Deep Learning for Biomedical Image Reconstruction (eBook, PDF)
Redaktion: Ye, Jong Chul; Unser, Michael; Eldar, Yonina C.
88,95 €
88,95 €
inkl. MwSt.
Sofort per Download lieferbar
44 °P sammeln
88,95 €
Als Download kaufen
88,95 €
inkl. MwSt.
Sofort per Download lieferbar
44 °P sammeln
Jetzt verschenken
Alle Infos zum eBook verschenken
88,95 €
inkl. MwSt.
Sofort per Download lieferbar
Alle Infos zum eBook verschenken
44 °P sammeln
Deep Learning for Biomedical Image Reconstruction (eBook, PDF)
Redaktion: Ye, Jong Chul; Unser, Michael; Eldar, Yonina C.
- Format: PDF
- Merkliste
- Auf die Merkliste
- Bewerten Bewerten
- Teilen
- Produkt teilen
- Produkterinnerung
- Produkterinnerung
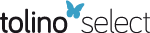
Bitte loggen Sie sich zunächst in Ihr Kundenkonto ein oder registrieren Sie sich bei
bücher.de, um das eBook-Abo tolino select nutzen zu können.
Hier können Sie sich einloggen
Hier können Sie sich einloggen
Sie sind bereits eingeloggt. Klicken Sie auf 2. tolino select Abo, um fortzufahren.
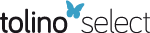
Bitte loggen Sie sich zunächst in Ihr Kundenkonto ein oder registrieren Sie sich bei bücher.de, um das eBook-Abo tolino select nutzen zu können.
- Geräte: PC
- mit Kopierschutz
- eBook Hilfe
- Größe: 20.79MB
- FamilySharing(5)
Andere Kunden interessierten sich auch für
- Machine Learning and Deep Learning Techniques for Medical Image Recognition (eBook, PDF)52,95 €
- Angshul MajumdarCompressed Sensing for Magnetic Resonance Image Reconstruction (eBook, PDF)99,95 €
- GrullAcceleration of Biomedical Image Processing with Dataflow on FPGAs (eBook, PDF)65,95 €
- Deep Learning Applications in Medical Image Segmentation (eBook, PDF)133,99 €
- Gengsheng ZengMedical Image Reconstruction (eBook, PDF)73,95 €
- Diagnosis of Neurological Disorders Based on Deep Learning Techniques (eBook, PDF)59,95 €
- Mark HaidekkerAdvanced Biomedical Image Analysis (eBook, PDF)148,99 €
-
-
-
Produktdetails
- Verlag: Cambridge University Press
- Erscheinungstermin: 12. Oktober 2023
- Englisch
- ISBN-13: 9781009051026
- Artikelnr.: 70908331
Dieser Download kann aus rechtlichen Gründen nur mit Rechnungsadresse in A, B, BG, CY, CZ, D, DK, EW, E, FIN, F, GR, HR, H, IRL, I, LT, L, LR, M, NL, PL, P, R, S, SLO, SK ausgeliefert werden.
- Herstellerkennzeichnung Die Herstellerinformationen sind derzeit nicht verfügbar.
Part I. Theory of Deep Learning for Image Reconstruction Michael Unser: 1.
Formalizing deep neural networks Jong Chul Ye and Sangmin Lee; 2. Geometry
of deep learning Saiprasad Ravishankar, Zhishen Huang, Michael McCann and
Siqi Ye; 3. Model-based reconstruction with learning: from unsupervised to
supervised and beyond Yuelong Li, Or Bar-Shira, Vishal Monga and Yonina C.
