David Skillicorn
Cyberspace, Data Analytics, and Policing (eBook, PDF)
47,95 €
47,95 €
inkl. MwSt.
Sofort per Download lieferbar
24 °P sammeln
47,95 €
Als Download kaufen
47,95 €
inkl. MwSt.
Sofort per Download lieferbar
24 °P sammeln
Jetzt verschenken
Alle Infos zum eBook verschenken
47,95 €
inkl. MwSt.
Sofort per Download lieferbar
Alle Infos zum eBook verschenken
24 °P sammeln
David Skillicorn
Cyberspace, Data Analytics, and Policing (eBook, PDF)
- Format: PDF
- Merkliste
- Auf die Merkliste
- Bewerten Bewerten
- Teilen
- Produkt teilen
- Produkterinnerung
- Produkterinnerung
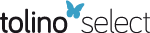
Bitte loggen Sie sich zunächst in Ihr Kundenkonto ein oder registrieren Sie sich bei
bücher.de, um das eBook-Abo tolino select nutzen zu können.
Hier können Sie sich einloggen
Hier können Sie sich einloggen
Sie sind bereits eingeloggt. Klicken Sie auf 2. tolino select Abo, um fortzufahren.
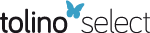
Bitte loggen Sie sich zunächst in Ihr Kundenkonto ein oder registrieren Sie sich bei bücher.de, um das eBook-Abo tolino select nutzen zu können.
Cyberspace, Data Analytics, and Policing surveys the changes that cyberspace has brought to criminality and to policing with enough technical content to expose the issues and suggest ways in which law enforcement organizations can adapt.
- Geräte: PC
- ohne Kopierschutz
- eBook Hilfe
- Größe: 12.6MB
Andere Kunden interessierten sich auch für
- David SkillicornCyberspace, Data Analytics, and Policing (eBook, ePUB)47,95 €
- David B. SkillicornCybersecurity for Everyone (eBook, PDF)21,95 €
- Tine MunkThe Rise of Politically Motivated Cyber Attacks (eBook, PDF)42,95 €
- Victoria BainesRhetoric of InSecurity (eBook, PDF)40,95 €
- Cybersecurity and Data Science Innovations for Sustainable Development of HEICC (eBook, PDF)52,95 €
- Recent Advances in Security, Privacy, and Trust for Internet of Things (IoT) and Cyber-Physical Systems (CPS) (eBook, PDF)51,95 €
- Financial Crimes (eBook, PDF)48,95 €
-
-
-
Cyberspace, Data Analytics, and Policing surveys the changes that cyberspace has brought to criminality and to policing with enough technical content to expose the issues and suggest ways in which law enforcement organizations can adapt.
Dieser Download kann aus rechtlichen Gründen nur mit Rechnungsadresse in A, B, BG, CY, CZ, D, DK, EW, E, FIN, F, GR, HR, H, IRL, I, LT, L, LR, M, NL, PL, P, R, S, SLO, SK ausgeliefert werden.
Produktdetails
- Produktdetails
- Verlag: Taylor & Francis
- Seitenzahl: 274
- Erscheinungstermin: 17. November 2021
- Englisch
- ISBN-13: 9781000465587
- Artikelnr.: 62361901
- Verlag: Taylor & Francis
- Seitenzahl: 274
- Erscheinungstermin: 17. November 2021
- Englisch
- ISBN-13: 9781000465587
- Artikelnr.: 62361901
- Herstellerkennzeichnung Die Herstellerinformationen sind derzeit nicht verfügbar.
David B. Skillicorn is a professor at the School of Computing, Queen's University, Canada.
