Data Analysis (eBook, PDF)
Redaktion: Govaert, Gérard
154,99 €
154,99 €
inkl. MwSt.
Sofort per Download lieferbar
0 °P sammeln
154,99 €
Als Download kaufen
154,99 €
inkl. MwSt.
Sofort per Download lieferbar
0 °P sammeln
Jetzt verschenken
Alle Infos zum eBook verschenken
154,99 €
inkl. MwSt.
Sofort per Download lieferbar
Alle Infos zum eBook verschenken
0 °P sammeln
Data Analysis (eBook, PDF)
Redaktion: Govaert, Gérard
- Format: PDF
- Merkliste
- Auf die Merkliste
- Bewerten Bewerten
- Teilen
- Produkt teilen
- Produkterinnerung
- Produkterinnerung
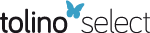
Bitte loggen Sie sich zunächst in Ihr Kundenkonto ein oder registrieren Sie sich bei
bücher.de, um das eBook-Abo tolino select nutzen zu können.
Hier können Sie sich einloggen
Hier können Sie sich einloggen
Sie sind bereits eingeloggt. Klicken Sie auf 2. tolino select Abo, um fortzufahren.
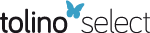
Bitte loggen Sie sich zunächst in Ihr Kundenkonto ein oder registrieren Sie sich bei bücher.de, um das eBook-Abo tolino select nutzen zu können.
The first part of this book is devoted to methods seeking relevant dimensions of data. The variables thus obtained provide a synthetic description which often results in a graphical representation of the data. After a general presentation of the discriminating analysis, the second part is devoted to clustering methods which constitute another method, often complementary to the methods described in the first part, to synthesize and to analyze the data. The book concludes by examining the links existing between data mining and data analysis.
- Geräte: PC
- ohne Kopierschutz
- eBook Hilfe
Andere Kunden interessierten sich auch für
- Simon MunzertAutomated Data Collection with R (eBook, PDF)60,99 €
- Data Analysis and Related Applications, Volume 2 (eBook, PDF)126,99 €
- Alan AndersonStatistics for Big Data For Dummies (eBook, PDF)15,99 €
- Data Analysis and Related Applications, Volume 1 (eBook, PDF)126,99 €
- George FernandezStatistical Data Mining Using SAS Applications (eBook, PDF)140,95 €
- Data Analysis and Related Applications 3 (eBook, PDF)142,99 €
- Boris MirkinClustering (eBook, PDF)64,95 €
-
-
-
The first part of this book is devoted to methods seeking relevant dimensions of data. The variables thus obtained provide a synthetic description which often results in a graphical representation of the data. After a general presentation of the discriminating analysis, the second part is devoted to clustering methods which constitute another method, often complementary to the methods described in the first part, to synthesize and to analyze the data. The book concludes by examining the links existing between data mining and data analysis.
Dieser Download kann aus rechtlichen Gründen nur mit Rechnungsadresse in D ausgeliefert werden.
Produktdetails
- Produktdetails
- Verlag: John Wiley & Sons
- Erscheinungstermin: 5. Januar 2010
- Englisch
- ISBN-13: 9780470610312
- Artikelnr.: 38189762
- Verlag: John Wiley & Sons
- Erscheinungstermin: 5. Januar 2010
- Englisch
- ISBN-13: 9780470610312
- Artikelnr.: 38189762
- Herstellerkennzeichnung Die Herstellerinformationen sind derzeit nicht verfügbar.
Gérard Govaert is Professor at the University of Technology of Compiègne, France. He is also a member of the CNRS Laboratory Heudiasyc (Heuristic and diagnostic of complex systems). His research interests include latent structure modeling, model selection, model-based cluster analysis, block clustering and statistical pattern recognition. He is one of the authors of the MIXMOD (MIXture MODelling) software.