Eldar; 4. Deep algorithm unrolling for biomedical; Part II. Deep Learning
Architecture for Various Imaging Modalities Haimiao Zhang, Bin Dong, Ge
Wang and Baodong Liu: 5. Deep learning for CT image reconstruction
Guang-Hong Chen, Chengzhu Zhang, Yinsheng Li, Yoseob Han and Jong Chul Ye;
6. Deep learning in CT reconstruction: bring the measured data to tasks
Patricia Johnson and Florian Knoll; 7. Overview deep learning
reconstruction of accelerated MRI Mathews Jacob, Hemant K. Aggarwal and
Qing Zou; 8. Model-based deep learning algorithms for inverse problems
Mehmet Akcakaya, Gyutaek Oh, Jong Chul Ye; 9. k-space deep learning for MR
reconstruction and artifact removal Ruud J. G. van Sloun, Jong Chul Ye and
Yonina C Eldar; 10. Deep learning for ultrasound beamforming Jaeyoung Huh,
Shujaat Khan and Jong Chul Ye; 11. Ultrasound image artifact removal using
deep neural network; Part III. Generative Models for Biomedical Imaging
Jaejun Yoo, Michael Unser: 12. Deep generative models for biomedical image
reconstruction Tolga C¿ukur, Mahmut Yurt, Salman Ul Hassan Dar, Hyungjin
Chun and, Jong Chul Ye; 13. Image synthesis in multi-contrast MRI with
generative adversarial networks Jaejun Yoo and Michael Unser; 14.
Regularizing deep-neural-network paradigm for the reconstruction of dynamic
magnetic resonance images Thanh-an Pham, Fangshu Yang and Michael Unser;
15. Regularizing neural network for phase unwrapping Michael T. McCann,
Laur`ene Donati, Harshit Gupta and Michael Unser; 16. CryoGAN: a deep
generative adversarial approach to single-particle cryo-em; Index.
Formalizing deep neural networks Jong Chul Ye and Sangmin Lee; 2. Geometry
of deep learning Saiprasad Ravishankar, Zhishen Huang, Michael McCann and
Siqi Ye; 3. Model-based reconstruction with learning: from unsupervised to
supervised and beyond Yuelong Li, Or Bar-Shira, Vishal Monga and Yonina C.
Eldar; 4. Deep algorithm unrolling for biomedical; Part II. Deep Learning
Architecture for Various Imaging Modalities Haimiao Zhang, Bin Dong, Ge
Wang and Baodong Liu: 5. Deep learning for CT image reconstruction
Guang-Hong Chen, Chengzhu Zhang, Yinsheng Li, Yoseob Han and Jong Chul Ye;
6. Deep learning in CT reconstruction: bring the measured data to tasks
Patricia Johnson and Florian Knoll; 7. Overview deep learning
reconstruction of accelerated MRI Mathews Jacob, Hemant K. Aggarwal and
Qing Zou; 8. Model-based deep learning algorithms for inverse problems
Mehmet Akcakaya, Gyutaek Oh, Jong Chul Ye; 9. k-space deep learning for MR
reconstruction and artifact removal Ruud J. G. van Sloun, Jong Chul Ye and
Yonina C Eldar; 10. Deep learning for ultrasound beamforming Jaeyoung Huh,
Shujaat Khan and Jong Chul Ye; 11. Ultrasound image artifact removal using
deep neural network; Part III. Generative Models for Biomedical Imaging
Jaejun Yoo, Michael Unser: 12. Deep generative models for biomedical image
reconstruction Tolga C¿ukur, Mahmut Yurt, Salman Ul Hassan Dar, Hyungjin
Chun and, Jong Chul Ye; 13. Image synthesis in multi-contrast MRI with
generative adversarial networks Jaejun Yoo and Michael Unser; 14.
Regularizing deep-neural-network paradigm for the reconstruction of dynamic
magnetic resonance images Thanh-an Pham, Fangshu Yang and Michael Unser;
15. Regularizing neural network for phase unwrapping Michael T. McCann,
Laur`ene Donati, Harshit Gupta and Michael Unser; 16. CryoGAN: a deep
generative adversarial approach to single-particle cryo-em; Index.
Part I. Theory of Deep Learning for Image Reconstruction Michael Unser: 1.
Formalizing deep neural networks Jong Chul Ye and Sangmin Lee; 2. Geometry
of deep learning Saiprasad Ravishankar, Zhishen Huang, Michael McCann and
Siqi Ye; 3. Model-based reconstruction with learning: from unsupervised to
supervised and beyond Yuelong Li, Or Bar-Shira, Vishal Monga and Yonina C.
