 21.2 Model and priors 363 21.3 SMC for dose finding studies 364 21.3.1 Importance sampling 364 21.3.2 SMC 365 21.3.3 Dose selection procedure 367 21.4 Example 369 21.5 Discussion 371 References 372 21.A Appendix: Extra example 373 22 Likelihood-free inference for transmission rates of nosocomial pathogens 374 Christopher C. Drovandi and Anthony N. Pettitt 22.1 Introduction 374 22.2 Case study: Estimating transmission rates of nosocomial pathogens 375 22.2.1 Background 375 22.2.2 Data 376 22.2.3 Objective 376 22.3 Models and methods 376 22.3.1 Models 376 22.3.2 Computing the likelihood 379 22.3.3 Model simulation 380 22.3.4 ABC 381 22.3.5 ABC algorithms 382 22.4 Data analysis and results 384 22.5 Discussion 385 References 386 23 Variational Bayesian inference for mixture models 388 Clare A. McGrory 23.1 Introduction 388 23.2 Case study: Computed tomography (CT) scanning of a loin portion of a pork carcase 390 23.3 Models and methods 392 23.4 Data analysis and results 397 23.5 Discussion 399 References 399 23.A Appendix: Form of the variational posterior for a mixture of multivariate normal densities 401 24 Issues in designing hybrid algorithms 403 Jeong E. Lee, Kerrie L. Mengersen and Christian P. Robert 24.1 Introduction 403 24.2 Algorithms and hybrid approaches 406 24.2.1 Particle system in the MCMC context 407 24.2.2 MALA 407 24.2.3 DRA 408 24.2.4 PS 409 24.2.5 Population Monte Carlo (PMC) algorithm 410 24.3 Illustration of hybrid algorithms 412 24.3.1 Simulated data set 412 24.3.2 Application: Aerosol particle size 415 24.4 Discussion 417 References 418 25 A Python package for Bayesian estimation using Markov chain Monte Carlo 421 Christopher M. Strickland, Robert J. Denham, Clair L. Alston and Kerrie L. Mengersen 25.1 Introduction 421 25.2 Bayesian analysis 423 25.2.1 MCMC methods and implementation 424 25.2.2 Normal linear Bayesian regression model 433 25.3 Empirical illustrations 437 25.3.1 Example 1: Linear regression model - variable selection and estimation 438 25.3.2 Example 2: Loglinear model 441 25.3.3 Example 3: First-order autoregressive regression 446 25.4 Using PyMCMC efficiently 451 25.4.1 Compiling code in Windows 455 25.5 PyMCMC interacting with R 457 25.6 Conclusions 458 25.7 Obtaining PyMCMC 459 References 459 Index 461
257 15.4.3 Sampling ß 1:n 257 15.4.4 Sampling
259 15.4.5 Likelihood calculation 260 15.5 Analysis 261 15.5.1 Prior elicitation 261 15.5.2 Estimation output 261 15.6 Conclusion 264 References 265 16 Bayesian mixture models: When the thing you need to know is the thing you cannot measure 267 Clair L. Alston, Kerrie L. Mengersen and Graham E. Gardner 16.1 Introduction 267 16.2 Case study: CT scan images of sheep 268 16.3 Models and methods 270 16.3.1 Bayesian mixture models 270 16.3.2 Parameter estimation using the Gibbs sampler 273 16.3.3 Extending the model to incorporate spatial information 274 16.4 Data analysis and results 276 16.4.1 Normal Bayesian mixture model 276 16.4.2 Spatial mixture model 278 16.4.3 Carcase volume calculation 281 16.5 Discussion 284 References 284 17 Latent class models in medicine 287 Margaret Rolfe, Nicole M. White and Carla Chen 17.1 Introduction 287 17.2 Case studies 288 17.2.1 Case study 1: Parkinson's disease 288 17.2.2 Case study 2: Cognition in breast cancer 288 17.3 Models and methods 289 17.3.1 Finite mixture models 290 17.3.2 Trajectory mixture models 292 17.3.3 Goodness of fit 296 17.3.4 Label switching 297 17.3.5 Model computation 298 17.4 Data analysis and results 300 17.4.1 Case study 1: Phenotype identification in PD 300 17.4.2 Case study 2: Trajectory groups for verbal memory 302 17.5 Discussion 306 References 307 18 Hidden Markov models for complex stochastic processes: A case study in electrophysiology 310 Nicole M. White, Helen Johnson, Peter Silburn, Judith Rousseau and Kerrie L. Mengersen 18.1 