Chemometrics for Pattern Recognition (eBook, PDF)
Alle Infos zum eBook verschenken
Chemometrics for Pattern Recognition (eBook, PDF)
- Format: PDF
- Merkliste
- Auf die Merkliste
- Bewerten Bewerten
- Teilen
- Produkt teilen
- Produkterinnerung
- Produkterinnerung
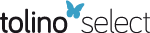
Hier können Sie sich einloggen
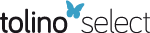
Bitte loggen Sie sich zunächst in Ihr Kundenkonto ein oder registrieren Sie sich bei bücher.de, um das eBook-Abo tolino select nutzen zu können.
Over the past decade, pattern recognition has been one of the fastest growth points in chemometrics. This has been catalysed by the increase in capabilities of automated instruments such as LCMS, GCMS, and NMR, to name a few, to obtain large quantities of data, and, in parallel, the significant growth in applications especially in biomedical analytical chemical measurements of extracts from humans and animals, together with the increased capabilities of desktop computing. The interpretation of such multivariate datasets has required the application and development of new chemometric techniques…mehr
- Geräte: PC
- mit Kopierschutz
- eBook Hilfe
- Größe: 37.25MB
- Chemometric Methods in Capillary Electrophoresis (eBook, PDF)123,99 €
- David N. LivingstoneA Practical Guide to Scientific Data Analysis (eBook, PDF)69,99 €
- Richard G. BreretonChemometrics (eBook, PDF)83,99 €
- Gil AlterovitzAutomation in Proteomics and Genomics (eBook, PDF)125,99 €
- Tibor CserhatiMultivariate Methods in Chromatography (eBook, PDF)152,99 €
- Alexey L. PomerantsevChemometrics in Excel (eBook, PDF)81,99 €
- Foo-Tim ChauChemometrics (eBook, PDF)155,99 €
-
-
-
Dieser Download kann aus rechtlichen Gründen nur mit Rechnungsadresse in A, B, BG, CY, CZ, D, DK, EW, E, FIN, F, GR, HR, H, IRL, I, LT, L, LR, M, NL, PL, P, R, S, SLO, SK ausgeliefert werden.
- Produktdetails
- Verlag: Jossey-Bass
- Seitenzahl: 522
- Erscheinungstermin: 1. Juli 2009
- Englisch
- ISBN-13: 9780470746479
- Artikelnr.: 37299040
- Verlag: Jossey-Bass
- Seitenzahl: 522
- Erscheinungstermin: 1. Juli 2009
- Englisch
- ISBN-13: 9780470746479
- Artikelnr.: 37299040
- Herstellerkennzeichnung Die Herstellerinformationen sind derzeit nicht verfügbar.
Preface xv
1 Introduction 1
1.1 Past, Present and Future 1
1.2 About this Book 9
Bibliography 12
2 Case Studies 15
2.1 Introduction 15
2.2 Datasets, Matrices and Vectors 17
2.3 Case Study 1: Forensic Analysis of Banknotes 20
2.4 Case Study 2: Near Infrared Spectroscopic Analysis of Food 23
2.5 Case Study 3: Thermal Analysis of Polymers 25
2.6 Case Study 4: Environmental Pollution using Headspace Mass Spectrometry
27
2.7 Case Study 5: Human Sweat Analysed by Gas Chromatography Mass
Spectrometry 30
2.8 Case Study 6: Liquid Chromatography Mass Spectrometry of Pharmaceutical
Tablets 32
2.9 Case Study 7: Atomic Spectroscopy for the Study of Hypertension 34
2.10 Case Study 8: Metabolic Profiling of Mouse Urine by Gas Chromatography
of Urine Extracts 36
2.11 Case Study 9: Nuclear Magnetic Resonance Spectroscopy for Salival
Analysis of the Effect of Mouthwash 37
2.12 Case Study 10: Simulations 38
2.13 Case Study 11: Null Dataset 40
2.14 Case Study 12: GCMS and Microbiology of Mouse Scent Marks 42
Bibliography 45
3 Exploratory Data Analysis 47
3.1 Introduction 47
3.2 Principal Components Analysis 49
3.2.1 Background 49
3.2.2 Scores and Loadings 50
3.2.3 Eigenvalues 53
3.2.4 PCA Algorithm 57
3.2.5 Graphical Representation 57
3.3 Dissimilarity Indices, Principal Co-ordinates Analysis and Ranking 75
