Discrete-Event Simulation and System Dynamics for Management Decision Making (eBook, ePUB)
Redaktion: Brailsford, Sally; Dangerfield, Brian; Churilov, Leonid
Alle Infos zum eBook verschenken
Discrete-Event Simulation and System Dynamics for Management Decision Making (eBook, ePUB)
Redaktion: Brailsford, Sally; Dangerfield, Brian; Churilov, Leonid
- Format: ePub
- Merkliste
- Auf die Merkliste
- Bewerten Bewerten
- Teilen
- Produkt teilen
- Produkterinnerung
- Produkterinnerung
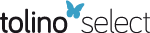
Hier können Sie sich einloggen
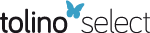
Bitte loggen Sie sich zunächst in Ihr Kundenkonto ein oder registrieren Sie sich bei bücher.de, um das eBook-Abo tolino select nutzen zu können.
In recent years, there has been a growing debate, particularly in the UK and Europe, over the merits of using discrete-event simulation (DES) and system dynamics (SD); there are now instances where both methodologies were employed on the same problem. This book details each method, comparing each in terms of both theory and their application to various problem situations. It also provides a seamless treatment of various topics--theory, philosophy, detailed mechanics, practical implementation--providing a systematic treatment of the methodologies of DES and SD, which previously have been treated separately.…mehr
- Geräte: eReader
- mit Kopierschutz
- eBook Hilfe
- Größe: 4.29MB
- Discrete-Event Simulation and System Dynamics for Management Decision Making (eBook, PDF)80,99 €
- Hanan LussEquitable Resource Allocation (eBook, ePUB)107,99 €
- Robin G. QiuService Science (eBook, ePUB)113,99 €
- Healthcare Analytics (eBook, ePUB)109,99 €
- Circular Economy for the Management of Operations (eBook, ePUB)48,95 €
- Gregory L. SchlegelSupply Chain Risk Management (eBook, ePUB)85,95 €
- Jean-Charles PomerolDecision Making and Action (eBook, ePUB)139,99 €
-
-
-
Dieser Download kann aus rechtlichen Gründen nur mit Rechnungsadresse in A, B, BG, CY, CZ, D, DK, EW, E, FIN, F, GR, HR, H, IRL, I, LT, L, LR, M, NL, PL, P, R, S, SLO, SK ausgeliefert werden.
- Produktdetails
- Verlag: John Wiley & Sons
- Seitenzahl: 360
- Erscheinungstermin: 31. März 2014
- Englisch
- ISBN-13: 9781118762752
- Artikelnr.: 40776759
- Verlag: John Wiley & Sons
- Seitenzahl: 360
- Erscheinungstermin: 31. März 2014
- Englisch
- ISBN-13: 9781118762752
- Artikelnr.: 40776759
- Herstellerkennzeichnung Die Herstellerinformationen sind derzeit nicht verfügbar.
List of contributors xvii
1 Introduction 1
Sally Brailsford, Leonid Churilov and Brian Dangerfield
1.1 How this book came about 1
1.2 The editors 2
1.3 Navigating the book 3
References 9
2 Discrete-event simulation: A primer 10
Stewart Robinson
2.1 Introduction 10
2.2 An example of a discrete-event simulation: Modelling a hospital
theatres process 11
2.3 The technical perspective: How DES works 12
2.3.1 Time handling in DES 14
2.3.2 Random sampling in DES 15
2.4 The philosophical perspective: The DES worldview 21
2.5 Software for DES 23
2.6 Conclusion 24
References 24
3 Systems thinking and system dynamics: A primer 26
Brian Dangerfield
3.1 Introduction 26
3.2 Systems thinking 28
3.2.1 'Behaviour over time' graphs 28
3.2.2 Archetypes 29
3.2.3 Principles of influence (or causal loop) diagrams 30
3.2.4 From diagrams to behaviour 32
3.3 System dynamics 34
3.3.1 Principles of stock-flow diagramming 34
3.3.2 Model purpose and model conceptualisation 35
3.3.3 Adding auxiliaries, parameters and information links to the spinal
stock-flow structure 36
3.3.4 Equation writing and dimensional checking 37
3.4 Some further important issues in SD modelling 40
3.4.1 Use of soft variables 40
3.4.2 Co-flows 42
3.4.3 Delays and smoothing functions 43
3.4.4 Model validation 46
3.4.5 Optimisation of SD models 48
3.4.6 The role of data in SD models 49
3.5 Further reading 49
References 50
4 Combining problem structuring methods with simulation: The philosophical
and practical challenges 52
Kathy Kotiadis and John Mingers
4.1 Introduction 52
4.2 What are problem structuring methods? 53
4.3 Multiparadigm multimethodology in management science 54
