Effective Experimentation (eBook, ePUB)
For Scientists and Technologists
Alle Infos zum eBook verschenken
Effective Experimentation (eBook, ePUB)
For Scientists and Technologists
- Format: ePub
- Merkliste
- Auf die Merkliste
- Bewerten Bewerten
- Teilen
- Produkt teilen
- Produkterinnerung
- Produkterinnerung
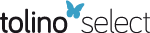
Hier können Sie sich einloggen
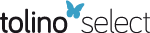
Bitte loggen Sie sich zunächst in Ihr Kundenkonto ein oder registrieren Sie sich bei bücher.de, um das eBook-Abo tolino select nutzen zu können.
Effective Experimentation is a practical book on how to design and analyse experiments. Each of the methods are introduced and illustrated through real world scenario drawn from industry or research. Formulae are kept to a minimum to enable the reader to concentrate on how to apply and understand the different methods presented. The book has been developed from courses run by Statistics for Industry Limited during which time more than 10,000 scientists and technologists have gained the knowledge and confidence to plan experiments successfully and to analyse their data. Each chapter starts with…mehr
- Geräte: eReader
- mit Kopierschutz
- eBook Hilfe
- Größe: 7.41MB
- Peter GoosOptimal Design of Experiments (eBook, ePUB)72,99 €
- Richard BoddyEffective Experimentation (eBook, PDF)41,99 €
- N. W. GalweyIntroduction to Mixed Modelling (eBook, ePUB)78,99 €
- C. F. Jeff WuExperiments (eBook, ePUB)140,99 €
- Design and Analysis of Experiments, Volume 3 (eBook, ePUB)127,99 €
- Bradley HuitemaThe Analysis of Covariance and Alternatives (eBook, ePUB)127,99 €
- Ajit C. TamhaneStatistical Analysis of Designed Experiments (eBook, ePUB)138,99 €
-
-
-
Dieser Download kann aus rechtlichen Gründen nur mit Rechnungsadresse in A, B, BG, CY, CZ, D, DK, EW, E, FIN, F, GR, HR, H, IRL, I, LT, L, LR, M, NL, PL, P, R, S, SLO, SK ausgeliefert werden.
- Produktdetails
- Verlag: John Wiley & Sons
- Seitenzahl: 270
- Erscheinungstermin: 9. Juni 2011
- Englisch
- ISBN-13: 9781119957423
- Artikelnr.: 37348600
- Verlag: John Wiley & Sons
- Seitenzahl: 270
- Erscheinungstermin: 9. Juni 2011
- Englisch
- ISBN-13: 9781119957423
- Artikelnr.: 37348600
- Herstellerkennzeichnung Die Herstellerinformationen sind derzeit nicht verfügbar.
1 Why Bother to Design an Experiment?
1.1 Indroduction.
1.2 Examples and Benefits.
1.2.1 Develop a Better Product.
1.2.2 Which Antiperspirant is Best?
1.2.3 A Complex Project.
1.3 Good Design and Good Analysis.
2 A Change for the Better - Significance Testing.
2.1 Introduction.
2.2 Towards a Darker Stout.
2.3 Summary Statistics.
2.4 The Normal Distribution.
2.5 How Accurate is My Mean?
2.6 Is the New Additive an Improvement?
2.7 How Many Trials are Needed for an Experiment?
2.8 Were the Aims of the Investigation Achieved?
2.9 Problems.
3 Improving Effectiveness Using a Paired Design.
3.1 Introduction.
3.2 An Example: Who Wears the Trousers?
3.3 How Do We Rate the Wear?
3.4 How Often Do You Carry Out an Assessment?
3.5 Choosing the Participants.
3.6 Controlling the Participants.
3.7 The Paired Design.
3.8 Was the Experiment Successful?
3.9 Problems.
4 A Simple but Effective Design for Two Variables.
4.1 Introduction.
4.2 An Investigation.
4.3 Limitations of a One-Variable-at-a-Time Experiment.
4.4 A Factorial Experiment.
4.5 Confidence Intervals for Effect Estimates.
4.6 What Conditions Should be Recommended?
4.7 Were the Aims of the Investigation Achieved?
4.8 Problems.
5 Investigating 3 and 4 Variables in an Experiment.
5.1 Introduction.
5.2 An Experiment with Three Variables.
5.3 The Design Matrix Method.
5.4 Computation of Predicted Values.
5.5 Computation of Confidence Interval.
5.6 95% Confidence Interval for an Effect.
5.7 95% Confidence Interval for a Predicted Value.
