Cognitive Radio Communication and Networking (eBook, ePUB)
Principles and Practice
Alle Infos zum eBook verschenken
Cognitive Radio Communication and Networking (eBook, ePUB)
Principles and Practice
- Format: ePub
- Merkliste
- Auf die Merkliste
- Bewerten Bewerten
- Teilen
- Produkt teilen
- Produkterinnerung
- Produkterinnerung
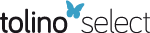
Hier können Sie sich einloggen
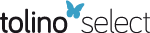
Bitte loggen Sie sich zunächst in Ihr Kundenkonto ein oder registrieren Sie sich bei bücher.de, um das eBook-Abo tolino select nutzen zu können.
The author presents a unified treatment of this highly interdisciplinary topic to help define the notion of cognitive radio. The book begins with addressing issues such as the fundamental system concept and basic mathematical tools such as spectrum sensing and machine learning, before moving on to more advanced concepts and discussions about the future of cognitive radio. From the fundamentals in spectrum sensing to the applications of cognitive algorithms to radio communications, and discussion of radio platforms and testbeds to show the applicability of the theory to practice, the author…mehr
- Geräte: eReader
- mit Kopierschutz
- eBook Hilfe
- Größe: 12.69MB
- Prabhat ThakurSpectrum Sharing in Cognitive Radio Networks (eBook, ePUB)106,99 €
- Dan ForsbergLTE Security (eBook, ePUB)95,99 €
- Jack L. BurbankWireless Networking (eBook, ePUB)127,99 €
- Benny BingBroadband Wireless Multimedia Networks (eBook, ePUB)98,99 €
- Seungjune YiRadio Protocols for LTE and LTE-Advanced (eBook, ePUB)111,99 €
- Mobile Ad Hoc Networking (eBook, ePUB)141,99 €
- G. Keith CambronGlobal Networks (eBook, ePUB)91,99 €
-
-
-
Dieser Download kann aus rechtlichen Gründen nur mit Rechnungsadresse in A, B, BG, CY, CZ, D, DK, EW, E, FIN, F, GR, HR, H, IRL, I, LT, L, LR, M, NL, PL, P, R, S, SLO, SK ausgeliefert werden.
- Produktdetails
- Verlag: John Wiley & Sons
- Seitenzahl: 536
- Erscheinungstermin: 10. September 2012
- Englisch
- ISBN-13: 9781118376294
- Artikelnr.: 37354568
- Verlag: John Wiley & Sons
- Seitenzahl: 536
- Erscheinungstermin: 10. September 2012
- Englisch
- ISBN-13: 9781118376294
- Artikelnr.: 37354568
- Herstellerkennzeichnung Die Herstellerinformationen sind derzeit nicht verfügbar.
B 105 4.5 Partial Ordering of Unitarily Invariant Norms: A < B 109 4.6 Partial Ordering of Positive Definite Matrices of Many Copies: K k=1 Ak
K k=1 Bk 109 4.7 Partial Ordering of Positive Operator Valued Random Variables: Prob(A
X
B) 110 4.8 Partial Ordering Using Stochastic Order: A
st B 115 4.9 Quantum Hypothesis Detection 115 4.10 Quantum Hypothesis Testing for Many Copies 118 5 Large Random Matrices 119 5.1 Large Dimensional Random Matrices: Moment Approach, Stieltjes Transform and Free Probability 119 5.2 Spectrum Sensing Using Large Random Matrices 121 5.2.1 System Model 121 5.2.2 Marchenko-Pastur Law 124 5.3 Moment Approach 129 5.3.1 Limiting Spectral Distribution 130 5.3.2 Limits of Extreme Eigenvalues 133 5.3.3 Convergence Rates of Spectral Distributions 136 5.3.4 Standard Vector-In, Vector-Out Model 137 5.3.5 Generalized Densities 138 5.4 Stieltjes Transform 139 5.4.1 Basic Theorems 143 5.4.2 Large Random Hankel, Markov and Toepltiz Matrices 149 5.4.3 Information Plus Noise Model of Random Matrices 152 5.4.4 Generalized Likelihood Ratio Test Using Large Random Matrices 157 5.4.5 Detection of High-Dimensional Signals in White Noise 164 5.4.6 Eigenvalues of (A + B)