4.3.1 Paradigm incommensurability 55
4.3.2 Cultural difficulties 57
4.3.3 Cognitive difficulties 58
4.3.4 Practical problems 59
4.4 Relevant projects and case studies 60
4.5 The case study: Evaluating intermediate care 62
4.5.1 The problem situation 62
4.5.2 Soft systems methodology 64
4.5.3 Discrete-event simulation modelling 66
4.5.4 Multimethodology 67
4.6 Discussion 68
4.6.1 The multiparadigm multimethodology position and strategy 68
4.6.2 The cultural difficulties 70
4.6.3 The cognitive difficulties 70
4.7 Conclusions 72
Acknowledgements 72
References 72
5 Philosophical positioning of discrete-event simulation and system
dynamics as management science tools for process systems: A critical
realist perspective 76
Kristian Rotaru, Leonid Churilov and Andrew Flitman
5.1 Introduction 76
5.2 Ontological and epistemological assumptions of CR 80
5.2.1 The stratified CR ontology 80
5.2.2 The abductive mode of reasoning 81
5.3 Process system modelling with SD and DES through the prism of CR
scientific positioning 82
5.3.1 Lifecycle perspective on SD and DES methods 84
5.4 Process system modelling with SD and DES: Trends in and implications
for MS 90
5.5 Summary and conclusions 97
References 99
6 Theoretical comparison of discrete-event simulation and system dynamics
105
Sally Brailsford
6.1 Introduction 105
6.2 System dynamics 106
6.3 Discrete-event simulation 108
6.4 Summary: The basic differences 110
6.5 Example: Modelling emergency care in Nottingham 112
6.5.1 Background 112
6.5.2 The ECOD project 113
6.5.3 Choice of modelling approach 114
6.5.4 Quantitative phase 114
6.5.5 Model validation 116
6.5.6 Scenario testing and model results 116
6.5.7 The ED model 118
6.5.8 Discussion 119
6.6 The $64 000 question: Which to choose? 120
6.7 Conclusion 123
References 123
7 Models as interfaces 125
Steffen Bayer, Tim Bolt, Sally Brailsford and Maria Kapsali
7.1 Introduction: Models at the interfaces or models as interfaces 125
7.2 The social roles of simulation 126
7.3 The modelling process 129
7.4 The modelling approach 131
7.5 Two case studies of modelling projects 134
7.6 Summary and conclusions 137
References 138
8 An empirical study comparing model development in discrete-event
simulation and system dynamics 140
Antuela Tako and Stewart Robinson
8.1 Introduction 140
8.2 Existing work comparing DES and SD modelling 142
8.2.1 DES and SD model development process 143
8.2.2 Summary 146
8.3 The study 146
8.3.1 The case study 146
8.3.2 Verbal protocol analysis 147
8.3.3 The VPA sessions 149
8.3.4 The subjects 149
8.3.5 The coding process 150
8.4 Study results 151
8.4.1 Attention paid to modelling topics 152
8.4.2 The sequence of modelling stages 154
8.4.3 Pattern of iterations among topics 155
8.5 Observations from the DES and SD expert modellers' behaviour 158
8.6 Conclusions 160
Acknowledgements 162
References 162
9 Explaining puzzling dynamics: A comparison of system dynamics and
discrete-event simulation 165
John Morecroft and Stewart Robinson
9.1 Introduction 165
9.2 Existing comparisons of SD and DES 166
9.3 Research focus 169
9.4 Erratic fisheries - chance, destiny and limited foresight 170
9.5 Structure and behaviour in fisheries: A comparison of SD and DES models
173
9.5.1 Alternative models of a natural fishery 174
9.5.2 Alternative models of a simple harvested fishery 178
9.5.3 Alternative models of a harvested fishery with endogenous ship
purchasing 184
9.6 Summary of findings 192
9.7 Limitations of the study 193
9.8 SD or DES? 194
Acknowledgements 196
References 196
10 DES view on simulation modelling: SIMUL8 199
Mark Elder
10.1 Introduction 199
10.2 How software fits into the project 200
10.3 Building a DES 202
10.4 Getting the right results from a DES 208
10.4.1 Verification and validation 210
10.4.2 Replications 211
10.5 What happens after the results? 212
10.6 What else does DES software do and why? 212
10.7 What next for DES software? 213
References 214
11 Vensim and the development of system dynamics 215
Lee Jones
11.1 Introduction 215
11.2 Coping with complexity: The need for system dynamics 216
11.3 Complexity arms race 219
11.4 The move to user-led innovation 221
11.5 Software support 222
