Alfred Z. Spector, Peter Norvig, Chris Wiggins, Jeannette M. Wing
Data Science in Context (eBook, PDF)
Foundations, Challenges, Opportunities
29,95 €
29,95 €
inkl. MwSt.
Sofort per Download lieferbar
15 °P sammeln
29,95 €
Als Download kaufen
29,95 €
inkl. MwSt.
Sofort per Download lieferbar
15 °P sammeln
Jetzt verschenken
Alle Infos zum eBook verschenken
29,95 €
inkl. MwSt.
Sofort per Download lieferbar
Alle Infos zum eBook verschenken
15 °P sammeln
Alfred Z. Spector, Peter Norvig, Chris Wiggins, Jeannette M. Wing
Data Science in Context (eBook, PDF)
Foundations, Challenges, Opportunities
- Format: PDF
- Merkliste
- Auf die Merkliste
- Bewerten Bewerten
- Teilen
- Produkt teilen
- Produkterinnerung
- Produkterinnerung
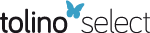
Bitte loggen Sie sich zunächst in Ihr Kundenkonto ein oder registrieren Sie sich bei
bücher.de, um das eBook-Abo tolino select nutzen zu können.
Hier können Sie sich einloggen
Hier können Sie sich einloggen
Sie sind bereits eingeloggt. Klicken Sie auf 2. tolino select Abo, um fortzufahren.
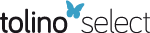
Bitte loggen Sie sich zunächst in Ihr Kundenkonto ein oder registrieren Sie sich bei bücher.de, um das eBook-Abo tolino select nutzen zu können.
Four leading experts convey the promise of data science and examine challenges in achieving its benefits and mitigating some harms.
- Geräte: PC
- mit Kopierschutz
- eBook Hilfe
- Größe: 3.45MB
- FamilySharing(5)
Andere Kunden interessierten sich auch für
- Valliappa LakshmananData Science on the Google Cloud Platform (eBook, PDF)43,95 €
- Jeroen JanssensData Science at the Command Line (eBook, PDF)36,95 €
- Ted DunningSharing Big Data Safely (eBook, PDF)14,95 €
- Ole Olesen-BagneuxEnterprise Data Catalog (eBook, PDF)36,95 €
- Shannon BradshawMongoDB: The Definitive Guide (eBook, PDF)36,95 €
- Vinicius M. GrippaLearning MySQL (eBook, PDF)36,95 €
- Tony HammondExploring Graphs with Elixir (eBook, PDF)29,95 €
-
-
-
Four leading experts convey the promise of data science and examine challenges in achieving its benefits and mitigating some harms.
Dieser Download kann aus rechtlichen Gründen nur mit Rechnungsadresse in A, B, BG, CY, CZ, D, DK, EW, E, FIN, F, GR, HR, H, IRL, I, LT, L, LR, M, NL, PL, P, R, S, SLO, SK ausgeliefert werden.
Produktdetails
- Produktdetails
- Verlag: Cambridge University Press
- Erscheinungstermin: 20. Oktober 2022
- Englisch
- ISBN-13: 9781009272216
- Artikelnr.: 70911401
- Verlag: Cambridge University Press
- Erscheinungstermin: 20. Oktober 2022
- Englisch
- ISBN-13: 9781009272216
- Artikelnr.: 70911401
- Herstellerkennzeichnung Die Herstellerinformationen sind derzeit nicht verfügbar.
Alfred Z. Spector is a technologist and research leader. His career has led him from innovation in large scale, networked computing systems (at Stanford, CMU, and his company, Transarc) to broad research leadership: first leading IBM Software Research and then Google Research. Following Google, he was the CTO at Two Sigma Investments, and he is presently a Visiting Scholar at MIT. In addition to his managerial career, Dr. Spector lectured widely on the growing importance of computer science across all disciplines (CS+X) and on the Societal Implications of Data Science. He is a fellow of the ACM, IEEE, and the American Academy of Arts and Sciences, and a member of the National Academy of Engineering. Dr. Spector won the 2001 IEEE Kanai Award for Distributed Computing, was co-awarded the 2016 ACM Software Systems Award, and was a Phi Beta Kappa Visiting Scholar. He received a Ph.D. in Computer Science from Stanford and an A.B. in Applied Mathematics from Harvard.