Preface xiii
Chapter 1. Principal Component Analysis: Application to Statistical Process
Control 1
Gilbert SAPORTA, Ndèye NIANG
1.1. Introduction 1
1.2. Data table and related subspaces 2
1.3. Principal component analysis 8
1.4. Interpretation of PCA results 11
1.5. Application to statistical process control 18
1.6. Conclusion 22
1.7. Bibliography 23
Chapter 2. Correspondence Analysis: Extensions and Applications to the
Statistical Analysis of Sensory Data 25
Jérôme PAGÈS
2.1. Correspondence analysis 25
2.2. Multiple correspondence analysis 39
2.3. An example of application at the crossroads of CA and MCA 50
2.4. Conclusion: two other extensions 63
2.5. Bibliography 64
Chapter 3. Exploratory Projection Pursuit 67
Henri CAUSSINUS, Anne RUIZ-GAZEN
3.1. Introduction 67
3.2. General principles 68
3.3. Some indexes of interest: presentation and use 71
3.4. Generalized principal component analysis 76
3.5. Example 81
3.6. Further topics 86
3.7. Bibliography 89
Chapter 4. The Analysis of Proximity Data 93
Gerard D'AUBIGNY
4.1. Introduction 93
4.2. Representation of proximity data in a metric space 97
4.3. Isometric embedding and projection 103
4.4. Multidimensional scaling and approximation 108
4.5. Afielded application 122
4.6. Bibliography 139
Chapter 5. Statistical Modeling of Functional Data 149
Philippe BESSE, Hervé CARDOT
5.1. Introduction 149
5.2. Functional framework152
5.3. Principal components analysis 156
5.4. Linear regression models and extensions 161
5.5. Forecasting 169
5.6. Concluding remarks 176
5.7. Bibliography 177
Chapter 6. Discriminant Analysis 181
Gilles CELEUX
6.1. Introduction 181
6.2. Main steps in supervised classification 182
6.3. Standard methods in supervised classification 190
6.4. Recent advances 204
6.5. Conclusion 211
6.6. Bibliography 212
Chapter 7. Cluster Analysis 215
Mohamed NADIF, Gérard GOVAERT
7.1. Introduction 215
7.2. General principles 217
7.3. Hierarchical clustering 224
7.4. Partitional clustering: the k-means algorithm 233
7.5. Miscellaneous clustering methods 239
7.6. Block clustering 245
7.7. Conclusion 251
7.8. Bibliography 251
Chapter 8. Clustering and the Mixture Model 257
Gérard GOVAERT
8.1. Probabilistic approaches in cluster analysis 257
8.2. The mixture model 261
8.3. EM algorithm 263
8.4. Clustering and the mixture model 267
8.5.Gaussian mixture model 271
8.6. Binary variables 275
8.7. Qualitative variables 279
8.8. Implementation 282
8.9. Conclusion 284
8.10. Bibliography 284
Chapter 9. Spatial Data Clustering 289
Christophe AMBROISE, Mo DANG
9.1. Introduction 289
9.2. Non-probabilistic approaches 293
9.3. Markov random fields as models 295
9.4. Estimating the parameters for a Markov field 305
9.5. Application to numerical ecology 313
9.6. Bibliography 316
List of Authors 319
Index 323
Chapter 1. Principal Component Analysis: Application to Statistical Process
Control 1
Gilbert SAPORTA, Ndèye NIANG
1.1. Introduction 1
1.2. Data table and related subspaces 2
1.3. Principal component analysis 8
1.4. Interpretation of PCA results 11
1.5. Application to statistical process control 18
1.6. Conclusion 22
1.7. Bibliography 23
Chapter 2. Correspondence Analysis: Extensions and Applications to the
Statistical Analysis of Sensory Data 25
Jérôme PAGÈS
2.1. Correspondence analysis 25
2.2. Multiple correspondence analysis 39
2.3. An example of application at the crossroads of CA and MCA 50
2.4. Conclusion: two other extensions 63
2.5. Bibliography 64
Chapter 3. Exploratory Projection Pursuit 67
Henri CAUSSINUS, Anne RUIZ-GAZEN
3.1. Introduction 67
3.2. General principles 68
3.3. Some indexes of interest: presentation and use 71
3.4. Generalized principal component analysis 76
3.5. Example 81
