Digital Signal Processing with Kernel Methods (eBook, PDF)
Alle Infos zum eBook verschenken
Digital Signal Processing with Kernel Methods (eBook, PDF)
- Format: PDF
- Merkliste
- Auf die Merkliste
- Bewerten Bewerten
- Teilen
- Produkt teilen
- Produkterinnerung
- Produkterinnerung
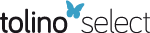
Hier können Sie sich einloggen
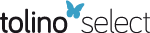
Bitte loggen Sie sich zunächst in Ihr Kundenkonto ein oder registrieren Sie sich bei bücher.de, um das eBook-Abo tolino select nutzen zu können.
A realistic and comprehensive review of joint approaches to machine learning and signal processing algorithms, with application to communications, multimedia, and biomedical engineering systems Digital Signal Processing with Kernel Methods reviews the milestones in the mixing of classical digital signal processing models and advanced kernel machines statistical learning tools. It explains the fundamental concepts from both fields of machine learning and signal processing so that readers can quickly get up to speed in order to begin developing the concepts and application software in their own…mehr
- Geräte: PC
- mit Kopierschutz
- eBook Hilfe
- Größe: 29.6MB
- Kernel Methods for Remote Sensing Data Analysis (eBook, PDF)112,99 €
- Maurice CharbitDigital Signal Processing (DSP) with Python Programming (eBook, PDF)139,99 €
- Streamlining Digital Signal Processing (eBook, PDF)80,99 €
- Weifeng LiuKernel Adaptive Filtering (eBook, PDF)107,99 €
- Maurice BellangerDigital Signal Processing (eBook, PDF)122,99 €
- Gérard BlanchetDigital Signal and Image Processing using MATLAB, Volume 2 (eBook, PDF)139,99 €
- Shoab Ahmed KhanDigital Design of Signal Processing Systems (eBook, PDF)107,99 €
-
-
-
Dieser Download kann aus rechtlichen Gründen nur mit Rechnungsadresse in A, B, BG, CY, CZ, D, DK, EW, E, FIN, F, GR, HR, H, IRL, I, LT, L, LR, M, NL, PL, P, R, S, SLO, SK ausgeliefert werden.
- Produktdetails
- Verlag: Wiley-Blackwell
- Seitenzahl: 672
- Erscheinungstermin: 22. Dezember 2017
- Englisch
- ISBN-13: 9781118705827
- Artikelnr.: 52553495
- Verlag: Wiley-Blackwell
- Seitenzahl: 672
- Erscheinungstermin: 22. Dezember 2017
- Englisch
- ISBN-13: 9781118705827
- Artikelnr.: 52553495
- Herstellerkennzeichnung Die Herstellerinformationen sind derzeit nicht verfügbar.
nite Kernels 169 4.2.3 Reproducing Kernel in Hilbert Space and Reproducing Property 170 4.2.4 Mercer's Theorem 173 4.3 Kernel Properties 174 4.3.1 Tikhonov's Regularization 175 4.3.2 Representer Theorem and Regularization Properties 176 4.3.3 Basic Operations with Kernels 178 4.4 Constructing Kernel Functions 179 4.4.1 Standard Kernels 179 4.4.2 Properties of Kernels 180 4.4.3 Engineering Signal Processing Kernels 181 4.5 Complex Reproducing Kernel in Hilbert Spaces 184 4.6 Support Vector Machine Elements for Regression and Estimation 186 4.6.1 Support Vector Regression Signal Model and Cost Function 186 4.6.2 Minimizing Functional 187 4.7 Tutorials and Application Examples 191 4.7.1 Kernel Calculations and Kernel Matrices 191 4.7.2 Basic Operations with Kernels 194 4.7.3 Constructing Kernels 197 4.7.4 Complex Kernels 199 4.7.5 Application Example for Support Vector Regression Elements 202 4.8 Concluding Remarks 205 4.9 Questions and Problems 205 Part II Function Approximation and Adaptive Filtering 209 5 A Support Vector Machine Signal Estimation Framework 211 5.1 Introduction 211 5.2 A Framework for Support Vector Machine Signal Estimation 213 5.3 Primal Signal Models for Support Vector Machine Signal Processing 216 5.3.1 Nonparametric Spectrum and System Identification 218 5.3.2 Orthogonal Frequency Division Multiplexing Digital Communications 220 5.3.3 Convolutional Signal Models 222 5.3.4 Array Processing 225 5.4 Tutorials and Application Examples 227 5.4.1 Nonparametric Spectral Analysis with Primal Signal Models 227 5.4.2 System Identification with Primal Signal Model ;;-
