Nirupam Chakraborti
Data-Driven Evolutionary Modeling in Materials Technology (eBook, ePUB)
58,95 €
58,95 €
inkl. MwSt.
Sofort per Download lieferbar
29 °P sammeln
58,95 €
Als Download kaufen
58,95 €
inkl. MwSt.
Sofort per Download lieferbar
29 °P sammeln
Jetzt verschenken
Alle Infos zum eBook verschenken
58,95 €
inkl. MwSt.
Sofort per Download lieferbar
Alle Infos zum eBook verschenken
29 °P sammeln
Nirupam Chakraborti
Data-Driven Evolutionary Modeling in Materials Technology (eBook, ePUB)
- Format: ePub
- Merkliste
- Auf die Merkliste
- Bewerten Bewerten
- Teilen
- Produkt teilen
- Produkterinnerung
- Produkterinnerung
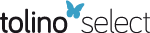
Bitte loggen Sie sich zunächst in Ihr Kundenkonto ein oder registrieren Sie sich bei
bücher.de, um das eBook-Abo tolino select nutzen zu können.
Hier können Sie sich einloggen
Hier können Sie sich einloggen
Sie sind bereits eingeloggt. Klicken Sie auf 2. tolino select Abo, um fortzufahren.
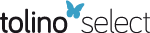
Bitte loggen Sie sich zunächst in Ihr Kundenkonto ein oder registrieren Sie sich bei bücher.de, um das eBook-Abo tolino select nutzen zu können.
This book presents the genetic and evolutionary, algorithms and strategies associated with pertinent issues in materials science domain. It discusses the procedures for evolutionary multi-objective optimization of objective functions including available professional and public domain codes and a gamut of recent applications.
- Geräte: eReader
- ohne Kopierschutz
- eBook Hilfe
- Größe: 44.6MB
Andere Kunden interessierten sich auch für
- Nirupam ChakrabortiData-Driven Evolutionary Modeling in Materials Technology (eBook, PDF)58,95 €
- Shubhabrata DattaSteel Informatics (eBook, ePUB)54,95 €
- Rajesh JhaArtificial Intelligence-Aided Materials Design (eBook, ePUB)48,95 €
- Machine Learning in 2D Materials Science (eBook, ePUB)131,95 €
- Metal Matrix Composites (eBook, ePUB)54,95 €
- Anshuman PatraOxide Dispersion Strengthened Refractory Alloys (eBook, ePUB)48,95 €
- Harshad K. D. H. BhadeshiaPearlite in Steels (eBook, ePUB)52,95 €
-
-
-
This book presents the genetic and evolutionary, algorithms and strategies associated with pertinent issues in materials science domain. It discusses the procedures for evolutionary multi-objective optimization of objective functions including available professional and public domain codes and a gamut of recent applications.
Dieser Download kann aus rechtlichen Gründen nur mit Rechnungsadresse in A, B, BG, CY, CZ, D, DK, EW, E, FIN, F, GR, HR, H, IRL, I, LT, L, LR, M, NL, PL, P, R, S, SLO, SK ausgeliefert werden.
Produktdetails
- Produktdetails
- Verlag: Taylor & Francis eBooks
- Seitenzahl: 318
- Erscheinungstermin: 15. September 2022
- Englisch
- ISBN-13: 9781000635867
- Artikelnr.: 65246383
- Verlag: Taylor & Francis eBooks
- Seitenzahl: 318
- Erscheinungstermin: 15. September 2022
- Englisch
- ISBN-13: 9781000635867
- Artikelnr.: 65246383
- Herstellerkennzeichnung Die Herstellerinformationen sind derzeit nicht verfügbar.
Professor Nirupam Chakraborti was educated in India and USA, receiving his B.Met.E from Jadavpur University, India, followed by an MS from New Mexico Tech, USA and PhD, PhD degrees from University of Washington, Seattle, USA. He joined Indian Institute of Technology, Kanpur as a member of the faculty in 1984 and switched to Indian Institute of Technology, Kharagpur in 2000.
Internationally known for his pioneering work on evolutionary computation in the area of Metallurgy and Materials, globally, Professor Chakraborti was rated among the top 2% highly cited researchers in the Materials area in 2000, as per Scopus records. A former Docent of Åbo Akademi, Finland, former Visiting Professors of Florida International University and POSTECH, Korea, he also taught and conducted research at several other academic institutions in Austria, Brazil, Finland, Germany, Italy and the US. An international symposium, under the KomPlasTech 2019, which is world's longest running conference series in the area of computational materials technology, was organized in Poland in 2019 to honor him. In 2020, an issue of a prominent Taylor of Francis journal, Materials and Manufacturing Processes was dedicated to him as well. In 2021 Indian Institute of Technology, Kharagpur and Indian Institute of Metals, a professional body, also organized another international seminar in his honor.
This book is a culmination of Professor Chakarborti's decades of research and teaching efforts in this area.
Internationally known for his pioneering work on evolutionary computation in the area of Metallurgy and Materials, globally, Professor Chakraborti was rated among the top 2% highly cited researchers in the Materials area in 2000, as per Scopus records. A former Docent of Åbo Akademi, Finland, former Visiting Professors of Florida International University and POSTECH, Korea, he also taught and conducted research at several other academic institutions in Austria, Brazil, Finland, Germany, Italy and the US. An international symposium, under the KomPlasTech 2019, which is world's longest running conference series in the area of computational materials technology, was organized in Poland in 2019 to honor him. In 2020, an issue of a prominent Taylor of Francis journal, Materials and Manufacturing Processes was dedicated to him as well. In 2021 Indian Institute of Technology, Kharagpur and Indian Institute of Metals, a professional body, also organized another international seminar in his honor.