Introduction 310 18.2 Case study: Spike identification and sorting of extracellular recordings 311 18.3 Models and methods 312 18.3.1 What is an HMM? 312 18.3.2 Modelling a single AP: Application of a simple HMM 313 18.3.3 Multiple neurons: An application of a factorial HMM 315 18.3.4 Model estimation and inference 317 18.4 Data analysis and results 320 18.4.1 Simulation study 320 18.4.2 Case study: Extracellular recordings collected during deep brain stimulation 323 18.5 Discussion 326 References 327 19 Bayesian classification and regression trees 330 Rebecca A. O'Leary, Samantha Low Choy, Wenbiao Hu and Kerrie L. Mengersen 19.1 Introduction 330 19.2 Case studies 332 19.2.1 Case study 1: Kyphosis 332 19.2.2 Case study 2: Cryptosporidium 332 19.3 Models and methods 334 19.3.1 CARTs 334 19.3.2 Bayesian CARTs 335 19.4 Computation 337 19.4.1 Building the BCART model - stochastic search 337 19.4.2 Model diagnostics and identifying good trees 339 19.5 Case studies - results 341 19.5.1 Case study 1: Kyphosis 341 19.5.2 Case study 2: Cryptosporidium 343 19.6 Discussion 345 References 346 20 Tangled webs: Using Bayesian networks in the fight against infection 348 Mary Waterhouse and Sandra Johnson 20.1 Introduction to Bayesian network modelling 348 20.1.1 Building a BN 349 20.2 Introduction to case study 351 20.3 Model 352 20.4 Methods 354 20.5 Results 355 20.6 Discussion 357 References 359 21 Implementing adaptive dose finding studies using sequential Monte Carlo 361 James M. McGree, Christopher C. Drovandi and Anthony N. Pettitt 21.1 Introduction 361 21.2 Model and priors 363 21.3 SMC for dose finding studies 364 21.3.1 Importance sampling 364 21.3.2 SMC 365 21.3.3 Dose selection procedure 367 21.4 Example 369 21.5 Discussion 371 References 372 21.A Appendix: Extra example 373 22 Likelihood-free inference for transmission rates of nosocomial pathogens 374 Christopher C. Drovandi and Anthony N. Pettitt 22.1 Introduction 374 22.2 Case study: Estimating transmission rates of nosocomial pathogens 375 22.2.1 Background 375 22.2.2 Data 376 22.2.3 Objective 376 22.3 Models and methods 376 22.3.1 Models 376 22.3.2 Computing the likelihood 379 22.3.3 Model simulation 380 22.3.4 ABC 381 22.3.5 ABC algorithms 382 22.4 Data analysis and results 384 22.5 Discussion 385 References 386 23 Variational Bayesian inference for mixture models 388 Clare A. McGrory 23.1 Introduction 388 23.2 Case study: Computed tomography (CT) scanning of a loin portion of a pork carcase 390 23.3 Models and methods 392 23.4 Data analysis and results 397 23.5 Discussion 399 References 399 23.A Appendix: Form of the variational posterior for a mixture of multivariate normal densities 401 24 Issues in designing hybrid algorithms 403 Jeong E. Lee, Kerrie L. Mengersen and Christian P. Robert 24.1 Introduction 403 24.2 Algorithms and hybrid approaches 406 24.2.1 Particle system in the MCMC context 407 24.2.2 MALA 407 24.2.3 DRA 408 24.2.4 PS 409 24.2.5 Population Monte Carlo (PMC) algorithm 410 24.3 Illustration of hybrid algorithms 412 24.3.1 Simulated data set 412 24.3.2 Application: Aerosol particle size 415 24.4 Discussion 417 References 418 25 A Python package for Bayesian estimation using Markov chain Monte Carlo 421 Christopher M. Strickland, Robert J. Denham, Clair L. Alston and Kerrie L. Mengersen 25.1 Introduction 421 25.2 Bayesian analysis 423 25.2.1 MCMC methods and implementation 424 25.2.2 Normal linear Bayesian regression model 433 25.3 Empirical illustrations 437 25.3.1 Example 1: Linear regression model - variable selection and estimation 438 25.3.2 Example 2: Loglinear model 441 25.3.3 Example 3: First-order autoregressive regression 446 25.4 Using PyMCMC efficiently 451 25.4.1 Compiling code in Windows 455 25.5 PyMCMC interacting with R 457 25.6 Conclusions 458 25.7 Obtaining PyMCMC 459 References 459 Index 461