3.6. Further topics 86
3.7. Bibliography 89
Chapter 4. The Analysis of Proximity Data 93
Gerard D'AUBIGNY
4.1. Introduction 93
4.2. Representation of proximity data in a metric space 97
4.3. Isometric embedding and projection 103
4.4. Multidimensional scaling and approximation 108
4.5. Afielded application 122
4.6. Bibliography 139
Chapter 5. Statistical Modeling of Functional Data 149
Philippe BESSE, Hervé CARDOT
5.1. Introduction 149
5.2. Functional framework152
5.3. Principal components analysis 156
5.4. Linear regression models and extensions 161
5.5. Forecasting 169
5.6. Concluding remarks 176
5.7. Bibliography 177
Chapter 6. Discriminant Analysis 181
Gilles CELEUX
6.1. Introduction 181
6.2. Main steps in supervised classification 182
6.3. Standard methods in supervised classification 190
6.4. Recent advances 204
6.5. Conclusion 211
6.6. Bibliography 212
Chapter 7. Cluster Analysis 215
Mohamed NADIF, Gérard GOVAERT
7.1. Introduction 215
7.2. General principles 217
7.3. Hierarchical clustering 224
7.4. Partitional clustering: the k-means algorithm 233
7.5. Miscellaneous clustering methods 239
7.6. Block clustering 245
7.7. Conclusion 251
7.8. Bibliography 251
Chapter 8. Clustering and the Mixture Model 257
Gérard GOVAERT
8.1. Probabilistic approaches in cluster analysis 257
8.2. The mixture model 261
8.3. EM algorithm 263
8.4. Clustering and the mixture model 267
8.5.Gaussian mixture model 271
8.6. Binary variables 275
8.7. Qualitative variables 279
8.8. Implementation 282
8.9. Conclusion 284
8.10. Bibliography 284
Chapter 9. Spatial Data Clustering 289
Christophe AMBROISE, Mo DANG
9.1. Introduction 289
9.2. Non-probabilistic approaches 293
9.3. Markov random fields as models 295
9.4. Estimating the parameters for a Markov field 305
9.5. Application to numerical ecology 313
9.6. Bibliography 316
List of Authors 319
Index 323
Preface xiii
Chapter 1. Principal Component Analysis: Application to Statistical Process
Control 1
Gilbert SAPORTA, Ndèye NIANG
1.1. Introduction 1
1.2. Data table and related subspaces 2
1.3. Principal component analysis 8
1.4. Interpretation of PCA results 11
1.5. Application to statistical process control 18
1.6. Conclusion 22
1.7. Bibliography 23
Chapter 2. Correspondence Analysis: Extensions and Applications to the
Statistical Analysis of Sensory Data 25
Jérôme PAGÈS
2.1. Correspondence analysis 25
2.2. Multiple correspondence analysis 39
2.3. An example of application at the crossroads of CA and MCA 50
2.4. Conclusion: two other extensions 63
2.5. Bibliography 64
Chapter 3. Exploratory Projection Pursuit 67
Henri CAUSSINUS, Anne RUIZ-GAZEN
3.1. Introduction 67
3.2. General principles 68
3.3. Some indexes of interest: presentation and use 71
3.4. Generalized principal component analysis 76
3.5. Example 81
3.6. Further topics 86
3.7. Bibliography 89
Chapter 4. The Analysis of Proximity Data 93
Gerard D'AUBIGNY
4.1. Introduction 93
4.2. Representation of proximity data in a metric space 97
4.3. Isometric embedding and projection 103
4.4. Multidimensional scaling and approximation 108
4.5. Afielded application 122
4.6. Bibliography 139
Chapter 5. Statistical Modeling of Functional Data 149
Philippe BESSE, Hervé CARDOT
5.1. Introduction 149
5.2. Functional framework152
5.3. Principal components analysis 156
5.4. Linear regression models and extensions 161
5.5. Forecasting 169
5.6. Concluding remarks 176
5.7. Bibliography 177
Chapter 6. Discriminant Analysis 181
Gilles CELEUX
6.1. Introduction 181
6.2. Main steps in supervised classification 182
6.3. Standard methods in supervised classification 190
6.4. Recent advances 204
6.5. Conclusion 211
6.6. Bibliography 212
Chapter 7. Cluster Analysis 215
Mohamed NADIF, Gérard GOVAERT