lter 228 5.4.3 Parametric Spectral Density Estimation with Primal Signal Models 230 5.4.4 Temporal Reference Array Processing with Primal Signal Models 231 5.4.5 Sinc Interpolation with Primal Signal Models 233 6 Reproducing Kernel Hilbert Space Models for Signal Processing 241 6.1 Introduction 241 6.2 Reproducing Kernel Hilbert Space Signal Models 242 6.2.1 Kernel Autoregressive Exogenous Identi
cation 244 6.2.2 Kernel Finite Impulse Response and the ;;-Filter 247 6.2.3 Kernel Array Processing with Spatial Reference 248 6.2.4 Kernel Semiparametric Regression 249 6.3 Tutorials and Application Examples 258 6.3.1 Nonlinear System Identification with Support Vector Machine-Autoregressive and Moving Average 258 6.3.2 Nonlinear System Identi
cation with the ;;-
lter 260 6.3.3 Electric Network Modeling with Semiparametric Regression 264 6.3.4 Promotional Data 272 6.3.5 Spatial and Temporal Antenna Array Kernel Processing 275 6.4 Questions and Problems 279 7 Dual Signal Models for Signal Processing 281 7.1 Introduction 281 7.2 Dual Signal Model Elements 281 7.3 Dual Signal Model Instantiations 283 7.3.1 Dual Signal Model for Nonuniform Signal Interpolation 283 7.3.2 Dual Signal Model for Sparse Signal Deconvolution 284 7.3.3 Spectrally Adapted Mercer Kernels 285 7.4 Tutorials and Application Examples 289 7.4.1 Nonuniform Interpolation with the Dual Signal Model 290 7.4.2 Sparse Deconvolution with the Dual Signal Model 292 7.4.3 Doppler Ultrasound Processing for Fault Detection 294 7.4.4 Spectrally Adapted Mercer Kernels 296 7.4.5 Interpolation of Heart Rate Variability Signals 304 7.4.6 Denoising in Cardiac Motion-Mode Doppler Ultrasound Images 309?m 7.4.7 Indoor Location from Mobile Devices Measurements 316 7.4.8 Electroanatomical Maps in Cardiac Navigation Systems 322 7.5 Questions and Problems 331 8 Advances in Kernel Regression and Function Approximation 333 8.1 Introduction 333 8.2 Kernel-Based Regression Methods 333 8.2.1 Advances in Support Vector Regression 334 8.2.2 Multi-output Support Vector Regression 338 8.2.3 Kernel Ridge Regression 339 8.2.4 Kernel Signal-To-Noise Regression 341 8.2.5 Semisupervised Support Vector Regression 343 8.2.6 Model Selection in Kernel Regression Methods 345 8.4.1 Comparing Support Vector Regression, Relevance Vector Machines, and Gaussian Process Regression 360 8.4.2 Pröle-Dependent Support Vector Regression 362 8.4.3 Multi-output Support Vector Regression 364 8.4.4 Kernel Signal-to-Noise Ratio Regression 366 8.4.5 Semisupervised Support Vector Regression 368 8.4.6 Bayesian Nonparametric Model 369 8.4.7 Gaussian Process Regression 370 8.4.8 Relevance Vector Machines 379 8.5 Concluding Remarks 382 8.6 Questions and Problems 383 9 Adaptive Kernel Learning for Signal Processing 387 9.1 Introduction 387 9.2 Linear Adaptive Filtering 387 9.2.1 Least Mean Squares Algorithm 388 9.2.2 Recursive Least-Squares Algorithm 389 9.3 Kernel Adaptive Filtering 392 9.4 Kernel Least Mean Squares 392 9.4.1 Derivation of Kernel Least Mean Squares 393 9.4.2 Implementation Challenges and Dual Formulation 394 9.5.3 Prediction of the Mackey-Glass Time Series with Kernel Recursive Least Squares 401 9.5.4 Beyond the Stationary Model 402 9.5.5 Example on Nonlinear Channel Identi
cation and Reconvergence 405 9.6 Explicit Recursivity for Adaptive Kernel Models 406 9.6.1 Recursivity in Hilbert Spaces 406 9.6.2 Recursive Filters in Reproducing Kernel Hilbert Spaces 408 9.7 Online Sparsi