3.3.1 Dissimilarity 75
3.3.2 Principal Co-ordinates Analysis 80
3.3.3 Ranking 84
3.4 Self Organizing Maps 87
3.4.1 Background 87
3.4.2 SOM Algorithm 88
3.4.3 Initialization 89
3.4.4 Training 90
3.4.5 Map Quality 93
3.4.6 Visualization 95
Bibliography 105
4 Preprocessing 107
4.1 Introduction 107
4.2 Data Scaling 108
4.2.1 Transforming Individual Elements 108
4.2.2 Row Scaling 117
4.2.3 Column Scaling 124
4.3 Multivariate Methods of Data Reduction 129
4.3.1 Largest Principal Components 129
4.3.2 Discriminatory Principal Components 137
4.3.3 Partial Least Squares Discriminatory Analysis Scores 145
4.4 Strategies for Data Preprocessing 150
4.4.1 Flow Charts 150
4.4.2 Level 1 153
4.4.3 Level 2 161
4.4.4 Level 3 162
4.4.5 Level 4 175
Bibliography 176
5 Two Class Classifiers 177
5.1 Introduction 177
5.1.1 Two Class Classifiers 178
5.1.2 Preprocessing 180
5.1.3 Notation 180
5.1.4 Autoprediction and Class Boundaries 181
5.2 Euclidean Distance to Centroids 184
5.3 Linear Discriminant Analysis 185
5.4 Quadratic Discriminant Analysis 192
5.5 Partial Least Squares Discriminant Analysis 196
5.5.1 PLS Method 196
5.5.2 PLS Algorithm 198
5.5.3 PLS-da 199
5.6 Learning Vector Quantization 201
5.6.1 Voronoi Tesselation and Codebooks 206
5.6.2 LVQ 1 207
5.6.3 LVQ 3 209
5.6.4 LVQ Illustration and Summary of Parameters 211
5.7 Support Vector Machines 213
5.7.1 Linear Learning Machines 214
5.7.2 Kernels 218
5.7.3 Controlling Complexity and Soft Margin SVMs 223
5.7.4 SVM Parameters 228
Bibliography 231
6 One Class Classifiers 233
6.1 Introduction 233
6.2 Distance Based Classifiers 235
6.3 PC Based Models and SIMCA 236
6.4 Indicators of Significance 239
6.4.1 Gaussian Density Estimators and Chi-Squared 239
6.4.2 Hotelling's T2241
6.4.3 D-Statistic 243
6.4.4 Q-Statistic or Squared Prediction Error 248
6.4.5 Visualization of D- and Q-Statistics for Disjoint PC Models 249
6.4.6 Multivariate Normality and What to do if it Fails 263
6.5 Support Vector Data Description 266
6.6 Summarizing One Class Classifiers 275
6.6.1 Class Membership Plots 275
6.6.2 ROC Curves 279
Bibliography 286
7 Multiclass Classifiers 289
7.1 Introduction 289
7.2 EDC, LDA and QDA 291
7.3 LVQ 295
7.4 PLS 298
7.4.1 PLS 2 298
7.4.2 PLS 1 300
7.5 SVM 304
7.6 One against One Decisions 304
Bibliography 309
8 Validation and Optimization 311
8.1 Introduction 311
8.1.1 Validation 311
8.1.2 Optimization 315
8.2 Classification Abilities, Contingency Tables and Related Concepts 315
8.2.1 Two Class Classifiers 315
8.2.2 Multiclass Classifiers 318
8.2.3 One Class Classifiers 318
8.3 Validation 320
8.3.1 Testing Models 320
8.3.2 Test and Training Sets 321
8.3.3 Predictions 324
8.3.4 Increasing the Number of Variables for the Classifier 331
8.4 Iterative Approaches for Validation 335
8.4.1 Predictive Ability, Model Stability, Classification by Majority Vote
and Cross Classification Rate 335
8.4.2 Number of Iterations 348
8.4.3 Test and Training Set Boundaries 352
8.5 Optimizing PLS Models 361
8.5.1 Number of Components: Cross-Validation and Bootstrap 361
8.5.2 Thresholds and ROC Curves 374
8.6 Optimizing Learning Vector Quantization Models 377
8.7 Optimizing Support Vector Machine Models 380
Bibliography 390
9 Determining Potential Discriminatory Variables 393
9.1 Introduction 393
9.1.1 Two Class Distributions 394
9.1.2 Multiclass Distributions 395
9.1.3 Multilevel and Multiway Distributions 396
9.1.4 Sample Sizes 399
9.1.5 Modelling after Variable Reduction 401
9.1.6 Preliminary Variable Reduction 405
