Deep Learning in Diabetes Mellitus Detection and Diagnosis (eBook, ePUB)
Redaktion: Chaki, Jyotismita; Wozniak, Marcin
52,95 €
52,95 €
inkl. MwSt.
Erscheint vor. 30.01.25
26 °P sammeln
52,95 €
Als Download kaufen
52,95 €
inkl. MwSt.
Erscheint vor. 30.01.25
26 °P sammeln
Jetzt verschenken
Alle Infos zum eBook verschenken
52,95 €
inkl. MwSt.
Erscheint vor. 30.01.25
Alle Infos zum eBook verschenken
26 °P sammeln
Unser Service für Vorbesteller - Ihr Vorteil ohne Risiko:
Sollten wir den Preis dieses Artikels vor dem Erscheinungsdatum senken, werden wir Ihnen den Artikel bei der Auslieferung automatisch zum günstigeren Preis berechnen.
Sollten wir den Preis dieses Artikels vor dem Erscheinungsdatum senken, werden wir Ihnen den Artikel bei der Auslieferung automatisch zum günstigeren Preis berechnen.
Deep Learning in Diabetes Mellitus Detection and Diagnosis (eBook, ePUB)
Redaktion: Chaki, Jyotismita; Wozniak, Marcin
- Format: ePub
- Merkliste
- Auf die Merkliste
- Bewerten Bewerten
- Teilen
- Produkt teilen
- Produkterinnerung
- Produkterinnerung
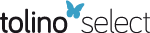
Bitte loggen Sie sich zunächst in Ihr Kundenkonto ein oder registrieren Sie sich bei
bücher.de, um das eBook-Abo tolino select nutzen zu können.
Hier können Sie sich einloggen
Hier können Sie sich einloggen
Sie sind bereits eingeloggt. Klicken Sie auf 2. tolino select Abo, um fortzufahren.
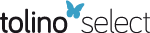
Bitte loggen Sie sich zunächst in Ihr Kundenkonto ein oder registrieren Sie sich bei bücher.de, um das eBook-Abo tolino select nutzen zu können.
This book focuses on deep learning-based approaches in the field of Diabetes Mellitus detection and diagnosis, including preprocessing techniques which are an essential part of this subject. This is the first book of its kind to cover deep learning-based approaches in the specific field of Diabetes Mellitus.
- Geräte: eReader
- mit Kopierschutz
- eBook Hilfe
Andere Kunden interessierten sich auch für
- Deep Learning in Diabetes Mellitus Detection and Diagnosis (eBook, PDF)52,95 €
- Louis J. CataniaAI for Immunology (eBook, ePUB)24,95 €
- Hagai MeirovitchEntropy and Free Energy in Structural Biology (eBook, ePUB)48,95 €
- Qing YanTranslational Bioinformatics and Systems Biology Methods for Personalized Medicine (eBook, ePUB)81,95 €
- Uri AlonSystems Medicine (eBook, ePUB)57,95 €
- Artificial Intelligence in Bioinformatics and Chemoinformatics (eBook, ePUB)136,95 €
- Recent Advances in AI-enabled Automated Medical Diagnosis (eBook, ePUB)54,95 €
-
-
-
This book focuses on deep learning-based approaches in the field of Diabetes Mellitus detection and diagnosis, including preprocessing techniques which are an essential part of this subject. This is the first book of its kind to cover deep learning-based approaches in the specific field of Diabetes Mellitus.
Dieser Download kann aus rechtlichen Gründen nur mit Rechnungsadresse in A, B, BG, CY, CZ, D, DK, EW, E, FIN, F, GR, HR, H, IRL, I, LT, L, LR, M, NL, PL, P, R, S, SLO, SK ausgeliefert werden.