cation with Kernels 411 9.7.1 Sparsity by Construction 411 9.7.2 Sparsity by Pruning 413 9.8 Probabilistic Approaches to Kernel Adaptive Filtering 414 9.8.1 Gaussian Processes and Kernel Ridge Regression 415 9.8.2 Online Recursive Solution for Gaussian Processes Regression 416 9.8.3 Kernel Recursive Least Squares Tracker 417 9.8.4 Probabilistic Kernel Least Mean Squares 418 9.9 Further Reading 418 9.9.1 Selection of Kernel Parameters 418 9.9.2 Multi-Kernel Adaptive Filtering 419 9.9.3 Recursive Filtering in Kernel Hilbert Spaces 419 9.10 Tutorials and Application Examples 419 9.10.1 Kernel Adaptive Filtering Toolbox 420 9.10.2 Prediction of a Respiratory Motion Time Series 421 9.10.3 Online Regression on the KIN?h?eK Dataset 423 9.10.4 The Mackey-Glass Time Series 425 9.10.5 Explicit Recursivity on Reproducing Kernel in Hilbert Space and Electroencephalogram Prediction 427 9.10.6 Adaptive Antenna Array Processing 428 9.11 Questions and Problems 430 Part III Classification, Detection, and Feature Extraction 433 10 Support Vector Machine and Kernel Classification Algorithms 435 10.1 Introduction 435 10.2 Support Vector Machine and Kernel Classi
ers 435 10.2.1 Support Vector Machines 435 10.2.2 Multiclass and Multilabel Support Vector Machines 441 10.2.3 Least-Squares Support Vector Machine 447 10.2.4 Kernel Fisher's Discriminant Analysis 448 10.3 Advances in Kernel-Based Classi
cation 452 10.3.1 Large Margin Filtering 452 10.3.2 Semisupervised Learning 454 10.3.3 Multiple Kernel Learning 460 10.3.4 Structured-Output Learning 462 10.3.5 Active Learning 468 10.4 Large-Scale Support Vector Machines 477 10.4.1 Large-Scale Support Vector Machine Implementations 477 10.4.2 Random Fourier Features 478 10.4.3 Parallel Support Vector Machine 480 10.4.4 Outlook 483 10.5 Tutorials and Application Examples 485 10.5.1 Examples of Support Vector Machine Classi
cation 485 10.5.2 Example of Least-Squares Support Vector Machine 492 10.5.3 Kernel-Filtering Support Vector Machine for Brain-Computer Interface Signal Classi
cation 493 10.5.4 Example of Laplacian Support Vector Machine 494 10.5.5 Example of Graph-Based Label Propagation 498 10.5.6 Examples of Multiple Kernel Learning 498 10.6 Concluding Remarks 501 10.7 Questions and Problems 502 11 Clustering and Anomaly Detection with Kernels 503 11.1 Introduction 503 11.2 Kernel Clustering 506 11.2.1 Kernelization of the Metric 506 11.2.2 Clustering in Feature Spaces 508 11.3 Domain Description Via Support Vectors 514 11.3.1 Support Vector Domain Description 514 11.3.2 One-Class Support Vector Machine 515 11.3.3 Relationship Between Support Vector Domain Description and Density Estimation 516 11.3.4 Semisupervised One-Class Classi
cation 517 11.4 Kernel Matched Subspace Detectors 518 11.4.1 Kernel Orthogonal Subspace Projection 518 11.4.2 Kernel Spectral Angle Mapper 520 11.5 Kernel Anomaly Change Detection 522 11.5.1 Linear Anomaly Change Detection Algorithms 522 11.5.2 Kernel Anomaly Change Detection Algorithms 523 11.6 Hypothesis Testing with Kernels 525 11.6.1 Distribution Embeddings 526 11.6.3 Maximum Mean Discrepancy 527 11.6.3 One-Class Support Measure Machine 528 11.7 Tutorials and Application Examples 529 11.7.1 Example on Kernelization of the Metric 529 11.7.2 Example on Kernel k-Means 530 11.7.3 Domain Description Examples 531 11.7.4 Kernel Spectral Angle Mapper and Kernel Orthogonal Subspace Projection Examples 534 11.7.5 Example of Kernel Anomaly Change Detection Algorithms 536 11.7.6 Example on Distribution Embeddings and Maximum Mean Discrepancy 540 11.8 Concluding