11.5.1 Apples and oranges (basic model testing) 223
11.5.2 Confidence 224
11.5.3 Helping the practitioner do more 237
11.6 The future for SD software 245
11.6.1 Innovation 245
11.6.2 Communication 245
References 247
12 Multi-method modeling: AnyLogic 248
Andrei Borshchev
12.1 Architectures 249
12.1.1 The choice of model architecture and methods 251
12.2 Technical aspect of combining modeling methods 252
12.2.1 System dynamics ® discrete elements 252
12.2.2 Discrete elements ® system dynamics 253
12.2.3 Agent based ' discrete event 255
12.3 Example: Consumer market and supply chain 257
12.3.1 The supply chain model 257
12.3.2 The market model 258
12.3.3 Linking the DE and the SD parts 259
12.3.4 The inventory policy 260
12.4 Example: Epidemic and clinic 262
12.4.1 The epidemic model 262
12.4.2 The clinic model and the integration of methods 264
12.5 Example: Product portfolio and investment policy 267
12.5.1 Assumptions 268
12.5.2 The model architecture 270
12.5.3 The agent product and agent population portfolio 271
12.5.4 The investment policy 274
12.5.5 Closing the loop and implementing launch of new products 275
12.5.6 Completing the investment policy 277
12.6 Discussion 278
References 279
13 Multiscale modelling for public health management: A practical guide 280
Rosemarie Sadsad and Geoff McDonnell
13.1 Introduction 280
13.2 Background 281
13.3 Multilevel system theories and methodologies 281
13.4 Multiscale simulation modelling and management 283
13.5 Discussion 289
13.6 Conclusion 290
References 290
14 Hybrid modelling case studies 295
Rosemarie Sadsad, Geoff McDonnell, Joe Viana, Shivam M. Desai, Paul Harper
and Sally Brailsford
14.1 Introduction 295
14.2 A multilevel model of MRSA endemicity and its control in hospitals 296
14.2.1 Introduction 296
14.2.2 Method 296
14.2.3 Results 297
14.2.4 Conclusion 302
14.3 Chlamydia composite model 302
14.3.1 Introduction 302
14.3.2 Chlamydia 302
14.3.3 DES model of a GUM department 303
14.3.4 SD model of chlamydia 304
14.3.5 Why combine the models 304
14.3.6 How the models were combined 305
14.3.7 Experiments with the composite model 305
14.3.8 Conclusions 307
14.4 A hybrid model for social care services operations 308
14.4.1 Introduction 308
14.4.2 Population model 308
14.4.3 Model construction 309
14.4.4 Contact centre model 310
14.4.5 Hybrid model 311
14.4.6 Conclusions and lessons learnt 313
References 316
15 The ways forward: A personal view of system dynamics and discrete-event
simulation 318
Michael Pidd
15.1 Genesis 318
15.2 Computer simulation in management science 319
15.3 The effect of developments in computing 320
15.4 The importance of process 324
15.5 My own comparison of the simulation approaches 324
15.5.1 Time handling 324
15.5.2 Stochastic and deterministic elements 326
15.5.3 Discrete entities versus continuous variables 327
15.6 Linking system dynamics and discrete-event simulation 328
15.7 The importance of intended model use 329
15.7.1 Decision automation 330
15.7.2 Routine decision support 331
15.7.3 System investigation and improvement 331
15.7.4 Providing insights for debate 332
15.8 The future? 333
15.8.1 Use of both methods will continue to grow 333
15.8.2 Developments in computing will continue to have an effect 334
15.8.3 Process really matters 335
References 335
Index 337
List of contributors xvii
1 Introduction 1
Sally Brailsford, Leonid Churilov and Brian Dangerfield
1.1 How this book came about 1
1.2 The editors 2
1.3 Navigating the book 3
References 9
2 Discrete-event simulation: A primer 10
Stewart Robinson
2.1 Introduction 10
2.2 An example of a discrete-event simulation: Modelling a hospital
theatres process 11
2.3 The technical perspective: How DES works 12
2.3.1 Time handling in DES 14
2.3.2 Random sampling in DES 15
2.4 The philosophical perspective: The DES worldview 21
2.5 Software for DES 23
2.6 Conclusion 24
References 24
3 Systems thinking and system dynamics: A primer 26
Brian Dangerfield
3.1 Introduction 26
3.2 Systems thinking 28
3.2.1 'Behaviour over time' graphs 28
3.2.2 Archetypes 29
3.2.3 Principles of influence (or causal loop) diagrams 30
3.2.4 From diagrams to behaviour 32
