Competing with High Quality Data (eBook, ePUB)
Concepts, Tools, and Techniques for Building a Successful Approach to Data Quality
Alle Infos zum eBook verschenken
Competing with High Quality Data (eBook, ePUB)
Concepts, Tools, and Techniques for Building a Successful Approach to Data Quality
- Format: ePub
- Merkliste
- Auf die Merkliste
- Bewerten Bewerten
- Teilen
- Produkt teilen
- Produkterinnerung
- Produkterinnerung
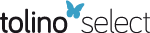
Hier können Sie sich einloggen
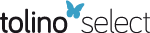
Bitte loggen Sie sich zunächst in Ihr Kundenkonto ein oder registrieren Sie sich bei bücher.de, um das eBook-Abo tolino select nutzen zu können.
Create a competitive advantage with data quality Data is rapidly becoming the powerhouse of industry, but low-quality data can actually put a company at a disadvantage. To be used effectively, data must accurately reflect the real-world scenario it represents, and it must be in a form that is usable and accessible. Quality data involves asking the right questions, targeting the correct parameters, and having an effective internal management, organization, and access system. It must be relevant, complete, and correct, while falling in line with pervasive regulatory oversight programs. Competing…mehr
- Geräte: eReader
- mit Kopierschutz
- eBook Hilfe
- Größe: 33.05MB
- Nicholas FisherThe Road to Quality Control (eBook, ePUB)100,99 €
- Rajesh JugulumCompeting with High Quality Data (eBook, PDF)83,99 €
- Kai YangQuality in the Era of Industry 4.0 (eBook, ePUB)73,99 €
- Peter W. G. MorrisThe Wiley Guide to Project Control (eBook, ePUB)68,99 €
- Souraj Salah77 Pillars of Quality and the Pursuit of Excellence (eBook, ePUB)43,95 €
- Ching M. ChangService Systems Management and Engineering (eBook, ePUB)127,99 €
- Ravi JainManaging Research, Development and Innovation (eBook, ePUB)131,99 €
-
-
-
Dieser Download kann aus rechtlichen Gründen nur mit Rechnungsadresse in A, B, BG, CY, CZ, D, DK, EW, E, FIN, F, GR, HR, H, IRL, I, LT, L, LR, M, NL, PL, P, R, S, SLO, SK ausgeliefert werden.
- Produktdetails
- Verlag: John Wiley & Sons
- Seitenzahl: 304
- Erscheinungstermin: 10. März 2014
- Englisch
- ISBN-13: 9781118416495
- Artikelnr.: 40614303
- Verlag: John Wiley & Sons
- Seitenzahl: 304
- Erscheinungstermin: 10. März 2014
- Englisch
- ISBN-13: 9781118416495
- Artikelnr.: 40614303
- Herstellerkennzeichnung Die Herstellerinformationen sind derzeit nicht verfügbar.
Prelude xv
Preface xvii
Acknowledgments xix
1 The Importance of Data Quality 1
1.0 Introduction 1
1.1 Understanding the Implications of Data Quality 1
1.2 The Data Management Function 4
1.3 The Solution Strategy 6
1.4 Guide to This Book 6
Section I Building a Data Quality Program 2 The Data Quality Operating
Model 13
2.0 Introduction 13
2.1 Data Quality Foundational Capabilities 13
2.1.1 Program Strategy and Governance 14
2.1.2 Skilled Data Quality Resources 14
2.1.3 Technology Infrastructure and Metadata 15
2.1.4 Data Profi ling and Analytics 15
2.1.5 Data Integration 15
2.1.6 Data Assessment 16
2.1.7 Issues Resolution (IR) 16
2.1.8 Data Quality Monitoring and Control 16
2.2 The Data Quality Methodology 17
2.2.1 Establish a Data Quality Program 17
2.2.2 Conduct a Current-State Analysis 17
2.2.3 Strengthen Data Quality Capability through Data Quality Projects 18
2.2.4 Monitor the Ongoing Production Environment and Measure Data Quality
Improvement Effectiveness 18
2.2.5 Detailed Discussion on Establishing the Data Quality Program 18
2.2.6 Assess the Current State of Data Quality 21
2.3 Conclusions 22
3 The DAIC Approach 23
3.0 Introduction 23
3.1 Six Sigma Methodologies 23
3.1.1 Development of Six Sigma Methodologies 25
3.2 DAIC Approach for Data Quality 28
3.2.1 The Defi ne Phase 28
3.2.2 The Assess Phase 31
3.2.3 The Improve Phase 36
3.2.4 The Control Phase (Monitor and Measure) 37
3.3 Conclusions 40
Section II Executing a Data Quality Program 4 Quantification of the Impact