Remarks 541 11.9 Questions and Problems 542 12 Kernel Feature Extraction in Signal Processing 543 12.1 Introduction 543 12.2 Multivariate Analysis in Reproducing Kernel Hilbert Spaces 545 12.2.1 Problem Statement and Notation 545 12.2.2 Linear Multivariate Analysis 546 12.2.3 Kernel Multivariate Analysis 549 12.2.4 Multivariate Analysis Experiments 551 12.3 Feature Extraction with Kernel Dependence Estimates 555 12.3.1 Feature Extraction Using Hilbert-Schmidt Independence Criterion 556 12.3.2 Blind Source Separation Using Kernels 563 12.4 Extensions for Large-Scale and Semisupervised Problems 570 12.4.2 E
ciency with the Incomplete Cholesky Decomposition 570 12.4.3 E
ciency with Random Fourier Features 570 12.4.3 Sparse Kernel Feature Extraction 571 12.4.4 Semisupervised Kernel Feature Extraction 573 12.5 Domain Adaptation with Kernels 575 12.5.1 Kernel Mean Matching 578 12.5.2 Transfer Component Analysis 579 12.5.3 Kernel Manifold Alignment 581 12.5.4 Relations between Domain Adaptation Methods 585 12.5.5 Experimental Comparison between Domain Adaptation Methods 12.6 Concluding Remarks 587 12.7 Questions and Problems 588 References 589 Index 631
nite Kernels 169 4.2.3 Reproducing Kernel in Hilbert Space and Reproducing Property 170 4.2.4 Mercer's Theorem 173 4.3 Kernel Properties 174 4.3.1 Tikhonov's Regularization 175 4.3.2 Representer Theorem and Regularization Properties 176 4.3.3 Basic Operations with Kernels 178 4.4 Constructing Kernel Functions 179 4.4.1 Standard Kernels 179 4.4.2 Properties of Kernels 180 4.4.3 Engineering Signal Processing Kernels 181 4.5 Complex Reproducing Kernel in Hilbert Spaces 184 4.6 Support Vector Machine Elements for Regression and Estimation 186 4.6.1 Support Vector Regression Signal Model and Cost Function 186 4.6.2 Minimizing Functional 187 4.7 Tutorials and Application Examples 191 4.7.1 Kernel Calculations and Kernel Matrices 191 4.7.2 Basic Operations with Kernels 194 4.7.3 Constructing Kernels 197 4.7.4 Complex Kernels 199 4.7.5 Application Example for Support Vector Regression Elements 202 4.8 Concluding Remarks 205 4.9 Questions and Problems 205 Part II Function Approximation and Adaptive Filtering 209 5 A Support Vector Machine Signal Estimation Framework 211 5.1 Introduction 211 5.2 A Framework for Support Vector Machine Signal Estimation 213 5.3 Primal Signal Models for Support Vector Machine Signal Processing 216 5.3.1 Nonparametric Spectrum and System Identification 218 5.3.2 Orthogonal Frequency Division Multiplexing Digital Communications 220 5.3.3 Convolutional Signal Models 222 5.3.4 Array Processing 225 5.4 Tutorials and Application Examples 227 5.4.1 Nonparametric Spectral Analysis with Primal Signal Models 227 5.4.2 System Identification with Primal Signal Model ;;-
lter 228 5.4.3 Parametric Spectral Density Estimation with Primal Signal Models 230 5.4.4 Temporal Reference Array Processing with Primal Signal Models 231 5.4.5 Sinc Interpolation with Primal Signal Models 233 6 Reproducing Kernel Hilbert Space Models for Signal Processing 241 6.1 Introduction 241 6.2 Reproducing Kernel Hilbert Space Signal Models 242 6.2.1 Kernel Autoregressive Exogenous Identi
cation 244 6.2.2 Kernel Finite Impulse Response and the ;;-Filter 247 6.2.3 Kernel Array Processing with Spatial Reference 248 6.2.4 Kernel Semiparametric Regression 249 6.3 Tutorials and Application Examples 258 6.3.1 Nonlinear System Identification with Support Vector Machine-Autoregressive and Moving Average 258 6.3.2 Nonlinear System Identi
cation with the ;;-