1B and Applications 169 5.4.7 Canonical Correlation Analysis 171 5.4.8 Angles and Distances between Subspaces 173 5.4.9 Multivariate Linear Model 173 5.4.10 Equality of Covariance Matrices 174 5.4.11 Multiple Discriminant Analysis 174 5.5 Case Studies and Applications 175 5.5.1 Fundamental Example of Using Large Random Matrix 175 5.5.2 Stieltjes Transform 177 5.5.3 Free Deconvolution 178 5.5.4 Optimal Precoding of MIMO Systems 178 5.5.5 Marchenko and Pastur's Probability Distribution 179 5.5.6 Convergence and Fluctuations Extreme Eigenvalues 180 5.5.7 Information plus Noise Model and Spiked Models 180 5.5.8 Hypothesis Testing and Spectrum Sensing 183 5.5.9 Energy Estimation in a Wireless Network 185 5.5.10 Multisource Power Inference 187 5.5.11 Target Detection, Localization, and Reconstruction 187 5.5.12 State Estimation and Malignant Attacker in the Smart Grid 191 5.5.13 Covariance Matrix Estimation 193 5.5.14 Deterministic Equivalents 197 5.5.15 Local Failure Detection and Diagnosis 200 5.6 Regularized Estimation of Large Covariance Matrices 200 5.6.1 Regularized Covariance Estimates 201 5.6.2 Banding the Inverse 203 5.6.3 Covariance Regularization by Thresholding 204 5.6.4 Regularized Sample Covariance Matrices 206 5.6.5 Optimal Rates of Convergence for Covariance Matrix Estimation 208 5.6.6 Banding Sample Autocovariance Matrices of Stationary Processes 211 5.7 Free Probability 213 5.7.1 Large Random Matrices and Free Convolution 218 5.7.2 Vandermonde Matrices 221 5.7.3 Convolution and Deconvolution with Vandermonde Matrices 229 5.7.4 Finite Dimensional Statistical Inference 232 6 Convex Optimization 235 6.1 Linear Programming 237 6.2 Quadratic Programming 238 6.3 Semidefinite Programming 239 6.4 Geometric Programming 239 6.5 Lagrange Duality 241 6.6 Optimization Algorithm 242 6.6.1 Interior Point Methods 242 6.6.2 Stochastic Methods 243 6.7 Robust Optimization 244 6.8 Multiobjective Optimization 248 6.9 Optimization for Radio Resource Management 249 6.10 Examples and Applications 250 6.10.1 Spectral Efficiency for Multiple Input Multiple Output Ultra-Wideband Communication System 250 6.10.2 Wideband Waveform Design for Single Input Single Output Communication System with Noncoherent Receiver 256 6.10.3 Wideband Waveform Design for Multiple Input Single Output Cognitive Radio 262 6.10.4 Wideband Beamforming Design 268 6.10.5 Layering as Optimization Decomposition for Cognitive Radio Network 272 6.11 Summary 282 7 Machine Learning 283 7.1 Unsupervised Learning 288 7.1.1 Centroid-Based Clustering 288 7.1.2 k-Nearest Neighbors 289 7.1.3 Principal Component Analysis 289 7.1.4 Independent Component Analysis 290 7.1.5 Nonnegative Matrix Factorization 291 7.1.6 Self-Organizing Map 292 7.2 Supervised Learning 293 7.2.1 Linear Regression 293 7.2.2 Logistic Regression 294 7.2.3 Artificial Neural Network 294 7.2.4 Decision Tree Learning 294 7.2.5 Naive Bayes Classifier 295 7.2.6 Support Vector Machines 295 7.3 Semisupervised Learning 298 7.3.1 Constrained Clustering 298 7.3.2 Co-Training 298 7.3.3 Graph-Based Methods 299 7.4 Transductive