5.8 Sequencing of the Trials.
5.9 Were the Aims of the Experiment Achieved?
5.10 A Four-Variable Experiment.
5.11 Half-Normal Plots.
5.12 Were the Aims of the Experiment Achieved?
5.13 Problems.
6 More for Even Less: Using a Fraction of a Full Design.
6.1 Introduction.
6.2 Obtaining Half-Fractional Designs.
6.2.1 With Defining Contrast ABC.
6.2.2 With Defining Contrast AC.
6.3 Design of ¿(24) Experiment.
6.4 Analysing a Fractional Experiment.
6.5 Summary 59
6.6 Did Wheelwright Achieve the Aims of His Experiment?
6.7 When and Where to Choose a Fractional Design.
6.7.1 Three Variables.
6.7.2 Four Variables.
6.7.3 Five Variables or More.
6.8 Problems.
7 Saturated Designs.
7.1 Introduction.
7.2 Towards a Better Oil?
7.3 The Experiment.
7.4 An Alternative Procedure for Estimating the Residual SD.
7.5 Did Doug Achieve the Aims of His Experiment?
7.6 How Rugged is My Method?
7.7 Analysis of the Design.
7.8 Conclusions from the Experiment.
7.9 Did Serena Achieve Her Aims?
7.10 Which Order Should I Use for the Trials?
7.11 How to Obtain the Designs.
7.12 Other Uses of Saturated Designs.
7.13 Problems.
8 Regression Analysis.
8.1 Introduction.
8.2 Example: Keeping Quality of Sprouts.
8.3 How Good a Fit Has the Line to the Data?
8.4 Residuals.
8.5 Percentage Fit.
8.6 Correlation Coefficient.
8.7 Percentage Fit - An Easier Method.
8.8 Is There a Significant Relationship between the Variables?
8.9 Confidence Intervals for the Regression Statistics.
8.10 Assumptions.
8.11 Problem.
9 Multiple Regression: The First Essentials.
9.1 Introduction.
9.2 An Experiment to Improve the Yield.
9.3 Building a Regression Model.
9.4 Selecting the First Independent Variable.
9.5 Relationship between Yield and Weight.
9.6 Model Building.
9.7 Selecting the Second Independent Variable.
9.8 An Alternative Model.
9.9 Limitations to the Analysis.
9.10 Was the Experiment Successful?
9.11 Problems.
10 Designs to Generate Response Surfaces.
10.1 Introduction.
10.2 An Example: Easing the Digestion.
10.3 Analysis of Crushing Strength.
10.4 Analysis of Dissolution Time.
10.5 How Many Levels of a Variable Should We Use in a Design?
10.6 Was the Experiment Successful?
10.7 Problem.
11 Outliers and Influential Observations.
11.1 Introduction.
11.2 An Outlier in One Variable.
11.3 Other Outlier Tests.
11.4 Outliers in Regression.
11.5 Influential Observations.
11.6 Outliers and Influence in Multiple Regression.
11.7 What to Do After Detection?
12 Central Composite Designs.
12.1 Introduction.
12.2 An Example: Design the Crunchiness.
12.3 Estimating the Variability.
12.4 Estimating the Effects.
12.5 Using Multiple Regression.
12.6 Second Stage of the Design.
12.7 Has the Experiment Been Successful?
12.8 Choosing a Central Composite Design.
12.9 Critique of Central Composite Designs.
13 Designs For Mixtures.
13.1 Introduction.
13.2 Mixtures of Two Components.
13.3 A Concrete Case Study.
13.4 Design and Analysis for a 3-Component Mixture.
13.5 Designs with Mixture Variables and Process Variables.
13.6 Fractional Experiments.
13.7 Was the Experiment Successful?
14 Computer-Aided Experimental Design (CAED).
14.1 Introduction.
14.2 How It Works.
14.3 An Example.
14.4 Selecting the Repertoire.
14.5 Selecting the Model and Number of Trials.
14.6 How the Program Chooses the Design Set.
14.7 Summary.
14.8 Problems.
15 Optimization Designs.
15.1 Introduction.
15.2 The Principles behind EVOP.
15.3 EVOP: The Experimental Design.
15.4 Running EVOP Programmes.
15.5 The Principles of Simplex Optimization.
15.6 Simplex Optimization: An Experiment.
15.7 Comparison of EVOP, Simplex and Response Surface Methods.
15.7.1 Number of Trials (Batches) Required.
15.7.2 Production of In-Specification Product.
15.7.3 Obtaining Optimum.
15.7.4 Understanding Variables.
15.7.5 Amount of Judgement Required.
15.7.6 Coping with Nuisance Variables.
16 Improving a Bad Experiment.