3.3 System dynamics 34
3.3.1 Principles of stock-flow diagramming 34
3.3.2 Model purpose and model conceptualisation 35
3.3.3 Adding auxiliaries, parameters and information links to the spinal
stock-flow structure 36
3.3.4 Equation writing and dimensional checking 37
3.4 Some further important issues in SD modelling 40
3.4.1 Use of soft variables 40
3.4.2 Co-flows 42
3.4.3 Delays and smoothing functions 43
3.4.4 Model validation 46
3.4.5 Optimisation of SD models 48
3.4.6 The role of data in SD models 49
3.5 Further reading 49
References 50
4 Combining problem structuring methods with simulation: The philosophical
and practical challenges 52
Kathy Kotiadis and John Mingers
4.1 Introduction 52
4.2 What are problem structuring methods? 53
4.3 Multiparadigm multimethodology in management science 54
4.3.1 Paradigm incommensurability 55
4.3.2 Cultural difficulties 57
4.3.3 Cognitive difficulties 58
4.3.4 Practical problems 59
4.4 Relevant projects and case studies 60
4.5 The case study: Evaluating intermediate care 62
4.5.1 The problem situation 62
4.5.2 Soft systems methodology 64
4.5.3 Discrete-event simulation modelling 66
4.5.4 Multimethodology 67
4.6 Discussion 68
4.6.1 The multiparadigm multimethodology position and strategy 68
4.6.2 The cultural difficulties 70
4.6.3 The cognitive difficulties 70
4.7 Conclusions 72
Acknowledgements 72
References 72
5 Philosophical positioning of discrete-event simulation and system
dynamics as management science tools for process systems: A critical
realist perspective 76
Kristian Rotaru, Leonid Churilov and Andrew Flitman
5.1 Introduction 76
5.2 Ontological and epistemological assumptions of CR 80
5.2.1 The stratified CR ontology 80
5.2.2 The abductive mode of reasoning 81
5.3 Process system modelling with SD and DES through the prism of CR
scientific positioning 82
5.3.1 Lifecycle perspective on SD and DES methods 84
5.4 Process system modelling with SD and DES: Trends in and implications
for MS 90
5.5 Summary and conclusions 97
References 99
6 Theoretical comparison of discrete-event simulation and system dynamics
105
Sally Brailsford
6.1 Introduction 105
6.2 System dynamics 106
6.3 Discrete-event simulation 108
6.4 Summary: The basic differences 110
6.5 Example: Modelling emergency care in Nottingham 112
6.5.1 Background 112
6.5.2 The ECOD project 113
6.5.3 Choice of modelling approach 114
6.5.4 Quantitative phase 114
6.5.5 Model validation 116
6.5.6 Scenario testing and model results 116
6.5.7 The ED model 118
6.5.8 Discussion 119
6.6 The $64 000 question: Which to choose? 120
6.7 Conclusion 123
References 123
7 Models as interfaces 125
Steffen Bayer, Tim Bolt, Sally Brailsford and Maria Kapsali
7.1 Introduction: Models at the interfaces or models as interfaces 125
7.2 The social roles of simulation 126
7.3 The modelling process 129
7.4 The modelling approach 131
7.5 Two case studies of modelling projects 134
7.6 Summary and conclusions 137
References 138
8 An empirical study comparing model development in discrete-event
simulation and system dynamics 140
Antuela Tako and Stewart Robinson
8.1 Introduction 140
8.2 Existing work comparing DES and SD modelling 142
8.2.1 DES and SD model development process 143
8.2.2 Summary 146
8.3 The study 146
8.3.1 The case study 146
8.3.2 Verbal protocol analysis 147
8.3.3 The VPA sessions 149
8.3.4 The subjects 149
8.3.5 The coding process 150
8.4 Study results 151
8.4.1 Attention paid to modelling topics 152
8.4.2 The sequence of modelling stages 154
8.4.3 Pattern of iterations among topics 155
8.5 Observations from the DES and SD expert modellers' behaviour 158
8.6 Conclusions 160
Acknowledgements 162
References 162
9 Explaining puzzling dynamics: A comparison of system dynamics and
discrete-event simulation 165
John Morecroft and Stewart Robinson
9.1 Introduction 165
9.2 Existing comparisons of SD and DES 166
9.3 Research focus 169
9.4 Erratic fisheries - chance, destiny and limited foresight 170
9.5 Structure and behaviour in fisheries: A comparison of SD and DES models
173
9.5.1 Alternative models of a natural fishery 174
9.5.2 Alternative models of a simple harvested fishery 178