Inference 299 7.5 Transfer Learning 299 7.6 Active Learning 299 7.7 Reinforcement Learning 300 7.7.1 Q-Learning 300 7.7.2 Markov Decision Process 301 7.7.3 Partially Observable MDPs 302 7.8 Kernel-Based Learning 303 7.9 Dimensionality Reduction 304 7.9.1 Kernel Principal Component Analysis 305 7.9.2 Multidimensional Scaling 307 7.9.3 Isomap 308 7.9.4 Locally-Linear Embedding 308 7.9.5 Laplacian Eigenmaps 309 7.9.6 Semidefinite Embedding 309 7.10 Ensemble Learning 311 7.11 Markov Chain Monte Carlo 312 7.12 Filtering Technique 313 7.12.1 Kalman Filtering 314 7.12.2 Particle Filtering 318 7.12.3 Collaborative Filtering 319 7.13 Bayesian Network 320 7.14 Summary 321 8 Agile Transmission Techniques (I): Multiple Input Multiple Output 323 8.1 Benefits of MIMO 323 8.1.1 Array Gain 323 8.1.2 Diversity Gain 323 8.1.3 Multiplexing Gain 324 8.2 Space Time Coding 324 8.2.1 Space Time Block Coding 325 8.2.2 Space Time Trellis Coding 326 8.2.3 Layered Space Time Coding 326 8.3 Multi-User MIMO 327 8.3.1 Space-Division Multiple Access 327 8.3.2 MIMO Broadcast Channel 328 8.3.3 MIMO Multiple Access Channel 330 8.3.4 MIMO Interference Channel 331 8.4 MIMO Network 334 8.5 MIMO Cognitive Radio Network 336 8.6 Summary 337 9 Agile Transmission Techniques (II): Orthogonal Frequency Division Multiplexing 339 9.1 OFDM Implementation 339 9.2 Synchronization 341 9.3 Channel Estimation 343 9.4 Peak Power Problem 345 9.5 Adaptive Transmission 345 9.6 Spectrum Shaping 347 9.7 Orthogonal Frequency Division Multiple Access 347 9.8 MIMO OFDM 349 9.9 OFDM Cognitive Radio Network 349 9.10 Summary 350 10 Game Theory 351 10.1 Basic Concepts of Games 351 10.1.1 Elements of Games 351 10.1.2 Nash Equilibrium: Definition and Existence 352 10.1.3 Nash Equilibrium: Computation 354 10.1.4 Nash Equilibrium: Zero-Sum Games 355 10.1.5 Nash Equilibrium: Bayesian Case 355 10.1.6 Nash Equilibrium: Stochastic Games 356 10.2 Primary User Emulation Attack Games 360 10.2.1 PUE Attack 360 10.2.2 Two-Player Case: A Strategic-Form Game 361 10.2.3 Game in Queuing Dynamics: A Stochastic Game 362 10.3 Games in Channel Synchronization 368 10.3.1 Background of the Game 368 10.3.2 System Model 368 10.3.3 Game Formulation 369 10.3.4 Bayesian Equilibrium 370 10.3.5 Numerical Results 371 10.4 Games in Collaborative Spectrum Sensing 372 10.4.1 False Report Attack 373 10.4.2 Game Formulation 373 10.4.3 Elements of Game 374 10.4.4 Bayesian Equilibrium 376 10.4.5 Numerical Results 379 11 Cognitive Radio Network 381 11.1 Basic Concepts of Networks 381 11.1.1 Network Architecture 381 11.1.2 Network Layers 382 11.1.3 Cross-Layer Design 384 11.1.4 Main Challenges in Cognitive Radio Networks 384 11.1.5 Complex Networks 385 11.2 Channel Allocation in MAC Layer 386 11.2.1 Problem Formulation 386 11.2.2 Scheduling Algorithm 387 11.2.3 Solution 389 11.2.4 Discussion 390 11.3 Scheduling in MAC Layer 391 11.3.1 Network Model 391 11.3.2 Goal of Scheduling 393 11.3.3 Scheduling Algorithm 393 11.3.4 Performance of the CNC Algorithm 395 11.3.5 Distributed Scheduling Algorithm 396 11.4 Routing in Network Layer 396 11.4.1 Challenges of Routing in Cognitive Radio 397 11.4.2 Stationary Routing 398 11.4.3 Dynamic Routing 402 11.5 Congestion Control in Transport Layer 404 11.5.1 Congestion Control in Internet 404 11.5.2 