Produktdetails
- Produktdetails
- Verlag: Taylor & Francis
- Erscheinungstermin: 30. Januar 2025
- Englisch
- ISBN-13: 9781040260654
- Artikelnr.: 72284189
- Verlag: Taylor & Francis
- Erscheinungstermin: 30. Januar 2025
- Englisch
- ISBN-13: 9781040260654
- Artikelnr.: 72284189
- Herstellerkennzeichnung Die Herstellerinformationen sind derzeit nicht verfügbar.
Dr. Jyotismita Chaki is an Associate Professor at the School of Computer Science and Engineering, Vellore Institute of Technology, India. Holding a PhD in Engineering from Jadavpur University, Kolkata, her research focuses on Computer Vision, Image Processing, Pattern Recognition, Medical Imaging, Artificial Intelligence, and Machine Learning. With a prolific publication record, Dr. Chaki has authored over 50 international conference and journal papers and contributed to more than ten books as author or editor. Her editorial roles currently include Editor of Engineering Applications of Artificial Intelligence (Elsevier), Section Editor of PeerJ Computer Science, and Associate Editor for Computer and Electrical Engineering, Array, and Machine Learning with Applications journals (Elsevier). She is a Senior Member of IEEE. Marcin Wozniak received the M.Sc. degree in applied mathematics, the Ph.D. degree in computational intelligence, the D.Sc. degree in computational intelligence and Full Professor honours from the President of Poland. M. Wozniak is currently a Full Professor with the Faculty of Applied Mathematics, Silesian University of Technology. He is a Scientific Supervisor in editions of "The Diamond Grant" and "The Best of the Best" programs for highly talented students from the Polish Ministry of Science and Higher Education. He participated in various scientific projects (as Lead Investigator, Scientific Investigator, Manager, Participant and Advisor) at Polish, Italian and Lithuanian universities and projects with applied results at IT industry both funded from the National Centre for Research and Development and abroad. He was a Visiting Researcher with universities in Italy, Sweden, and Germany. He has authored/coauthored over 300 research papers in international conferences and journals. His current research interests include neural networks with their applications together with various aspects of fuzzy logic and control, applied computational intelligence accelerated by evolutionary computation and federated learning models. In 2017 Prof Marcin Wozniak was awarded by the Polish Ministry of Science and Higher Education with a scholarship for an outstanding young scientist. In years 2021 and 2024 he received two awards from the Polish Ministry of Science and Higher Education for research achievements. In 2020, 2021, 2022 and 2023 Prof Marcin Wozniak was presented among "TOP 2% Scientists in the World" by Stanford University for his career achievements. Prof Marcin Wozniak is also presented among the Best Computer Science Scientists in Poland by Research.com. Marcin Wozniak is the Editorial Board member or an Editor for Biomedical Signal Processing and Control, Sensors, Machine Learning with Applications, Pattern Analysis and Applications. He also guest edit special issues ie. IEEE Journal Biomedical and Health Informatics, IEEE ACCESS, Measurement, Sustainable Energy Technologies and Assessments, Frontiers in Human Neuroscience, PeerJ CS, International Journal of Distributed Sensor Networks, Computational Intelligence and Neuroscience, Journal of Universal Computer Science, etc. Prof. Marcin Wozniak is a Session Chair at various international conferences and symposiums, including IEEE Symposium Series on Computational Intelligence, IEEE Congress on Evolutionary Computation, International Joint Conference on Neural Networks, etc.
1. Introduction to Diabetes Mellitus Detection and Diagnosis using deep
Learning. 2. Pre-processing and Detection of Diabetes Mellitus from
physiological data using deep learning. 3. Graph-based Explainable Method
for Blood Glucose Prediction through Federated Learning. 4. Automated Early
detection of Diabetes Mellitus from Retinal Fundus images using Residual
U-Network Approach. 5. Towards Classifying the Severity of Diabetic
Retinopathy Using Deep Learning. 6. Deep Learning saves lives of diabetes
mellitus patients and cuts treatment costs. 7. Diabetes mellitus detection
using deep learning model. 8. A Comprehensive Review of the Use of Deep
Learning Algorithms in Diabetes Mellitus Detection and Diagnosis. 9.