of Data Quality 43
4.0 Introduction 43
4.1 Building a Data Quality Cost Quantifi cation Framework 43
4.1.1 The Cost Waterfall 44
4.1.2 Prioritization Matrix 46
4.1.3 Remediation and Return on Investment 50
4.2 A Trading Offi ce Illustrative Example 51
4.3 Conclusions 54
5 Statistical Process Control and Its Relevance in Data Quality Monitoring
and Reporting 55
5.0 Introduction 55
5.1 What Is Statistical Process Control? 55
5.1.1 Common Causes and Special Causes 57
5.2 Control Charts 59
5.2.1 Different Types of Data 59
5.2.2 Sample and Sample Parameters 60
5.2.3 Construction of Attribute Control Charts 62
5.2.4 Construction of Variable Control Charts 65
5.2.5 Other Control Charts 67
5.2.6 Multivariate Process Control Charts 69
5.3 Relevance of Statistical Process Control in Data Quality Monitoring and
Reporting 69
5.4 Conclusions 70
6 Critical Data Elements: Identification, Validation, and Assessment 71
6.0 Introduction 71
6.1 Identifi cation of Critical Data Elements 71
6.1.1 Data Elements and Critical Data Elements 71
6.1.2 CDE Rationalization Matrix 72
6.2 Assessment of Critical Data Elements 75
6.2.1 Data Quality Dimensions 76
6.2.2 Data Quality Business Rules 78
6.2.3 Data Profi ling 79
6.2.4 Measurement of Data Quality Scores 80
6.2.5 Results Recording and Reporting (Scorecard) 80
6.3 Conclusions 82
7 Prioritization of Critical Data Elements (Funnel Approach) 83
7.0 Introduction 83
7.1 The Funnel Methodology (Statistical Analysis for CDE Reduction) 83
7.1.1 Correlation and Regression Analysis for Continuous CDEs 85
7.1.2 Association Analysis for Discrete CDEs 88
7.1.3 Signal-to-Noise Ratios Analysis 90
7.2 Case Study: Basel II 91
7.2.1 Basel II: CDE Rationalization Matrix 91
7.2.2 Basel II: Correlation and Regression Analysis 94
7.2.3 Basel II: Signal-to-Noise (S/N) Ratios 96
7.3 Conclusions 99
8 Data Quality Monitoring and Reporting Scorecards 101
8.0 Introduction 101
8.1 Development of the DQ Scorecards 102
8.2 Analytical Framework (ANOVA, SPCs, Thresholds, Heat Maps) 102
8.2.1 Thresholds and Heat Maps 103
8.2.2 Analysis of Variance (ANOVA) and SPC Charts 107
8.3 Application of the Framework 109
8.4 Conclusions 112
9 Data Quality Issue Resolution 113
9.0 Introduction 113
9.1 Description of the Methodology 113
9.2 Data Quality Methodology 114
9.3 Process Quality/Six Sigma Approach 115
9.4 Case Study: Issue Resolution Process Reengineering 117
9.5 Conclusions 119
10 Information System Testing 121
10.0 Introduction 121
10.1 Typical System Arrangement 122
10.1.1 The Role of Orthogonal Arrays 123
10.2 Method of System Testing 123
10.2.1 Study of Two-Factor Combinations 123
10.2.2 Construction of Combination Tables 124
10.3 MTS Software Testing 126
10.4 Case Study: A Japanese Software Company 130
10.5 Case Study: A Finance Company 133
10.6 Conclusions 138
11 Statistical Approach for Data Tracing 139
11.0 Introduction 139
11.1 Data Tracing Methodology 139
11.1.1 Statistical Sampling 142
11.2 Case Study: Tracing 144
11.2.1 Analysis of Test Cases and CDE Prioritization 144
11.3 Data Lineage through Data Tracing 149
11.4 Conclusions 151
12 Design and Development of Multivariate Diagnostic Systems 153
12.0 Introduction 153
12.1 The Mahalanobis-Taguchi Strategy 153
12.1.1 The Gram Schmidt Orthogonalization Process 155
12.2 Stages in MTS 158
12.3 The Role of Orthogonal Arrays and Signal-to-Noise Ratio in
Multivariate Diagnosis 159
12.3.1 The Role of Orthogonal Arrays 159
12.3.2 The Role of S/N Ratios in MTS 161
12.3.3 Types of S/N Ratios 162
12.3.4 Direction of Abnormals 164
12.4 A Medical Diagnosis Example 172
12.5 Case Study: Improving Client Experience 175
12.5.1 Improvements Made Based on Recommendations from MTS Analysis 177
12.6 Case Study: Understanding the Behavior Patterns of Defaulting
Customers 178
12.7 Case Study: Marketing 180
12.7.1 Construction of the Reference Group 181
12.7.2 Validation of the Scale 181
12.7.3 Identification of Useful Variables 181