Challenges in Cognitive Radio 405 11.5.3 TP-CRAHN 406 11.5.4 Early Start Scheme 408 11.6 Complex Networks in Cognitive Radio 417 11.6.1 Brief Introduction to Complex Networks 418 11.6.2 Connectivity of Cognitive Radio Networks 421 11.6.3 Behavior Propagation in Cognitive Radio Networks 423 12 Cognitive Radio Network as Sensors 427 12.1 Intrusion Detection by Machine Learning 429 12.2 Joint Spectrum Sensing and Localization 429 12.3 Distributed Aspect Synthetic Aperture Radar 429 12.4 Wireless Tomography 433 12.5 Mobile Crowdsensing 434 12.6 Integration of 3S 435 12.7 The Cyber-Physical System 435 12.8 Computing 436 12.8.1 Graphics Processor Unit 437 12.8.2 Task Distribution and Load Balancing 437 12.9 Security and Privacy 438 12.10 Summary 438 Appendix A Matrix Analysis 441 A.1 Vector Spaces and Hilbert Space 441 A.2 Transformations 443 A.3 Trace 444 A.4 Basics of C
-Algebra 444 A.5 Noncommunicative Matrix-Valued Random Variables 445 A.6 Distances and Projections 447 A.6.1 Matrix Inequalities 450 A.6.2 Partial Ordering of Positive Semidefinite Matrices 451 A.6.3 Partial Ordering of Hermitian Matrices 451 References 453 Index 511
B 105 4.5 Partial Ordering of Unitarily Invariant Norms: A < B 109 4.6 Partial Ordering of Positive Definite Matrices of Many Copies: K k=1 Ak
K k=1 Bk 109 4.7 Partial Ordering of Positive Operator Valued Random Variables: Prob(A
X
B) 110 4.8 Partial Ordering Using Stochastic Order: A
st B 115 4.9 Quantum Hypothesis Detection 115 4.10 Quantum Hypothesis Testing for Many Copies 118 5 Large Random Matrices 119 5.1 Large Dimensional Random Matrices: Moment Approach, Stieltjes Transform and Free Probability 119 5.2 Spectrum Sensing Using Large Random Matrices 121 5.2.1 System Model 121 5.2.2 Marchenko-Pastur Law 124 5.3 Moment Approach 129 5.3.1 Limiting Spectral Distribution 130 5.3.2 Limits of Extreme Eigenvalues 133 5.3.3 Convergence Rates of Spectral Distributions 136 5.3.4 Standard Vector-In, Vector-Out Model 137 5.3.5 Generalized Densities 138 5.4 Stieltjes Transform 139 5.4.1 Basic Theorems 143 5.4.2 Large Random Hankel, Markov and Toepltiz Matrices 149 5.4.3 Information Plus Noise Model of Random Matrices 152 5.4.4 Generalized Likelihood Ratio Test Using Large Random Matrices 157 5.4.5 Detection of High-Dimensional Signals in White Noise 164 5.4.6 Eigenvalues of (A + B)
1B and Applications 169 5.4.7 Canonical Correlation Analysis 171 5.4.8 Angles and Distances between Subspaces 173 5.4.9 Multivariate Linear Model 173 5.4.10 Equality of Covariance Matrices 174 5.4.11 Multiple Discriminant Analysis 174 5.5 Case Studies and Applications 175 5.5.1 Fundamental Example of Using Large Random Matrix 175 5.5.2 Stieltjes Transform 177 5.5.3 Free Deconvolution 178 5.5.4 Optimal Precoding of MIMO Systems 178 5.5.5 Marchenko and Pastur's Probability Distribution 179 5.5.6 Convergence and Fluctuations Extreme Eigenvalues 180 5.5.7 Information plus Noise Model and Spiked Models 180 5.5.8 Hypothesis Testing and Spectrum Sensing 183 5.5.9 Energy Estimation in a Wireless Network 185 5.5.10 Multisource Power Inference 187 5.5.11 Target Detection, Localization, and Reconstruction 187 5.5.12 State Estimation and Malignant Attacker in the Smart Grid 191 5.5.13 Covariance Matrix Estimation 193 5.5.14 Deterministic Equivalents 