This book is a culmination of Professor Chakarborti's decades of research and teaching efforts in this area.
1. Introduction
2. Data with random noise and its modeling
3. Nature inspired non-calculus optimization
4. Single-objective evolutionary algorithms
5. Multi-objective evolutionary optimization
6. Evolutionary learning and optimization using Neural Net paradigm
7. Evolutionary learning and optimization using Genetic Programming paradigm
8. The challenge of big data and Evolutionary Deep Learning
9. Software available in public domain and the commercial software
10. Applications in Iron and Steel making
11. Applications in chemical and metallurgical unit processing
12. Applications in Materials Design
13. Applications in Atomistic Materials Design
14. Applications in Manufacturing
15. Miscellaneous Applications
2. Data with random noise and its modeling
3. Nature inspired non-calculus optimization
4. Single-objective evolutionary algorithms
5. Multi-objective evolutionary optimization
6. Evolutionary learning and optimization using Neural Net paradigm
7. Evolutionary learning and optimization using Genetic Programming paradigm
8. The challenge of big data and Evolutionary Deep Learning
9. Software available in public domain and the commercial software
10. Applications in Iron and Steel making
11. Applications in chemical and metallurgical unit processing
12. Applications in Materials Design
13. Applications in Atomistic Materials Design
14. Applications in Manufacturing
15. Miscellaneous Applications
1. Introduction
2. Data with random noise and its modeling
3. Nature inspired non-calculus optimization
4. Single-objective evolutionary algorithms
5. Multi-objective evolutionary optimization
6. Evolutionary learning and optimization using Neural Net paradigm
7. Evolutionary learning and optimization using Genetic Programming
paradigm
8. The challenge of big data and Evolutionary Deep Learning
9. Software available in public domain and the commercial software
10. Applications in Iron and Steel making
11. Applications in chemical and metallurgical unit processing
12. Applications in Materials Design
13. Applications in Atomistic Materials Design
14. Applications in Manufacturing
15. Miscellaneous Applications
2. Data with random noise and its modeling
3. Nature inspired non-calculus optimization
4. Single-objective evolutionary algorithms
5. Multi-objective evolutionary optimization
6. Evolutionary learning and optimization using Neural Net paradigm
7. Evolutionary learning and optimization using Genetic Programming
paradigm
8. The challenge of big data and Evolutionary Deep Learning
9. Software available in public domain and the commercial software
10. Applications in Iron and Steel making
11. Applications in chemical and metallurgical unit processing
12. Applications in Materials Design
13. Applications in Atomistic Materials Design
14. Applications in Manufacturing
15. Miscellaneous Applications
1. Introduction
2. Data with random noise and its modeling
3. Nature inspired non-calculus optimization
4. Single-objective evolutionary algorithms
5. Multi-objective evolutionary optimization
6. Evolutionary learning and optimization using Neural Net paradigm
7. Evolutionary learning and optimization using Genetic Programming paradigm
8. The challenge of big data and Evolutionary Deep Learning
9. Software available in public domain and the commercial software
10. Applications in Iron and Steel making
11. Applications in chemical and metallurgical unit processing
12. Applications in Materials Design
13. Applications in Atomistic Materials Design
14. Applications in Manufacturing
15. Miscellaneous Applications
2. Data with random noise and its modeling
3. Nature inspired non-calculus optimization
4. Single-objective evolutionary algorithms
5. Multi-objective evolutionary optimization
6. Evolutionary learning and optimization using Neural Net paradigm
7. Evolutionary learning and optimization using Genetic Programming paradigm
8. The challenge of big data and Evolutionary Deep Learning
9. Software available in public domain and the commercial software
10. Applications in Iron and Steel making
11. Applications in chemical and metallurgical unit processing
12. Applications in Materials Design
13. Applications in Atomistic Materials Design
14. Applications in Manufacturing
15. Miscellaneous Applications
1. Introduction
2. Data with random noise and its modeling
3. Nature inspired non-calculus optimization
4. Single-objective evolutionary algorithms
5. Multi-objective evolutionary optimization
6. Evolutionary learning and optimization using Neural Net paradigm
7. Evolutionary learning and optimization using Genetic Programming
paradigm
8. The challenge of big data and Evolutionary Deep Learning
9. Software available in public domain and the commercial software
10. Applications in Iron and Steel making
11. Applications in chemical and metallurgical unit processing
12. Applications in Materials Design
13. Applications in Atomistic Materials Design
14. Applications in Manufacturing
15. Miscellaneous Applications
2. Data with random noise and its modeling
3. Nature inspired non-calculus optimization
4. Single-objective evolutionary algorithms
5. Multi-objective evolutionary optimization
6. Evolutionary learning and optimization using Neural Net paradigm
7. Evolutionary learning and optimization using Genetic Programming
paradigm
8. The challenge of big data and Evolutionary Deep Learning
9. Software available in public domain and the commercial software
10. Applications in Iron and Steel making
11. Applications in chemical and metallurgical unit processing
12. Applications in Materials Design
13. Applications in Atomistic Materials Design
14. Applications in Manufacturing
15. Miscellaneous Applications