Eldar; 4. Deep algorithm unrolling for biomedical; Part II. Deep Learning
Architecture for Various Imaging Modalities Haimiao Zhang, Bin Dong, Ge
Wang and Baodong Liu: 5. Deep learning for CT image reconstruction
Guang-Hong Chen, Chengzhu Zhang, Yinsheng Li, Yoseob Han and Jong Chul Ye;
6. Deep learning in CT reconstruction: bring the measured data to tasks
Patricia Johnson and Florian Knoll; 7. Overview deep learning
reconstruction of accelerated MRI Mathews Jacob, Hemant K. Aggarwal and
Qing Zou; 8. Model-based deep learning algorithms for inverse problems
Mehmet Akcakaya, Gyutaek Oh, Jong Chul Ye; 9. k-space deep learning for MR
reconstruction and artifact removal Ruud J. G. van Sloun, Jong Chul Ye and
Yonina C Eldar; 10. Deep learning for ultrasound beamforming Jaeyoung Huh,
Shujaat Khan and Jong Chul Ye; 11. Ultrasound image artifact removal using
deep neural network; Part III. Generative Models for Biomedical Imaging
Jaejun Yoo, Michael Unser: 12. Deep generative models for biomedical image
reconstruction Tolga C¿ukur, Mahmut Yurt, Salman Ul Hassan Dar, Hyungjin
Chun and, Jong Chul Ye; 13. Image synthesis in multi-contrast MRI with
generative adversarial networks Jaejun Yoo and Michael Unser; 14.
Regularizing deep-neural-network paradigm for the reconstruction of dynamic
magnetic resonance images Thanh-an Pham, Fangshu Yang and Michael Unser;
15. Regularizing neural network for phase unwrapping Michael T. McCann,
Laur`ene Donati, Harshit Gupta and Michael Unser; 16. CryoGAN: a deep
generative adversarial approach to single-particle cryo-em; Index.
Formalizing deep neural networks Jong Chul Ye and Sangmin Lee; 2. Geometry
of deep learning Saiprasad Ravishankar, Zhishen Huang, Michael McCann and
Siqi Ye; 3. Model-based reconstruction with learning: from unsupervised to
supervised and beyond Yuelong Li, Or Bar-Shira, Vishal Monga and Yonina C.
Eldar; 4. Deep algorithm unrolling for biomedical; Part II. Deep Learning
Architecture for Various Imaging Modalities Haimiao Zhang, Bin Dong, Ge
Wang and Baodong Liu: 5. Deep learning for CT image reconstruction
Guang-Hong Chen, Chengzhu Zhang, Yinsheng Li, Yoseob Han and Jong Chul Ye;
6. Deep learning in CT reconstruction: bring the measured data to tasks
Patricia Johnson and Florian Knoll; 7. Overview deep learning
reconstruction of accelerated MRI Mathews Jacob, Hemant K. Aggarwal and
Qing Zou; 8. Model-based deep learning algorithms for inverse problems
Mehmet Akcakaya, Gyutaek Oh, Jong Chul Ye; 9. k-space deep learning for MR
reconstruction and artifact removal Ruud J. G. van Sloun, Jong Chul Ye and
Yonina C Eldar; 10. Deep learning for ultrasound beamforming Jaeyoung Huh,
Shujaat Khan and Jong Chul Ye; 11. Ultrasound image artifact removal using
deep neural network; Part III. Generative Models for Biomedical Imaging
Jaejun Yoo, Michael Unser: 12. Deep generative models for biomedical image
reconstruction Tolga C¿ukur, Mahmut Yurt, Salman Ul Hassan Dar, Hyungjin
Chun and, Jong Chul Ye; 13. Image synthesis in multi-contrast MRI with
generative adversarial networks Jaejun Yoo and Michael Unser; 14.
Regularizing deep-neural-network paradigm for the reconstruction of dynamic
magnetic resonance images Thanh-an Pham, Fangshu Yang and Michael Unser;
15. Regularizing neural network for phase unwrapping Michael T. McCann,
Laur`ene Donati, Harshit Gupta and Michael Unser; 16. CryoGAN: a deep
generative adversarial approach to single-particle cryo-em; Index.