Preface
List of Figures
List of Tables
Introduction
Cyberspace
2.1 What is cyberspace?
2.2 The impact of cyberspace
2.3 Identity and authentication
2.4 Encryption
2.5 Crime is changing
2.6 Policing is changing
New opportunities for criminality
3.1 Unprecedented access to information
3.2 Crimes directed against cyberspace
3.2.1 Malware
3.2.2 Crimes of destruction
3.2.3 Monetized cybercrimes
3.2.4 Data theft crimes
3.2.5 Secondary markets
3.3 Crimes that rely on cyberspace
3.3.1 Spam, scams, and cons
3.3.2 Financial crime
3.3.3 Online shopping
3.3.4 Crimes against children
3.4 Crimes done differently because of cyberspace
3.4.1 Disseminating hatred
3.4.2 Selling drugs
3.4.3 Stalking and crime preparation
3.4.4 Digital vigilantes
3.5 Money laundering
3.5.1 Cash
3.5.2 The financial system
3.5.3 International money laundering
3.5.4 Cryptocurrencies
3.6 Overlap with violent extremism
New ways for criminals to interact
4.1 Criminal collaboration
4.2 Planning together
4.3 Information sharing
4.3.1 Sharing techniques
4.3.2 Sharing resources
4.3.3 Sharing vulnerabilities
4.4 International interactions
Data analytics makes criminals easier to find
5.1 Understanding by deduction
5.2 Understanding by induction
5.3 Subverting data analytics
5.4 Intelligence-led policing
5.5 Hot spot policing
5.5.1 Place
5.5.2 Time
5.5.3 Weather
5.5.4 People involved
5.5.5 Social network position
5.6 Exploiting skewed distributions
Data collection
6.1 Ways to collect data
6.2 Types of data collected
6.2.1 Focused data
6.2.2 Large volume data
6.2.3 Incident data
6.2.4 Spatial data
6.2.5 Temporal data
6.2.6 Non-crime data
6.2.7 Data fusion
6.2.8 Protecting data collected by law enforcement
6.3 Issues around data collection
6.3.1 Suspicion
6.3.2 Wholesale data collection
6.3.3 Privacy
6.3.4 Racism and other -isms
6.3.5 Errors
6.3.6 Bias
6.3.7 Sabotaging data collection
6.3.8 Getting better data by sharing
Techniques for data analytics
7.1 Clustering
7.2 Prediction
7.3 Meta issues in prediction
7.3.1 Classification versus regression
7.3.2 Problems with the data
7.3.3 Why did the model make this prediction?
7.3.4 How good is this model?
7.3.5 Selecting attributes
7.3.6 Making predictions in stages
7.3.7 Bagging and boosting
7.3.8 Anomaly detection
7.3.9 Ranking
7.3.10 Should I make a prediction at all?
7.4 Prediction techniques
7.4.1 Counting techniques
7.4.2 Optimization techniques
7.4.3 Other ensembles
7.5 Social network analysis
7.6 Natural language analytics
7.7 Making data analytics available
7.8 Demonstrating compliance
Case studies
8.1 Predicting crime rates
8.2 Clustering RMS data
8.3 Geographical distribution patterns
8.4 Risk of gun violence
8.5 Copresence networks
8.6 Criminal networks with a purpose
8.7 Analyzing online posts
8.7.1 Detecting abusive language
8.7.2 Detecting intent
8.7.3 Deception
8.7.4 Detecting fraud in text
8.7.5 Detecting sellers in dark-web marketplaces
8.8 Behavior - detecting fraud from mouse movements
8.9 Understanding drug trafficking pathways
Law enforcement can use interaction too
9.1 Structured interaction through transnational organizations
9.2 Divisions within countries
9.3 Sharing of information about crimes
9.4 Sharing of data
9.5 Sharing models
9.6 International issues
Summary
Index
List of Figures
List of Tables
Introduction
Cyberspace
2.1 What is cyberspace?
2.2 The impact of cyberspace
2.3 Identity and authentication
2.4 Encryption
2.5 Crime is changing
2.6 Policing is changing
New opportunities for criminality
3.1 Unprecedented access to information
3.2 Crimes directed against cyberspace
3.2.1 Malware
3.2.2 Crimes of destruction
3.2.3 Monetized cybercrimes
3.2.4 Data theft crimes
3.2.5 Secondary markets
3.3 Crimes that rely on cyberspace
3.3.1 Spam, scams, and cons
3.3.2 Financial crime
3.3.3 Online shopping
3.3.4 Crimes against children
3.4 Crimes done differently because of cyberspace
3.4.1 Disseminating hatred
3.4.2 Selling drugs
3.4.3 Stalking and crime preparation
3.4.4 Digital vigilantes
3.5 Money laundering
3.5.1 Cash
3.5.2 The financial system
3.5.3 International money laundering
3.5.4 Cryptocurrencies
3.6 Overlap with violent extremism
New ways for criminals to interact
4.1 Criminal collaboration
4.2 Planning together
4.3 Information sharing
4.3.1 Sharing techniques
4.3.2 Sharing resources
4.3.3 Sharing vulnerabilities