9.2 Which Variables are most Significant? 405
9.2.1 Basic Concepts: Statistical Indicators and Rank 405
9.2.2 T-Statistic and Fisher Weights 407
9.2.3 Multiple Linear Regression, ANOVA and the F-Ratio 417
9.2.4 Partial Least Squares 431
9.2.5 Relationship between the Indicator Functions 434
9.3 How Many Variables are Significant? 440
9.3.1 Probabilistic Approaches 440
9.3.2 Empirical Methods: Monte Carlo 442
9.3.3 Cost/Benefit of Increasing the Number of Variables 447
Bibliography 450
10 Bayesian Methods and Unequal Class Sizes 453
10.1 Introduction 453
10.2 Contingency Tables and Bayes' Theorem 453
10.3 Bayesian Extensions to Classifiers 458
Bibliography 467
11 Class Separation Indices 469
11.1 Introduction 469
11.2 Davies Bouldin Index 470
11.3 Silhouette Width and Modified Silhouette Width 475
11.3.1 Silhouette Width 475
11.3.2 Modified Silhouette Width 475
11.4 Overlap Coefficient 477
Bibliography 478
12 Comparing Different Patterns 479
12.1 Introduction 479
12.2 Correlation Based Methods 481
12.2.1 Mantel Test 481
12.2.2 R V Coefficient 483
12.3 Consensus PCA 484
12.4 Procrustes Analysis 487
Bibliography 492
Index 493
Preface xv
1 Introduction 1
1.1 Past, Present and Future 1
1.2 About this Book 9
Bibliography 12
2 Case Studies 15
2.1 Introduction 15
2.2 Datasets, Matrices and Vectors 17
2.3 Case Study 1: Forensic Analysis of Banknotes 20
2.4 Case Study 2: Near Infrared Spectroscopic Analysis of Food 23
2.5 Case Study 3: Thermal Analysis of Polymers 25
2.6 Case Study 4: Environmental Pollution using Headspace Mass Spectrometry
27
2.7 Case Study 5: Human Sweat Analysed by Gas Chromatography Mass
Spectrometry 30
2.8 Case Study 6: Liquid Chromatography Mass Spectrometry of Pharmaceutical
Tablets 32
2.9 Case Study 7: Atomic Spectroscopy for the Study of Hypertension 34
2.10 Case Study 8: Metabolic Profiling of Mouse Urine by Gas Chromatography
of Urine Extracts 36
2.11 Case Study 9: Nuclear Magnetic Resonance Spectroscopy for Salival
Analysis of the Effect of Mouthwash 37
2.12 Case Study 10: Simulations 38
2.13 Case Study 11: Null Dataset 40
2.14 Case Study 12: GCMS and Microbiology of Mouse Scent Marks 42
Bibliography 45
3 Exploratory Data Analysis 47
3.1 Introduction 47
3.2 Principal Components Analysis 49
3.2.1 Background 49
3.2.2 Scores and Loadings 50
3.2.3 Eigenvalues 53
3.2.4 PCA Algorithm 57
3.2.5 Graphical Representation 57
3.3 Dissimilarity Indices, Principal Co-ordinates Analysis and Ranking 75
3.3.1 Dissimilarity 75
3.3.2 Principal Co-ordinates Analysis 80
3.3.3 Ranking 84
3.4 Self Organizing Maps 87
3.4.1 Background 87
3.4.2 SOM Algorithm 88
3.4.3 Initialization 89
3.4.4 Training 90
3.4.5 Map Quality 93
3.4.6 Visualization 95
Bibliography 105
4 Preprocessing 107
4.1 Introduction 107
4.2 Data Scaling 108
4.2.1 Transforming Individual Elements 108
4.2.2 Row Scaling 117
4.2.3 Column Scaling 124
4.3 Multivariate Methods of Data Reduction 129
4.3.1 Largest Principal Components 129
4.3.2 Discriminatory Principal Components 137
4.3.3 Partial Least Squares Discriminatory Analysis Scores 145
4.4 Strategies for Data Preprocessing 150
4.4.1 Flow Charts 150
4.4.2 Level 1 153
4.4.3 Level 2 161
4.4.4 Level 3 162
4.4.5 Level 4 175
Bibliography 176
5 Two Class Classifiers 177
5.1 Introduction 177
5.1.1 Two Class Classifiers 178
5.1.2 Preprocessing 180
5.1.3 Notation 180
5.1.4 Autoprediction and Class Boundaries 181
5.2 Euclidean Distance to Centroids 184
5.3 Linear Discriminant Analysis 185
5.4 Quadratic Discriminant Analysis 192
5.5 Partial Least Squares Discriminant Analysis 196