Examining the Role of Machine Learning and Deep Learning in Diabetes
Mellitus Detection and Diagnosis - A Critical Review. 10. Deep Learning in
Diabetes Mellitus Detection and Diagnosis. 11. Title: Deep Learning
Algorithms for Diabetes Mellitus Detection and Management. 12. An Analysis
of Deep Learning Models for Diabetic Retinopathy Detection and
Classification Based on Fundus Image.
Learning. 2. Pre-processing and Detection of Diabetes Mellitus from
physiological data using deep learning. 3. Graph-based Explainable Method
for Blood Glucose Prediction through Federated Learning. 4. Automated Early
detection of Diabetes Mellitus from Retinal Fundus images using Residual
U-Network Approach. 5. Towards Classifying the Severity of Diabetic
Retinopathy Using Deep Learning. 6. Deep Learning saves lives of diabetes
mellitus patients and cuts treatment costs. 7. Diabetes mellitus detection
using deep learning model. 8. A Comprehensive Review of the Use of Deep
Learning Algorithms in Diabetes Mellitus Detection and Diagnosis. 9.
Examining the Role of Machine Learning and Deep Learning in Diabetes
Mellitus Detection and Diagnosis - A Critical Review. 10. Deep Learning in
Diabetes Mellitus Detection and Diagnosis. 11. Title: Deep Learning
Algorithms for Diabetes Mellitus Detection and Management. 12. An Analysis
of Deep Learning Models for Diabetic Retinopathy Detection and
Classification Based on Fundus Image.
1. Introduction to Diabetes Mellitus Detection and Diagnosis using deep
Learning. 2. Pre-processing and Detection of Diabetes Mellitus from
physiological data using deep learning. 3. Graph-based Explainable Method
for Blood Glucose Prediction through Federated Learning. 4. Automated Early
detection of Diabetes Mellitus from Retinal Fundus images using Residual
U-Network Approach. 5. Towards Classifying the Severity of Diabetic
Retinopathy Using Deep Learning. 6. Deep Learning saves lives of diabetes
mellitus patients and cuts treatment costs. 7. Diabetes mellitus detection
using deep learning model. 8. A Comprehensive Review of the Use of Deep
Learning Algorithms in Diabetes Mellitus Detection and Diagnosis. 9.
Examining the Role of Machine Learning and Deep Learning in Diabetes
Mellitus Detection and Diagnosis - A Critical Review. 10. Deep Learning in
Diabetes Mellitus Detection and Diagnosis. 11. Title: Deep Learning
Algorithms for Diabetes Mellitus Detection and Management. 12. An Analysis
of Deep Learning Models for Diabetic Retinopathy Detection and
Classification Based on Fundus Image.
Learning. 2. Pre-processing and Detection of Diabetes Mellitus from
physiological data using deep learning. 3. Graph-based Explainable Method
for Blood Glucose Prediction through Federated Learning. 4. Automated Early
detection of Diabetes Mellitus from Retinal Fundus images using Residual
U-Network Approach. 5. Towards Classifying the Severity of Diabetic
Retinopathy Using Deep Learning. 6. Deep Learning saves lives of diabetes
mellitus patients and cuts treatment costs. 7. Diabetes mellitus detection
using deep learning model. 8. A Comprehensive Review of the Use of Deep
Learning Algorithms in Diabetes Mellitus Detection and Diagnosis. 9.
Examining the Role of Machine Learning and Deep Learning in Diabetes
Mellitus Detection and Diagnosis - A Critical Review. 10. Deep Learning in
Diabetes Mellitus Detection and Diagnosis. 11. Title: Deep Learning
Algorithms for Diabetes Mellitus Detection and Management. 12. An Analysis
of Deep Learning Models for Diabetic Retinopathy Detection and
Classification Based on Fundus Image.