12.8 Case Study: Gear Motor Assembly 182
12.8.1 Apparatus 183
12.8.2 Sensors 184
12.8.3 High-Resolution Encoder 184
12.8.4 Life Test 185
12.8.5 Characterization 185
12.8.6 Construction of the Reference Group or Mahalanobis Space 186
12.8.7 Validation of the MTS Scale 187
12.8.8 Selection of Useful Variables 188
12.9 Conclusions 189
13 Data Analytics 191
13.0 Introduction 191
13.1 Data and Analytics as Key Resources 191
13.1.1 Different Types of Analytics 193
13.1.2 Requirements for Executing Analytics 195
13.1.3 Process of Executing Analytics 196
13.2 Data Innovation 197
13.2.1 Big Data 198
13.2.2 Big Data Analytics 199
13.2.3 Big Data Analytics Operating Model 206
13.2.4 Big Data Analytics Projects: Examples 207
13.3 Conclusions 208
14. Building a Data Quality Practices Center 209
14.0 Introduction 209
14.1 Building a DQPC 209
14.2 Conclusions 211
Appendix A 213
Equations for Signal-to-Noise (S/N) Ratios 213
Nondynamic S/N Ratios 213
Dynamic S/N Ratios 214
Appendix B 217
Matrix Theory: Related Topics 217
What Is a Matrix? 217
Appendix C 221
Some Useful Orthogonal Arrays 221
Two-Level Orthogonal Arrays 221
Three-Level Orthogonal Arrays 255
Index of Terms and Symbols 259
References 261
Index 267
Prelude xv
Preface xvii
Acknowledgments xix
1 The Importance of Data Quality 1
1.0 Introduction 1
1.1 Understanding the Implications of Data Quality 1
1.2 The Data Management Function 4
1.3 The Solution Strategy 6
1.4 Guide to This Book 6
Section I Building a Data Quality Program 2 The Data Quality Operating
Model 13
2.0 Introduction 13
2.1 Data Quality Foundational Capabilities 13
2.1.1 Program Strategy and Governance 14
2.1.2 Skilled Data Quality Resources 14
2.1.3 Technology Infrastructure and Metadata 15
2.1.4 Data Profi ling and Analytics 15
2.1.5 Data Integration 15
2.1.6 Data Assessment 16
2.1.7 Issues Resolution (IR) 16
2.1.8 Data Quality Monitoring and Control 16
2.2 The Data Quality Methodology 17
2.2.1 Establish a Data Quality Program 17
2.2.2 Conduct a Current-State Analysis 17
2.2.3 Strengthen Data Quality Capability through Data Quality Projects 18
2.2.4 Monitor the Ongoing Production Environment and Measure Data Quality
Improvement Effectiveness 18
2.2.5 Detailed Discussion on Establishing the Data Quality Program 18
2.2.6 Assess the Current State of Data Quality 21
2.3 Conclusions 22
3 The DAIC Approach 23
3.0 Introduction 23
3.1 Six Sigma Methodologies 23
3.1.1 Development of Six Sigma Methodologies 25
3.2 DAIC Approach for Data Quality 28
3.2.1 The Defi ne Phase 28
3.2.2 The Assess Phase 31
3.2.3 The Improve Phase 36
3.2.4 The Control Phase (Monitor and Measure) 37
3.3 Conclusions 40
Section II Executing a Data Quality Program 4 Quantification of the Impact
of Data Quality 43
4.0 Introduction 43
4.1 Building a Data Quality Cost Quantifi cation Framework 43
4.1.1 The Cost Waterfall 44
4.1.2 Prioritization Matrix 46
4.1.3 Remediation and Return on Investment 50
4.2 A Trading Offi ce Illustrative Example 51
4.3 Conclusions 54
5 Statistical Process Control and Its Relevance in Data Quality Monitoring
and Reporting 55
5.0 Introduction 55
5.1 What Is Statistical Process Control? 55
5.1.1 Common Causes and Special Causes 57
5.2 Control Charts 59
5.2.1 Different Types of Data 59
5.2.2 Sample and Sample Parameters 60
5.2.3 Construction of Attribute Control Charts 62
5.2.4 Construction of Variable Control Charts 65
5.2.5 Other Control Charts 67
5.2.6 Multivariate Process Control Charts 69
5.3 Relevance of Statistical Process Control in Data Quality Monitoring and
Reporting 69
5.4 Conclusions 70
6 Critical Data Elements: Identification, Validation, and Assessment 71
6.0 Introduction 71
6.1 Identifi cation of Critical Data Elements 71
6.1.1 Data Elements and Critical Data Elements 71
6.1.2 CDE Rationalization Matrix 72
6.2 Assessment of Critical Data Elements 75
6.2.1 Data Quality Dimensions 76
6.2.2 Data Quality Business Rules 78
6.2.3 Data Profi ling 79