197 5.5.15 Local Failure Detection and Diagnosis 200 5.6 Regularized Estimation of Large Covariance Matrices 200 5.6.1 Regularized Covariance Estimates 201 5.6.2 Banding the Inverse 203 5.6.3 Covariance Regularization by Thresholding 204 5.6.4 Regularized Sample Covariance Matrices 206 5.6.5 Optimal Rates of Convergence for Covariance Matrix Estimation 208 5.6.6 Banding Sample Autocovariance Matrices of Stationary Processes 211 5.7 Free Probability 213 5.7.1 Large Random Matrices and Free Convolution 218 5.7.2 Vandermonde Matrices 221 5.7.3 Convolution and Deconvolution with Vandermonde Matrices 229 5.7.4 Finite Dimensional Statistical Inference 232 6 Convex Optimization 235 6.1 Linear Programming 237 6.2 Quadratic Programming 238 6.3 Semidefinite Programming 239 6.4 Geometric Programming 239 6.5 Lagrange Duality 241 6.6 Optimization Algorithm 242 6.6.1 Interior Point Methods 242 6.6.2 Stochastic Methods 243 6.7 Robust Optimization 244 6.8 Multiobjective Optimization 248 6.9 Optimization for Radio Resource Management 249 6.10 Examples and Applications 250 6.10.1 Spectral Efficiency for Multiple Input Multiple Output Ultra-Wideband Communication System 250 6.10.2 Wideband Waveform Design for Single Input Single Output Communication System with Noncoherent Receiver 256 6.10.3 Wideband Waveform Design for Multiple Input Single Output Cognitive Radio 262 6.10.4 Wideband Beamforming Design 268 6.10.5 Layering as Optimization Decomposition for Cognitive Radio Network 272 6.11 Summary 282 7 Machine Learning 283 7.1 Unsupervised Learning 288 7.1.1 Centroid-Based Clustering 288 7.1.2 k-Nearest Neighbors 289 7.1.3 Principal Component Analysis 289 7.1.4 Independent Component Analysis 290 7.1.5 Nonnegative Matrix Factorization 291 7.1.6 Self-Organizing Map 292 7.2 Supervised Learning 293 7.2.1 Linear Regression 293 7.2.2 Logistic Regression 294 7.2.3 Artificial Neural Network 294 7.2.4 Decision Tree Learning 294 7.2.5 Naive Bayes Classifier 295 7.2.6 Support Vector Machines 295 7.3 Semisupervised Learning 298 7.3.1 Constrained Clustering 298 7.3.2 Co-Training 298 7.3.3 Graph-Based Methods 299 7.4 Transductive Inference 299 7.5 Transfer Learning 299 7.6 Active Learning 299 7.7 Reinforcement Learning 300 7.7.1 Q-Learning 300 7.7.2 Markov Decision Process 301 7.7.3 Partially Observable MDPs 302 7.8 Kernel-Based Learning 303 7.9 Dimensionality Reduction 304 7.9.1 Kernel Principal Component Analysis 305 7.9.2 Multidimensional Scaling 307 7.9.3 Isomap 308 7.9.4 Locally-Linear Embedding 308 7.9.5 Laplacian Eigenmaps 309 7.9.6 Semidefinite Embedding 309 7.10 Ensemble Learning 311 7.11 Markov Chain Monte Carlo 312 7.12 Filtering Technique 313 7.12.1 Kalman Filtering 314 7.12.2 Particle Filtering 318 7.12.3 Collaborative Filtering 319 7.13 Bayesian Network 320 7.14 Summary 321 8 Agile Transmission Techniques (I): Multiple Input Multiple Output 323 8.1 Benefits of MIMO 323 8.1.1 Array Gain 323 8.1.2 Diversity Gain 323 8.1.3 Multiplexing Gain 324 8.2 Space Time Coding 324 8.2.1 Space Time Block Coding 325 8.2.2 Space Time Trellis Coding 326 8.2.3 Layered Space Time Coding 326 8.3 Multi-User MIMO 327 8.3.1 Space-Division Multiple Access 327 8.3.2 MIMO Broadcast Channel 328 8.3.3 MIMO Multiple Access Channel 330 8.3.4 MIMO Interference Channel 331 