lter 260 6.3.3 Electric Network Modeling with Semiparametric Regression 264 6.3.4 Promotional Data 272 6.3.5 Spatial and Temporal Antenna Array Kernel Processing 275 6.4 Questions and Problems 279 7 Dual Signal Models for Signal Processing 281 7.1 Introduction 281 7.2 Dual Signal Model Elements 281 7.3 Dual Signal Model Instantiations 283 7.3.1 Dual Signal Model for Nonuniform Signal Interpolation 283 7.3.2 Dual Signal Model for Sparse Signal Deconvolution 284 7.3.3 Spectrally Adapted Mercer Kernels 285 7.4 Tutorials and Application Examples 289 7.4.1 Nonuniform Interpolation with the Dual Signal Model 290 7.4.2 Sparse Deconvolution with the Dual Signal Model 292 7.4.3 Doppler Ultrasound Processing for Fault Detection 294 7.4.4 Spectrally Adapted Mercer Kernels 296 7.4.5 Interpolation of Heart Rate Variability Signals 304 7.4.6 Denoising in Cardiac Motion-Mode Doppler Ultrasound Images 309?m 7.4.7 Indoor Location from Mobile Devices Measurements 316 7.4.8 Electroanatomical Maps in Cardiac Navigation Systems 322 7.5 Questions and Problems 331 8 Advances in Kernel Regression and Function Approximation 333 8.1 Introduction 333 8.2 Kernel-Based Regression Methods 333 8.2.1 Advances in Support Vector Regression 334 8.2.2 Multi-output Support Vector Regression 338 8.2.3 Kernel Ridge Regression 339 8.2.4 Kernel Signal-To-Noise Regression 341 8.2.5 Semisupervised Support Vector Regression 343 8.2.6 Model Selection in Kernel Regression Methods 345 8.4.1 Comparing Support Vector Regression, Relevance Vector Machines, and Gaussian Process Regression 360 8.4.2 Pröle-Dependent Support Vector Regression 362 8.4.3 Multi-output Support Vector Regression 364 8.4.4 Kernel Signal-to-Noise Ratio Regression 366 8.4.5 Semisupervised Support Vector Regression 368 8.4.6 Bayesian Nonparametric Model 369 8.4.7 Gaussian Process Regression 370 8.4.8 Relevance Vector Machines 379 8.5 Concluding Remarks 382 8.6 Questions and Problems 383 9 Adaptive Kernel Learning for Signal Processing 387 9.1 Introduction 387 9.2 Linear Adaptive Filtering 387 9.2.1 Least Mean Squares Algorithm 388 9.2.2 Recursive Least-Squares Algorithm 389 9.3 Kernel Adaptive Filtering 392 9.4 Kernel Least Mean Squares 392 9.4.1 Derivation of Kernel Least Mean Squares 393 9.4.2 Implementation Challenges and Dual Formulation 394 9.5.3 Prediction of the Mackey-Glass Time Series with Kernel Recursive Least Squares 401 9.5.4 Beyond the Stationary Model 402 9.5.5 Example on Nonlinear Channel Identi
cation and Reconvergence 405 9.6 Explicit Recursivity for Adaptive Kernel Models 406 9.6.1 Recursivity in Hilbert Spaces 406 9.6.2 Recursive Filters in Reproducing Kernel Hilbert Spaces 408 9.7 Online Sparsi
cation with Kernels 411 9.7.1 Sparsity by Construction 411 9.7.2 Sparsity by Pruning 413 9.8 Probabilistic Approaches to Kernel Adaptive Filtering 414 9.8.1 Gaussian Processes and Kernel Ridge Regression 415 9.8.2 Online Recursive Solution for Gaussian Processes Regression 416 9.8.3 Kernel Recursive Least Squares Tracker 417 9.8.4 Probabilistic Kernel Least Mean Squares 418 9.9 Further Reading 418 9.9.1 Selection of Kernel Parameters 418 9.9.2 Multi-Kernel Adaptive Filtering 419 9.9.3 Recursive Filtering in Kernel Hilbert Spaces 419 9.10 Tutorials and Application Examples 419 9.10.1 Kernel Adaptive Filtering Toolbox 420 9.10.2 Prediction of a Respiratory Motion Time Series 421 9.10.3 Online Regression on the KIN?h?eK Dataset 423 9.10.4 The Mackey-Glass Time Series 425 9.10.5 Explicit Recursivity on Reproducing Kernel in Hilbert Space and Electroencephalogram Prediction 427 9.10.6 Adaptive Antenna Array Processing 428 9.11 Questions and Problems 430 Part III Classification, Detection, and Feature Extraction 433 10 Support Vector Machine and Kernel Classification Algorithms 435 10.1 Introduction 435 10.2 Support Vector Machine and Kernel Classi