6.2.4 Measurement of Data Quality Scores 80
6.2.5 Results Recording and Reporting (Scorecard) 80
6.3 Conclusions 82
7 Prioritization of Critical Data Elements (Funnel Approach) 83
7.0 Introduction 83
7.1 The Funnel Methodology (Statistical Analysis for CDE Reduction) 83
7.1.1 Correlation and Regression Analysis for Continuous CDEs 85
7.1.2 Association Analysis for Discrete CDEs 88
7.1.3 Signal-to-Noise Ratios Analysis 90
7.2 Case Study: Basel II 91
7.2.1 Basel II: CDE Rationalization Matrix 91
7.2.2 Basel II: Correlation and Regression Analysis 94
7.2.3 Basel II: Signal-to-Noise (S/N) Ratios 96
7.3 Conclusions 99
8 Data Quality Monitoring and Reporting Scorecards 101
8.0 Introduction 101
8.1 Development of the DQ Scorecards 102
8.2 Analytical Framework (ANOVA, SPCs, Thresholds, Heat Maps) 102
8.2.1 Thresholds and Heat Maps 103
8.2.2 Analysis of Variance (ANOVA) and SPC Charts 107
8.3 Application of the Framework 109
8.4 Conclusions 112
9 Data Quality Issue Resolution 113
9.0 Introduction 113
9.1 Description of the Methodology 113
9.2 Data Quality Methodology 114
9.3 Process Quality/Six Sigma Approach 115
9.4 Case Study: Issue Resolution Process Reengineering 117
9.5 Conclusions 119
10 Information System Testing 121
10.0 Introduction 121
10.1 Typical System Arrangement 122
10.1.1 The Role of Orthogonal Arrays 123
10.2 Method of System Testing 123
10.2.1 Study of Two-Factor Combinations 123
10.2.2 Construction of Combination Tables 124
10.3 MTS Software Testing 126
10.4 Case Study: A Japanese Software Company 130
10.5 Case Study: A Finance Company 133
10.6 Conclusions 138
11 Statistical Approach for Data Tracing 139
11.0 Introduction 139
11.1 Data Tracing Methodology 139
11.1.1 Statistical Sampling 142
11.2 Case Study: Tracing 144
11.2.1 Analysis of Test Cases and CDE Prioritization 144
11.3 Data Lineage through Data Tracing 149
11.4 Conclusions 151
12 Design and Development of Multivariate Diagnostic Systems 153
12.0 Introduction 153
12.1 The Mahalanobis-Taguchi Strategy 153
12.1.1 The Gram Schmidt Orthogonalization Process 155
12.2 Stages in MTS 158
12.3 The Role of Orthogonal Arrays and Signal-to-Noise Ratio in
Multivariate Diagnosis 159
12.3.1 The Role of Orthogonal Arrays 159
12.3.2 The Role of S/N Ratios in MTS 161
12.3.3 Types of S/N Ratios 162
12.3.4 Direction of Abnormals 164
12.4 A Medical Diagnosis Example 172
12.5 Case Study: Improving Client Experience 175
12.5.1 Improvements Made Based on Recommendations from MTS Analysis 177
12.6 Case Study: Understanding the Behavior Patterns of Defaulting
Customers 178
12.7 Case Study: Marketing 180
12.7.1 Construction of the Reference Group 181
12.7.2 Validation of the Scale 181
12.7.3 Identification of Useful Variables 181
12.8 Case Study: Gear Motor Assembly 182
12.8.1 Apparatus 183
12.8.2 Sensors 184
12.8.3 High-Resolution Encoder 184
12.8.4 Life Test 185
12.8.5 Characterization 185
12.8.6 Construction of the Reference Group or Mahalanobis Space 186
12.8.7 Validation of the MTS Scale 187
12.8.8 Selection of Useful Variables 188
12.9 Conclusions 189
13 Data Analytics 191
13.0 Introduction 191
13.1 Data and Analytics as Key Resources 191
13.1.1 Different Types of Analytics 193
13.1.2 Requirements for Executing Analytics 195
13.1.3 Process of Executing Analytics 196
13.2 Data Innovation 197
13.2.1 Big Data 198
13.2.2 Big Data Analytics 199
13.2.3 Big Data Analytics Operating Model 206
13.2.4 Big Data Analytics Projects: Examples 207
13.3 Conclusions 208
14. Building a Data Quality Practices Center 209
14.0 Introduction 209
14.1 Building a DQPC 209
14.2 Conclusions 211
Appendix A 213
Equations for Signal-to-Noise (S/N) Ratios 213
Nondynamic S/N Ratios 213
Dynamic S/N Ratios 214
Appendix B 217
Matrix Theory: Related Topics 217
What Is a Matrix? 217
Appendix C 221
Some Useful Orthogonal Arrays 221
Two-Level Orthogonal Arrays 221
Three-Level Orthogonal Arrays 255
Index of Terms and Symbols 259
References 261
Index 267