4.4 International interactions
Data analytics makes criminals easier to find
5.1 Understanding by deduction
5.2 Understanding by induction
5.3 Subverting data analytics
5.4 Intelligence-led policing
5.5 Hot spot policing
5.5.1 Place
5.5.2 Time
5.5.3 Weather
5.5.4 People involved
5.5.5 Social network position
5.6 Exploiting skewed distributions
Data collection
6.1 Ways to collect data
6.2 Types of data collected
6.2.1 Focused data
6.2.2 Large volume data
6.2.3 Incident data
6.2.4 Spatial data
6.2.5 Temporal data
6.2.6 Non-crime data
6.2.7 Data fusion
6.2.8 Protecting data collected by law enforcement
6.3 Issues around data collection
6.3.1 Suspicion
6.3.2 Wholesale data collection
6.3.3 Privacy
6.3.4 Racism and other -isms
6.3.5 Errors
6.3.6 Bias
6.3.7 Sabotaging data collection
6.3.8 Getting better data by sharing
Techniques for data analytics
7.1 Clustering
7.2 Prediction
7.3 Meta issues in prediction
7.3.1 Classification versus regression
7.3.2 Problems with the data
7.3.3 Why did the model make this prediction?
7.3.4 How good is this model?
7.3.5 Selecting attributes
7.3.6 Making predictions in stages
7.3.7 Bagging and boosting
7.3.8 Anomaly detection
7.3.9 Ranking
7.3.10 Should I make a prediction at all?
7.4 Prediction techniques
7.4.1 Counting techniques
7.4.2 Optimization techniques
7.4.3 Other ensembles
7.5 Social network analysis
7.6 Natural language analytics
7.7 Making data analytics available
7.8 Demonstrating compliance
Case studies
8.1 Predicting crime rates
8.2 Clustering RMS data
8.3 Geographical distribution patterns
8.4 Risk of gun violence
8.5 Copresence networks
8.6 Criminal networks with a purpose
8.7 Analyzing online posts
8.7.1 Detecting abusive language
8.7.2 Detecting intent
8.7.3 Deception
8.7.4 Detecting fraud in text
8.7.5 Detecting sellers in dark-web marketplaces
8.8 Behavior - detecting fraud from mouse movements
8.9 Understanding drug trafficking pathways
Law enforcement can use interaction too
9.1 Structured interaction through transnational organizations
9.2 Divisions within countries
9.3 Sharing of information about crimes
9.4 Sharing of data
9.5 Sharing models
9.6 International issues
Summary
Index
Preface
List of Figures
List of Tables
Introduction
Cyberspace
2.1 What is cyberspace?
2.2 The impact of cyberspace
2.3 Identity and authentication
2.4 Encryption
2.5 Crime is changing
2.6 Policing is changing
New opportunities for criminality
3.1 Unprecedented access to information
3.2 Crimes directed against cyberspace
3.2.1 Malware
3.2.2 Crimes of destruction
3.2.3 Monetized cybercrimes
3.2.4 Data theft crimes
3.2.5 Secondary markets
3.3 Crimes that rely on cyberspace
3.3.1 Spam, scams, and cons
3.3.2 Financial crime
3.3.3 Online shopping
3.3.4 Crimes against children
3.4 Crimes done differently because of cyberspace
3.4.1 Disseminating hatred
3.4.2 Selling drugs
3.4.3 Stalking and crime preparation
3.4.4 Digital vigilantes
3.5 Money laundering
3.5.1 Cash
3.5.2 The financial system
3.5.3 International money laundering
3.5.4 Cryptocurrencies
3.6 Overlap with violent extremism
New ways for criminals to interact
4.1 Criminal collaboration
4.2 Planning together
4.3 Information sharing
4.3.1 Sharing techniques
4.3.2 Sharing resources
4.3.3 Sharing vulnerabilities
4.4 International interactions
Data analytics makes criminals easier to find
5.1 Understanding by deduction
5.2 Understanding by induction
5.3 Subverting data analytics
5.4 Intelligence-led policing
5.5 Hot spot policing
5.5.1 Place
5.5.2 Time
5.5.3 Weather
5.5.4 People involved
5.5.5 Social network position
5.6 Exploiting skewed distributions
Data collection
6.1 Ways to collect data
6.2 Types of data collected
6.2.1 Focused data
6.2.2 Large volume data
6.2.3 Incident data
6.2.4 Spatial data
6.2.5 Temporal data
6.2.6 Non-crime data
6.2.7 Data fusion
6.2.8 Protecting data collected by law enforcement
6.3 Issues around data collection
6.3.1 Suspicion
6.3.2 Wholesale data collection
6.3.3 Privacy
6.3.4 Racism and other -isms
6.3.5 Errors
6.3.6 Bias
6.3.7 Sabotaging data collection
6.3.8 Getting better data by sharing
Techniques for data analytics
7.1 Clustering
7.2 Prediction
7.3 Meta issues in prediction
7.3.1 Classification versus regression
7.3.2 Problems with the data
7.3.3 Why did the model make this prediction?
7.3.4 How good is this model?
7.3.5 Selecting attributes