ers 435 10.2.1 Support Vector Machines 435 10.2.2 Multiclass and Multilabel Support Vector Machines 441 10.2.3 Least-Squares Support Vector Machine 447 10.2.4 Kernel Fisher's Discriminant Analysis 448 10.3 Advances in Kernel-Based Classi
cation 452 10.3.1 Large Margin Filtering 452 10.3.2 Semisupervised Learning 454 10.3.3 Multiple Kernel Learning 460 10.3.4 Structured-Output Learning 462 10.3.5 Active Learning 468 10.4 Large-Scale Support Vector Machines 477 10.4.1 Large-Scale Support Vector Machine Implementations 477 10.4.2 Random Fourier Features 478 10.4.3 Parallel Support Vector Machine 480 10.4.4 Outlook 483 10.5 Tutorials and Application Examples 485 10.5.1 Examples of Support Vector Machine Classi
cation 485 10.5.2 Example of Least-Squares Support Vector Machine 492 10.5.3 Kernel-Filtering Support Vector Machine for Brain-Computer Interface Signal Classi
cation 493 10.5.4 Example of Laplacian Support Vector Machine 494 10.5.5 Example of Graph-Based Label Propagation 498 10.5.6 Examples of Multiple Kernel Learning 498 10.6 Concluding Remarks 501 10.7 Questions and Problems 502 11 Clustering and Anomaly Detection with Kernels 503 11.1 Introduction 503 11.2 Kernel Clustering 506 11.2.1 Kernelization of the Metric 506 11.2.2 Clustering in Feature Spaces 508 11.3 Domain Description Via Support Vectors 514 11.3.1 Support Vector Domain Description 514 11.3.2 One-Class Support Vector Machine 515 11.3.3 Relationship Between Support Vector Domain Description and Density Estimation 516 11.3.4 Semisupervised One-Class Classi
cation 517 11.4 Kernel Matched Subspace Detectors 518 11.4.1 Kernel Orthogonal Subspace Projection 518 11.4.2 Kernel Spectral Angle Mapper 520 11.5 Kernel Anomaly Change Detection 522 11.5.1 Linear Anomaly Change Detection Algorithms 522 11.5.2 Kernel Anomaly Change Detection Algorithms 523 11.6 Hypothesis Testing with Kernels 525 11.6.1 Distribution Embeddings 526 11.6.3 Maximum Mean Discrepancy 527 11.6.3 One-Class Support Measure Machine 528 11.7 Tutorials and Application Examples 529 11.7.1 Example on Kernelization of the Metric 529 11.7.2 Example on Kernel k-Means 530 11.7.3 Domain Description Examples 531 11.7.4 Kernel Spectral Angle Mapper and Kernel Orthogonal Subspace Projection Examples 534 11.7.5 Example of Kernel Anomaly Change Detection Algorithms 536 11.7.6 Example on Distribution Embeddings and Maximum Mean Discrepancy 540 11.8 Concluding Remarks 541 11.9 Questions and Problems 542 12 Kernel Feature Extraction in Signal Processing 543 12.1 Introduction 543 12.2 Multivariate Analysis in Reproducing Kernel Hilbert Spaces 545 12.2.1 Problem Statement and Notation 545 12.2.2 Linear Multivariate Analysis 546 12.2.3 Kernel Multivariate Analysis 549 12.2.4 Multivariate Analysis Experiments 551 12.3 Feature Extraction with Kernel Dependence Estimates 555 12.3.1 Feature Extraction Using Hilbert-Schmidt Independence Criterion 556 12.3.2 Blind Source Separation Using Kernels 563 12.4 Extensions for Large-Scale and Semisupervised Problems 570 12.4.2 E
ciency with the Incomplete Cholesky Decomposition 570 12.4.3 E
ciency with Random Fourier Features 570 12.4.3 Sparse Kernel Feature Extraction 571 12.4.4 Semisupervised Kernel Feature Extraction 573 12.5 Domain Adaptation with Kernels 575 12.5.1 Kernel Mean Matching 578 12.5.2 Transfer Component Analysis 579 12.5.3 Kernel Manifold Alignment 581 12.5.4 Relations between Domain Adaptation Methods 585 12.5.5 Experimental Comparison between Domain Adaptation Methods 12.6 Concluding Remarks 587 12.7 Questions and Problems 588 References 589 Index 631