Computational and Statistical Approaches to Genomics (eBook, PDF)
149,79 €
inkl. MwSt.
Sofort per Download lieferbar
Computational and Statistical Approaches to Genomics (eBook, PDF)
- Format: PDF
- Merkliste
- Auf die Merkliste
- Bewerten Bewerten
- Teilen
- Produkt teilen
- Produkterinnerung
- Produkterinnerung
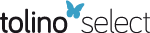
Bitte loggen Sie sich zunächst in Ihr Kundenkonto ein oder registrieren Sie sich bei
bücher.de, um das eBook-Abo tolino select nutzen zu können.
Hier können Sie sich einloggen
Hier können Sie sich einloggen
Sie sind bereits eingeloggt. Klicken Sie auf 2. tolino select Abo, um fortzufahren.
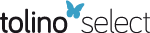
Bitte loggen Sie sich zunächst in Ihr Kundenkonto ein oder registrieren Sie sich bei bücher.de, um das eBook-Abo tolino select nutzen zu können.
The 2 nd edition of this book adds 8 new contributors to reflect a modern cutting edge approach to genomics. The expanded scope includes coverage of statistical issues on single nucleotide polymorphism analysis array, CGH analysis, SAGE analysis, gene shaving and related methods for microarray data analysis, and cross-hybridization issues on oligo arrays. The authors of the 17 original chapters have updated the contents of their chapters, including references, on such topics as the development of novel engineering, statistical and computational principles, as well as methods, models, and tools from these disciplines applied to genomics.…mehr
- Geräte: PC
- ohne Kopierschutz
- eBook Hilfe
- Größe: 6.29MB
- Upload möglich
Andere Kunden interessierten sich auch für
- Nucleic Acids Hybridization (eBook, PDF)149,79 €
- Methods of Microarray Data Analysis V (eBook, PDF)96,29 €
- RNA Towards Medicine (eBook, PDF)227,95 €
- Gary H. PerdewRegulation of Gene Expression (eBook, PDF)96,29 €
- Experimental Glycoscience (eBook, PDF)96,29 €
- Zinc Finger Proteins (eBook, PDF)149,79 €
- Advances in Computational Biology (eBook, PDF)255,73 €
-
-
-
The 2nd edition of this book adds 8 new contributors to reflect a modern cutting edge approach to genomics. The expanded scope includes coverage of statistical issues on single nucleotide polymorphism analysis array, CGH analysis, SAGE analysis, gene shaving and related methods for microarray data analysis, and cross-hybridization issues on oligo arrays. The authors of the 17 original chapters have updated the contents of their chapters, including references, on such topics as the development of novel engineering, statistical and computational principles, as well as methods, models, and tools from these disciplines applied to genomics.
Produktdetails
- Produktdetails
- Verlag: Springer US
- Erscheinungstermin: 26. Dezember 2007
- Englisch
- ISBN-13: 9780387262888
- Artikelnr.: 37287354
- Verlag: Springer US
- Erscheinungstermin: 26. Dezember 2007
- Englisch
- ISBN-13: 9780387262888
- Artikelnr.: 37287354
Ilya Shmulevich received his Ph.D. degree in Electrical and Computer Engineering from Purdue University, West Lafayette, Indiana in 1997. In 1997-1998, he was a postdoctoral researcher at the Nijmegen Institute for Cognition and Information at the University of Nijmegen and National Research Institute for Mathematics and Computer Science at the University of Amsterdam in The Netherlands, where he studied computational models of music perception and recognition. In 1998-2000, he worked as a senior researcher at the Tampere International Center for Signal Processing at the Signal Processing Laboratory in Tampere University of Technology, Tampere, Finland. Presently, he is an Assistant Professor at the Cancer Genomics Laboratory at The University of Texas M. D. Anderson Cancer Center in Houston, TX.
Wei Zhang received his Ph.D. degree from The University of Texas Graduate School of Biomedical Sciences. He joined the faculty of M. D. Anderson Cancer Center in 1994 where he is currently an Associate Professor and the Director of the Cancer Genomics Core Laboratory. Dr. Zhang's research programs include cancer genomics and informatics, tumor suppressor genes, and signal transduction studies. Dr. Zhang is an Associate Editor for American Association of Cancer Research journal Clinical Cancer Research, and serves on editorial board of journals Cancer Biology and Therapy, Histology and Histopathology, International Journal of Oncology, BMC Genomics, Technology in Cancer Research and Treatment, and Molecular Cancer.
Wei Zhang received his Ph.D. degree from The University of Texas Graduate School of Biomedical Sciences. He joined the faculty of M. D. Anderson Cancer Center in 1994 where he is currently an Associate Professor and the Director of the Cancer Genomics Core Laboratory. Dr. Zhang's research programs include cancer genomics and informatics, tumor suppressor genes, and signal transduction studies. Dr. Zhang is an Associate Editor for American Association of Cancer Research journal Clinical Cancer Research, and serves on editorial board of journals Cancer Biology and Therapy, Histology and Histopathology, International Journal of Oncology, BMC Genomics, Technology in Cancer Research and Treatment, and Molecular Cancer.