5.5.1 PLS Method 196
5.5.2 PLS Algorithm 198
5.5.3 PLS-da 199
5.6 Learning Vector Quantization 201
5.6.1 Voronoi Tesselation and Codebooks 206
5.6.2 LVQ 1 207
5.6.3 LVQ 3 209
5.6.4 LVQ Illustration and Summary of Parameters 211
5.7 Support Vector Machines 213
5.7.1 Linear Learning Machines 214
5.7.2 Kernels 218
5.7.3 Controlling Complexity and Soft Margin SVMs 223
5.7.4 SVM Parameters 228
Bibliography 231
6 One Class Classifiers 233
6.1 Introduction 233
6.2 Distance Based Classifiers 235
6.3 PC Based Models and SIMCA 236
6.4 Indicators of Significance 239
6.4.1 Gaussian Density Estimators and Chi-Squared 239
6.4.2 Hotelling's T2241
6.4.3 D-Statistic 243
6.4.4 Q-Statistic or Squared Prediction Error 248
6.4.5 Visualization of D- and Q-Statistics for Disjoint PC Models 249
6.4.6 Multivariate Normality and What to do if it Fails 263
6.5 Support Vector Data Description 266
6.6 Summarizing One Class Classifiers 275
6.6.1 Class Membership Plots 275
6.6.2 ROC Curves 279
Bibliography 286
7 Multiclass Classifiers 289
7.1 Introduction 289
7.2 EDC, LDA and QDA 291
7.3 LVQ 295
7.4 PLS 298
7.4.1 PLS 2 298
7.4.2 PLS 1 300
7.5 SVM 304
7.6 One against One Decisions 304
Bibliography 309
8 Validation and Optimization 311
8.1 Introduction 311
8.1.1 Validation 311
8.1.2 Optimization 315
8.2 Classification Abilities, Contingency Tables and Related Concepts 315
8.2.1 Two Class Classifiers 315
8.2.2 Multiclass Classifiers 318
8.2.3 One Class Classifiers 318
8.3 Validation 320
8.3.1 Testing Models 320
8.3.2 Test and Training Sets 321
8.3.3 Predictions 324
8.3.4 Increasing the Number of Variables for the Classifier 331
8.4 Iterative Approaches for Validation 335
8.4.1 Predictive Ability, Model Stability, Classification by Majority Vote
and Cross Classification Rate 335
8.4.2 Number of Iterations 348
8.4.3 Test and Training Set Boundaries 352
8.5 Optimizing PLS Models 361
8.5.1 Number of Components: Cross-Validation and Bootstrap 361
8.5.2 Thresholds and ROC Curves 374
8.6 Optimizing Learning Vector Quantization Models 377
8.7 Optimizing Support Vector Machine Models 380
Bibliography 390
9 Determining Potential Discriminatory Variables 393
9.1 Introduction 393
9.1.1 Two Class Distributions 394
9.1.2 Multiclass Distributions 395
9.1.3 Multilevel and Multiway Distributions 396
9.1.4 Sample Sizes 399
9.1.5 Modelling after Variable Reduction 401
9.1.6 Preliminary Variable Reduction 405
9.2 Which Variables are most Significant? 405
9.2.1 Basic Concepts: Statistical Indicators and Rank 405
9.2.2 T-Statistic and Fisher Weights 407
9.2.3 Multiple Linear Regression, ANOVA and the F-Ratio 417
9.2.4 Partial Least Squares 431
9.2.5 Relationship between the Indicator Functions 434
9.3 How Many Variables are Significant? 440
9.3.1 Probabilistic Approaches 440
9.3.2 Empirical Methods: Monte Carlo 442
9.3.3 Cost/Benefit of Increasing the Number of Variables 447
Bibliography 450
10 Bayesian Methods and Unequal Class Sizes 453
10.1 Introduction 453
10.2 Contingency Tables and Bayes' Theorem 453
10.3 Bayesian Extensions to Classifiers 458
Bibliography 467
11 Class Separation Indices 469
11.1 Introduction 469
11.2 Davies Bouldin Index 470
11.3 Silhouette Width and Modified Silhouette Width 475
11.3.1 Silhouette Width 475
11.3.2 Modified Silhouette Width 475
11.4 Overlap Coefficient 477
Bibliography 478
12 Comparing Different Patterns 479
12.1 Introduction 479
12.2 Correlation Based Methods 481
12.2.1 Mantel Test 481
12.2.2 R V Coefficient 483
12.3 Consensus PCA 484
12.4 Procrustes Analysis 487
Bibliography 492
Index 493