7.3.6 Making predictions in stages
7.3.7 Bagging and boosting
7.3.8 Anomaly detection
7.3.9 Ranking
7.3.10 Should I make a prediction at all?
7.4 Prediction techniques
7.4.1 Counting techniques
7.4.2 Optimization techniques
7.4.3 Other ensembles
7.5 Social network analysis
7.6 Natural language analytics
7.7 Making data analytics available
7.8 Demonstrating compliance
Case studies
8.1 Predicting crime rates
8.2 Clustering RMS data
8.3 Geographical distribution patterns
8.4 Risk of gun violence
8.5 Copresence networks
8.6 Criminal networks with a purpose
8.7 Analyzing online posts
8.7.1 Detecting abusive language
8.7.2 Detecting intent
8.7.3 Deception
8.7.4 Detecting fraud in text
8.7.5 Detecting sellers in dark-web marketplaces
8.8 Behavior - detecting fraud from mouse movements
8.9 Understanding drug trafficking pathways
Law enforcement can use interaction too
9.1 Structured interaction through transnational organizations
9.2 Divisions within countries
9.3 Sharing of information about crimes
9.4 Sharing of data
9.5 Sharing models
9.6 International issues
Summary
Index
List of Figures
List of Tables
Introduction
Cyberspace
2.1 What is cyberspace?
2.2 The impact of cyberspace
2.3 Identity and authentication
2.4 Encryption
2.5 Crime is changing
2.6 Policing is changing
New opportunities for criminality
3.1 Unprecedented access to information
3.2 Crimes directed against cyberspace
3.2.1 Malware
3.2.2 Crimes of destruction
3.2.3 Monetized cybercrimes
3.2.4 Data theft crimes
3.2.5 Secondary markets
3.3 Crimes that rely on cyberspace
3.3.1 Spam, scams, and cons
3.3.2 Financial crime
3.3.3 Online shopping
3.3.4 Crimes against children
3.4 Crimes done differently because of cyberspace
3.4.1 Disseminating hatred
3.4.2 Selling drugs
3.4.3 Stalking and crime preparation
3.4.4 Digital vigilantes
3.5 Money laundering
3.5.1 Cash
3.5.2 The financial system
3.5.3 International money laundering
3.5.4 Cryptocurrencies
3.6 Overlap with violent extremism
New ways for criminals to interact
4.1 Criminal collaboration
4.2 Planning together
4.3 Information sharing
4.3.1 Sharing techniques
4.3.2 Sharing resources
4.3.3 Sharing vulnerabilities
4.4 International interactions
Data analytics makes criminals easier to find
5.1 Understanding by deduction
5.2 Understanding by induction
5.3 Subverting data analytics
5.4 Intelligence-led policing
5.5 Hot spot policing
5.5.1 Place
5.5.2 Time
5.5.3 Weather
5.5.4 People involved
5.5.5 Social network position
5.6 Exploiting skewed distributions
Data collection
6.1 Ways to collect data
6.2 Types of data collected
6.2.1 Focused data
6.2.2 Large volume data
6.2.3 Incident data
6.2.4 Spatial data
6.2.5 Temporal data
6.2.6 Non-crime data
6.2.7 Data fusion
6.2.8 Protecting data collected by law enforcement
6.3 Issues around data collection
6.3.1 Suspicion
6.3.2 Wholesale data collection
6.3.3 Privacy
6.3.4 Racism and other -isms
6.3.5 Errors
6.3.6 Bias
6.3.7 Sabotaging data collection
6.3.8 Getting better data by sharing
Techniques for data analytics
7.1 Clustering
7.2 Prediction
7.3 Meta issues in prediction
7.3.1 Classification versus regression
7.3.2 Problems with the data
7.3.3 Why did the model make this prediction?
7.3.4 How good is this model?
7.3.5 Selecting attributes
7.3.6 Making predictions in stages
7.3.7 Bagging and boosting
7.3.8 Anomaly detection
7.3.9 Ranking
7.3.10 Should I make a prediction at all?
7.4 Prediction techniques
7.4.1 Counting techniques
7.4.2 Optimization techniques
7.4.3 Other ensembles
7.5 Social network analysis
7.6 Natural language analytics
7.7 Making data analytics available
7.8 Demonstrating compliance
Case studies
8.1 Predicting crime rates
8.2 Clustering RMS data
8.3 Geographical distribution patterns
8.4 Risk of gun violence
8.5 Copresence networks
8.6 Criminal networks with a purpose
8.7 Analyzing online posts
8.7.1 Detecting abusive language
8.7.2 Detecting intent
8.7.3 Deception
8.7.4 Detecting fraud in text
8.7.5 Detecting sellers in dark-web marketplaces
8.8 Behavior - detecting fraud from mouse movements
8.9 Understanding drug trafficking pathways
Law enforcement can use interaction too
9.1 Structured interaction through transnational organizations
9.2 Divisions within countries
9.3 Sharing of information about crimes
9.4 Sharing of data
9.5 Sharing models
9.6 International issues
Summary
Index