7.1. Introduction 215
7.2. General principles 217
7.3. Hierarchical clustering 224
7.4. Partitional clustering: the k-means algorithm 233
7.5. Miscellaneous clustering methods 239
7.6. Block clustering 245
7.7. Conclusion 251
7.8. Bibliography 251
Chapter 8. Clustering and the Mixture Model 257
Gérard GOVAERT
8.1. Probabilistic approaches in cluster analysis 257
8.2. The mixture model 261
8.3. EM algorithm 263
8.4. Clustering and the mixture model 267
8.5.Gaussian mixture model 271
8.6. Binary variables 275
8.7. Qualitative variables 279
8.8. Implementation 282
8.9. Conclusion 284
8.10. Bibliography 284
Chapter 9. Spatial Data Clustering 289
Christophe AMBROISE, Mo DANG
9.1. Introduction 289
9.2. Non-probabilistic approaches 293
9.3. Markov random fields as models 295
9.4. Estimating the parameters for a Markov field 305
9.5. Application to numerical ecology 313
9.6. Bibliography 316
List of Authors 319
Index 323
Chapter 1. Principal Component Analysis: Application to Statistical Process
Control 1
Gilbert SAPORTA, Ndèye NIANG
1.1. Introduction 1
1.2. Data table and related subspaces 2
1.3. Principal component analysis 8
1.4. Interpretation of PCA results 11
1.5. Application to statistical process control 18
1.6. Conclusion 22
1.7. Bibliography 23
Chapter 2. Correspondence Analysis: Extensions and Applications to the
Statistical Analysis of Sensory Data 25
Jérôme PAGÈS
2.1. Correspondence analysis 25
2.2. Multiple correspondence analysis 39
2.3. An example of application at the crossroads of CA and MCA 50
2.4. Conclusion: two other extensions 63
2.5. Bibliography 64
Chapter 3. Exploratory Projection Pursuit 67
Henri CAUSSINUS, Anne RUIZ-GAZEN
3.1. Introduction 67
3.2. General principles 68
3.3. Some indexes of interest: presentation and use 71
3.4. Generalized principal component analysis 76
3.5. Example 81
3.6. Further topics 86
3.7. Bibliography 89
Chapter 4. The Analysis of Proximity Data 93
Gerard D'AUBIGNY
4.1. Introduction 93
4.2. Representation of proximity data in a metric space 97
4.3. Isometric embedding and projection 103
4.4. Multidimensional scaling and approximation 108
4.5. Afielded application 122
4.6. Bibliography 139
Chapter 5. Statistical Modeling of Functional Data 149
Philippe BESSE, Hervé CARDOT
5.1. Introduction 149
5.2. Functional framework152
5.3. Principal components analysis 156
5.4. Linear regression models and extensions 161
5.5. Forecasting 169
5.6. Concluding remarks 176
5.7. Bibliography 177
Chapter 6. Discriminant Analysis 181
Gilles CELEUX
6.1. Introduction 181
6.2. Main steps in supervised classification 182
6.3. Standard methods in supervised classification 190
6.4. Recent advances 204
6.5. Conclusion 211
6.6. Bibliography 212
Chapter 7. Cluster Analysis 215
Mohamed NADIF, Gérard GOVAERT
7.1. Introduction 215
7.2. General principles 217
7.3. Hierarchical clustering 224
7.4. Partitional clustering: the k-means algorithm 233
7.5. Miscellaneous clustering methods 239
7.6. Block clustering 245
7.7. Conclusion 251
7.8. Bibliography 251
Chapter 8. Clustering and the Mixture Model 257
Gérard GOVAERT
8.1. Probabilistic approaches in cluster analysis 257
8.2. The mixture model 261
8.3. EM algorithm 263
8.4. Clustering and the mixture model 267
8.5.Gaussian mixture model 271
8.6. Binary variables 275
8.7. Qualitative variables 279
8.8. Implementation 282
8.9. Conclusion 284
8.10. Bibliography 284
Chapter 9. Spatial Data Clustering 289
Christophe AMBROISE, Mo DANG
9.1. Introduction 289
9.2. Non-probabilistic approaches 293
9.3. Markov random fields as models 295
9.4. Estimating the parameters for a Markov field 305
9.5. Application to numerical ecology 313
9.6. Bibliography 316
List of Authors 319
Index 323