Introduction
Part I. Data Science: 1. Foundations of data science
2. Data science is transdisciplinary
3. A framework for ethical considerations
Recap of Part I - Data Science
Part II. Applying Data Science: 4. Data science applications: six examples
5. The analysis rubric
6. Applying the analysis rubric
7. A principlist approach to ethical considerations
Recap of Part II - Transitioning from Examples and Learnings to Challenges
Part III. Challenges in Applying Data Science: 8. Tractable data
9. Building and deploying models
10. Dependability
11. Understandability
12. Setting the right objectives
13. Toleration of failures
14. Ethical, legal, and societal challenges
Recap of Part III - Challenges in Applying Data Science
Part IV. Addressing Concerns: 15. Societal concerns
16. Education and intelligent discourse
17. Regulation
18. Research and development
19. Quality and ethical governance
Recap of Part IV - Addressing Concerns: 20. Concluding thoughts
Appendix. Summary of recommendations from Part IV
About the authors
References
Index.
Part I. Data Science: 1. Foundations of data science
2. Data science is transdisciplinary
3. A framework for ethical considerations
Recap of Part I - Data Science
Part II. Applying Data Science: 4. Data science applications: six examples
5. The analysis rubric
6. Applying the analysis rubric
7. A principlist approach to ethical considerations
Recap of Part II - Transitioning from Examples and Learnings to Challenges
Part III. Challenges in Applying Data Science: 8. Tractable data
9. Building and deploying models
10. Dependability
11. Understandability
12. Setting the right objectives
13. Toleration of failures
14. Ethical, legal, and societal challenges
Recap of Part III - Challenges in Applying Data Science
Part IV. Addressing Concerns: 15. Societal concerns
16. Education and intelligent discourse
17. Regulation
18. Research and development
19. Quality and ethical governance
Recap of Part IV - Addressing Concerns: 20. Concluding thoughts
Appendix. Summary of recommendations from Part IV
About the authors
References
Index.
Introduction
Part I. Data Science: 1. Foundations of data science
2. Data science is transdisciplinary
3. A framework for ethical considerations
Recap of Part I - Data Science
Part II. Applying Data Science: 4. Data science applications: six examples
5. The analysis rubric
6. Applying the analysis rubric
7. A principlist approach to ethical considerations
Recap of Part II - Transitioning from Examples and Learnings to Challenges
Part III. Challenges in Applying Data Science: 8. Tractable data
9. Building and deploying models
10. Dependability
11. Understandability
12. Setting the right objectives
13. Toleration of failures
14. Ethical, legal, and societal challenges
Recap of Part III - Challenges in Applying Data Science
Part IV. Addressing Concerns: 15. Societal concerns
16. Education and intelligent discourse
17. Regulation
18. Research and development
19. Quality and ethical governance
Recap of Part IV - Addressing Concerns: 20. Concluding thoughts
Appendix. Summary of recommendations from Part IV
About the authors
References
Index.
Part I. Data Science: 1. Foundations of data science
2. Data science is transdisciplinary
3. A framework for ethical considerations
Recap of Part I - Data Science
Part II. Applying Data Science: 4. Data science applications: six examples
5. The analysis rubric
6. Applying the analysis rubric
7. A principlist approach to ethical considerations
Recap of Part II - Transitioning from Examples and Learnings to Challenges
Part III. Challenges in Applying Data Science: 8. Tractable data
9. Building and deploying models
10. Dependability
11. Understandability
12. Setting the right objectives
13. Toleration of failures
14. Ethical, legal, and societal challenges
Recap of Part III - Challenges in Applying Data Science
Part IV. Addressing Concerns: 15. Societal concerns
16. Education and intelligent discourse
17. Regulation
18. Research and development
19. Quality and ethical governance
Recap of Part IV - Addressing Concerns: 20. Concluding thoughts
Appendix. Summary of recommendations from Part IV
About the authors
References
Index.