8.4 MIMO Network 334 8.5 MIMO Cognitive Radio Network 336 8.6 Summary 337 9 Agile Transmission Techniques (II): Orthogonal Frequency Division Multiplexing 339 9.1 OFDM Implementation 339 9.2 Synchronization 341 9.3 Channel Estimation 343 9.4 Peak Power Problem 345 9.5 Adaptive Transmission 345 9.6 Spectrum Shaping 347 9.7 Orthogonal Frequency Division Multiple Access 347 9.8 MIMO OFDM 349 9.9 OFDM Cognitive Radio Network 349 9.10 Summary 350 10 Game Theory 351 10.1 Basic Concepts of Games 351 10.1.1 Elements of Games 351 10.1.2 Nash Equilibrium: Definition and Existence 352 10.1.3 Nash Equilibrium: Computation 354 10.1.4 Nash Equilibrium: Zero-Sum Games 355 10.1.5 Nash Equilibrium: Bayesian Case 355 10.1.6 Nash Equilibrium: Stochastic Games 356 10.2 Primary User Emulation Attack Games 360 10.2.1 PUE Attack 360 10.2.2 Two-Player Case: A Strategic-Form Game 361 10.2.3 Game in Queuing Dynamics: A Stochastic Game 362 10.3 Games in Channel Synchronization 368 10.3.1 Background of the Game 368 10.3.2 System Model 368 10.3.3 Game Formulation 369 10.3.4 Bayesian Equilibrium 370 10.3.5 Numerical Results 371 10.4 Games in Collaborative Spectrum Sensing 372 10.4.1 False Report Attack 373 10.4.2 Game Formulation 373 10.4.3 Elements of Game 374 10.4.4 Bayesian Equilibrium 376 10.4.5 Numerical Results 379 11 Cognitive Radio Network 381 11.1 Basic Concepts of Networks 381 11.1.1 Network Architecture 381 11.1.2 Network Layers 382 11.1.3 Cross-Layer Design 384 11.1.4 Main Challenges in Cognitive Radio Networks 384 11.1.5 Complex Networks 385 11.2 Channel Allocation in MAC Layer 386 11.2.1 Problem Formulation 386 11.2.2 Scheduling Algorithm 387 11.2.3 Solution 389 11.2.4 Discussion 390 11.3 Scheduling in MAC Layer 391 11.3.1 Network Model 391 11.3.2 Goal of Scheduling 393 11.3.3 Scheduling Algorithm 393 11.3.4 Performance of the CNC Algorithm 395 11.3.5 Distributed Scheduling Algorithm 396 11.4 Routing in Network Layer 396 11.4.1 Challenges of Routing in Cognitive Radio 397 11.4.2 Stationary Routing 398 11.4.3 Dynamic Routing 402 11.5 Congestion Control in Transport Layer 404 11.5.1 Congestion Control in Internet 404 11.5.2 Challenges in Cognitive Radio 405 11.5.3 TP-CRAHN 406 11.5.4 Early Start Scheme 408 11.6 Complex Networks in Cognitive Radio 417 11.6.1 Brief Introduction to Complex Networks 418 11.6.2 Connectivity of Cognitive Radio Networks 421 11.6.3 Behavior Propagation in Cognitive Radio Networks 423 12 Cognitive Radio Network as Sensors 427 12.1 Intrusion Detection by Machine Learning 429 12.2 Joint Spectrum Sensing and Localization 429 12.3 Distributed Aspect Synthetic Aperture Radar 429 12.4 Wireless Tomography 433 12.5 Mobile Crowdsensing 434 12.6 Integration of 3S 435 12.7 The Cyber-Physical System 435 12.8 Computing 436 12.8.1 Graphics Processor Unit 437 12.8.2 Task Distribution and Load Balancing 437 12.9 Security and Privacy 438 12.10 Summary 438 Appendix A Matrix Analysis 441 A.1 Vector Spaces and Hilbert Space 441 A.2 Transformations 443 A.3 Trace 444 A.4 Basics of C
-Algebra 444 A.5 Noncommunicative Matrix-Valued Random Variables 445 A.6 Distances and Projections 447 A.6.1 Matrix Inequalities 450 A.6.2 Partial Ordering of Positive Semidefinite Matrices 451 A.6.3 Partial Ordering of Hermitian Matrices 451 References 453 Index 511