9.5.3 Alternative models of a harvested fishery with endogenous ship
purchasing 184
9.6 Summary of findings 192
9.7 Limitations of the study 193
9.8 SD or DES? 194
Acknowledgements 196
References 196
10 DES view on simulation modelling: SIMUL8 199
Mark Elder
10.1 Introduction 199
10.2 How software fits into the project 200
10.3 Building a DES 202
10.4 Getting the right results from a DES 208
10.4.1 Verification and validation 210
10.4.2 Replications 211
10.5 What happens after the results? 212
10.6 What else does DES software do and why? 212
10.7 What next for DES software? 213
References 214
11 Vensim and the development of system dynamics 215
Lee Jones
11.1 Introduction 215
11.2 Coping with complexity: The need for system dynamics 216
11.3 Complexity arms race 219
11.4 The move to user-led innovation 221
11.5 Software support 222
11.5.1 Apples and oranges (basic model testing) 223
11.5.2 Confidence 224
11.5.3 Helping the practitioner do more 237
11.6 The future for SD software 245
11.6.1 Innovation 245
11.6.2 Communication 245
References 247
12 Multi-method modeling: AnyLogic 248
Andrei Borshchev
12.1 Architectures 249
12.1.1 The choice of model architecture and methods 251
12.2 Technical aspect of combining modeling methods 252
12.2.1 System dynamics ® discrete elements 252
12.2.2 Discrete elements ® system dynamics 253
12.2.3 Agent based ' discrete event 255
12.3 Example: Consumer market and supply chain 257
12.3.1 The supply chain model 257
12.3.2 The market model 258
12.3.3 Linking the DE and the SD parts 259
12.3.4 The inventory policy 260
12.4 Example: Epidemic and clinic 262
12.4.1 The epidemic model 262
12.4.2 The clinic model and the integration of methods 264
12.5 Example: Product portfolio and investment policy 267
12.5.1 Assumptions 268
12.5.2 The model architecture 270
12.5.3 The agent product and agent population portfolio 271
12.5.4 The investment policy 274
12.5.5 Closing the loop and implementing launch of new products 275
12.5.6 Completing the investment policy 277
12.6 Discussion 278
References 279
13 Multiscale modelling for public health management: A practical guide 280
Rosemarie Sadsad and Geoff McDonnell
13.1 Introduction 280
13.2 Background 281
13.3 Multilevel system theories and methodologies 281
13.4 Multiscale simulation modelling and management 283
13.5 Discussion 289
13.6 Conclusion 290
References 290
14 Hybrid modelling case studies 295
Rosemarie Sadsad, Geoff McDonnell, Joe Viana, Shivam M. Desai, Paul Harper
and Sally Brailsford
14.1 Introduction 295
14.2 A multilevel model of MRSA endemicity and its control in hospitals 296
14.2.1 Introduction 296
14.2.2 Method 296
14.2.3 Results 297
14.2.4 Conclusion 302
14.3 Chlamydia composite model 302
14.3.1 Introduction 302
14.3.2 Chlamydia 302
14.3.3 DES model of a GUM department 303
14.3.4 SD model of chlamydia 304
14.3.5 Why combine the models 304
14.3.6 How the models were combined 305
14.3.7 Experiments with the composite model 305
14.3.8 Conclusions 307
14.4 A hybrid model for social care services operations 308
14.4.1 Introduction 308
14.4.2 Population model 308
14.4.3 Model construction 309
14.4.4 Contact centre model 310
14.4.5 Hybrid model 311
14.4.6 Conclusions and lessons learnt 313
References 316
15 The ways forward: A personal view of system dynamics and discrete-event
simulation 318
Michael Pidd
15.1 Genesis 318
15.2 Computer simulation in management science 319
15.3 The effect of developments in computing 320
15.4 The importance of process 324
15.5 My own comparison of the simulation approaches 324
15.5.1 Time handling 324
15.5.2 Stochastic and deterministic elements 326
15.5.3 Discrete entities versus continuous variables 327
15.6 Linking system dynamics and discrete-event simulation 328
15.7 The importance of intended model use 329
15.7.1 Decision automation 330
15.7.2 Routine decision support 331
15.7.3 System investigation and improvement 331
15.7.4 Providing insights for debate 332
15.8 The future? 333
15.8.1 Use of both methods will continue to grow 333
15.8.2 Developments in computing will continue to have an effect 334
15.8.3 Process really matters 335
References 335
Index 337