Microarray Image Analysis and Gene Expression Ratio Statistics.- Statistical Considerations in the Assessment of cDNA Microarray Data Obtained Using Amplification.- Sources of Variation in Microarray Experiments.- Studentizing Microarray Data.- Exploratory Clustering of Gene Expression Profiles of Mutated Yeast Strains.- Selecting Informative Genes for Cancer Classification Using Gene Expression Data.- Finding Functional Structures in Ggioma Gene-Expressions Using Gene Shaving Clustering and MDL Principle.- Design Issues and Comparison of Methods for Microarray-Based Classification.- Analyzing Protein Sequences Using Signal Analysis Techniques.- Scale-Dependent Statistics of the Numbers of Transcripts and Protein Sequences Encoded in the Genome.- Statistical Methods in Serial Analysis of Gene Expression (Sage).- Normalized Maximum Likelihood Models for Boolean Regression with Application to Prediction and Classification in Genomics.- Inference of Genetic Regulatory Networks via Best-Fit Extensions.- Regularization and Noise Injection for Improving Genetic Network Models.- Parallel Computation and Visualization Tools for Codetermination Analysis of Multivariate Gene Expression Relations.- Single Nucleotide Polymorphisms and Their Applications.- The Contribution of Alternative Transcription and Alternative Splicing to the Complexity of Mammalian Transcriptomes.- Computational Imaging, and Statistical Analysis of Tissue Microarrays: Quantitative Automated Analysis of Tissue Microarrays.
Microarray Image Analysis and Gene Expression Ratio Statistics.- Statistical Considerations in the Assessment of cDNA Microarray Data Obtained Using Amplification.- Sources of Variation in Microarray Experiments.- Studentizing Microarray Data.- Exploratory Clustering of Gene Expression Profiles of Mutated Yeast Strains.- Selecting Informative Genes for Cancer Classification Using Gene Expression Data.- Finding Functional Structures in Ggioma Gene-Expressions Using Gene Shaving Clustering and MDL Principle.- Design Issues and Comparison of Methods for Microarray-Based Classification.- Analyzing Protein Sequences Using Signal Analysis Techniques.- Scale-Dependent Statistics of the Numbers of Transcripts and Protein Sequences Encoded in the Genome.- Statistical Methods in Serial Analysis of Gene Expression (Sage).- Normalized Maximum Likelihood Models for Boolean Regression with Application to Prediction and Classification in Genomics.- Inference of Genetic Regulatory Networks via Best-Fit Extensions.- Regularization and Noise Injection for Improving Genetic Network Models.- Parallel Computation and Visualization Tools for Codetermination Analysis of Multivariate Gene Expression Relations.- Single Nucleotide Polymorphisms and Their Applications.- The Contribution of Alternative Transcription and Alternative Splicing to the Complexity of Mammalian Transcriptomes.- Computational Imaging, and Statistical Analysis of Tissue Microarrays: Quantitative Automated Analysis of Tissue Microarrays.
Microarray Image Analysis and Gene Expression Ratio Statistics.- Statistical Considerations in the Assessment of cDNA Microarray Data Obtained Using Amplification.- Sources of Variation in Microarray Experiments.- Studentizing Microarray Data.- Exploratory Clustering of Gene Expression Profiles of Mutated Yeast Strains.- Selecting Informative Genes for Cancer Classification Using Gene Expression Data.- Finding Functional Structures in Ggioma Gene-Expressions Using Gene Shaving Clustering and MDL Principle.- Design Issues and Comparison of Methods for Microarray-Based Classification.- Analyzing Protein Sequences Using Signal Analysis Techniques.- Scale-Dependent Statistics of the Numbers of Transcripts and Protein Sequences Encoded in the Genome.- Statistical Methods in Serial Analysis of Gene Expression (Sage).- Normalized Maximum Likelihood Models for Boolean Regression with Application to Prediction and Classification in Genomics.- Inference of Genetic Regulatory Networks via Best-Fit Extensions.- Regularization and Noise Injection for Improving Genetic Network Models.- Parallel Computation and Visualization Tools for Codetermination Analysis of Multivariate Gene Expression Relations.- Single Nucleotide Polymorphisms and Their Applications.- The Contribution of Alternative Transcription and Alternative Splicing to the Complexity of Mammalian Transcriptomes.- Computational Imaging, and Statistical Analysis of Tissue Microarrays: Quantitative Automated Analysis of Tissue Microarrays.
Microarray Image Analysis and Gene Expression Ratio Statistics.- Statistical Considerations in the Assessment of cDNA Microarray Data Obtained Using Amplification.- Sources of Variation in Microarray Experiments.- Studentizing Microarray Data.- Exploratory Clustering of Gene Expression Profiles of Mutated Yeast Strains.- Selecting Informative Genes for Cancer Classification Using Gene Expression Data.- Finding Functional Structures in Ggioma Gene-Expressions Using Gene Shaving Clustering and MDL Principle.- Design Issues and Comparison of Methods for Microarray-Based Classification.- Analyzing Protein Sequences Using Signal Analysis Techniques.- Scale-Dependent Statistics of the Numbers of Transcripts and Protein Sequences Encoded in the Genome.- Statistical Methods in Serial Analysis of Gene Expression (Sage).- Normalized Maximum Likelihood Models for Boolean Regression with Application to Prediction and Classification in Genomics.- Inference of Genetic Regulatory Networks via Best-Fit Extensions.- Regularization and Noise Injection for Improving Genetic Network Models.- Parallel Computation and Visualization Tools for Codetermination Analysis of Multivariate Gene Expression Relations.- Single Nucleotide Polymorphisms and Their Applications.- The Contribution of Alternative Transcription and Alternative Splicing to the Complexity of Mammalian Transcriptomes.- Computational Imaging, and Statistical Analysis of Tissue Microarrays: Quantitative Automated Analysis of Tissue Microarrays.