16.1 Introduction.
16.2 Was the Experiment Successful?
17 How to Compare Several Treatments.
17.1 Introduction.
17.2 An Example: Which is the Best Treatment?
17.3 Analysis of Variance.
17.4 Multiple Comparison Test.
17.5 Are the Standard Deviations Significantly Different?
17.6 Cochran's Test for Standard Deviations.
17.7 When Should the Above Method Not be Used?
17.8 Was Golightly's Experiment Successful?
17.9 Problems.
18 Experiments in Blocks.
18.1 Introduction.
18.2 An Example: Kill the Sweat.
18.3 Analysis of the Data.
18.4 Benefits of a Randomized Block Experiment.
18.5 Was the Experiment Successful?
18.6 Double and Treble Blocking.
18.7 Example: A Dog's Life.
18.8 The Latin Square Design.
18.9 Latin Square Analysis of Variance.
18.10 Properties and Assumptions of the Latin Square Design.
18.11 Examples of Latin Squares.
18.12 Was the Experiment Successful?
18.13 An Extra Blocking Factor - Graeco-Latin Square.
18.14 Problem.
19 Two-Way Designs.
19.1 Introduction.
19.2 An Example: Improving the Taste of Coffee.
19.3 Two-Way Analysis of Variance.
19.4 Multiple Comparison Test.
19.5 Was the Experiment Successful?
19.6 Problem.
20 Too Much at Once: Incomplete Block Experiments.
20.1 Introduction.
20.2 Example: An Incomplete Block Experiment.
20.3 Adjusted Means.
20.4 Analysis of Variance for Balanced Incomplete Block Design.
20.5 Alternative Designs.
20.6 A Design with a Control.
20.7 Was Jeremy's Experiment Successful?
20.8 Problem.
21 23 Ways of Messing Up an Experiment.
21.1 Introduction.
21.2 23 Ways of Messing Up an Experiment.
21.3 Initial Thoughts When Planning an Experiment.
21.4 Developing the Ideas.
21.5 Designing the Experiment.
21.6 Conducting the Experiment.
21.7 Analysing the Data.
21.8 Summary.
Solutions to Problems.
Statistical Tables.
Index.
1 Why Bother to Design an Experiment?
1.1 Indroduction.
1.2 Examples and Benefits.
1.2.1 Develop a Better Product.
1.2.2 Which Antiperspirant is Best?
1.2.3 A Complex Project.
1.3 Good Design and Good Analysis.
2 A Change for the Better - Significance Testing.
2.1 Introduction.
2.2 Towards a Darker Stout.
2.3 Summary Statistics.
2.4 The Normal Distribution.
2.5 How Accurate is My Mean?
2.6 Is the New Additive an Improvement?
2.7 How Many Trials are Needed for an Experiment?
2.8 Were the Aims of the Investigation Achieved?
2.9 Problems.
3 Improving Effectiveness Using a Paired Design.
3.1 Introduction.
3.2 An Example: Who Wears the Trousers?
3.3 How Do We Rate the Wear?
3.4 How Often Do You Carry Out an Assessment?
3.5 Choosing the Participants.
3.6 Controlling the Participants.
3.7 The Paired Design.
3.8 Was the Experiment Successful?
3.9 Problems.
4 A Simple but Effective Design for Two Variables.
4.1 Introduction.
4.2 An Investigation.
4.3 Limitations of a One-Variable-at-a-Time Experiment.
4.4 A Factorial Experiment.
4.5 Confidence Intervals for Effect Estimates.
4.6 What Conditions Should be Recommended?
4.7 Were the Aims of the Investigation Achieved?
4.8 Problems.
5 Investigating 3 and 4 Variables in an Experiment.
5.1 Introduction.
5.2 An Experiment with Three Variables.
5.3 The Design Matrix Method.
5.4 Computation of Predicted Values.
5.5 Computation of Confidence Interval.
5.6 95% Confidence Interval for an Effect.
5.7 95% Confidence Interval for a Predicted Value.
5.8 Sequencing of the Trials.
5.9 Were the Aims of the Experiment Achieved?
5.10 A Four-Variable Experiment.
5.11 Half-Normal Plots.
5.12 Were the Aims of the Experiment Achieved?
5.13 Problems.
6 More for Even Less: Using a Fraction of a Full Design.
6.1 Introduction.
6.2 Obtaining Half-Fractional Designs.
6.2.1 With Defining Contrast ABC.
6.2.2 With Defining Contrast AC.
6.3 Design of ¿(24) Experiment.
6.4 Analysing a Fractional Experiment.
6.5 Summary 59
6.6 Did Wheelwright Achieve the Aims of His Experiment?
6.7 When and Where to Choose a Fractional Design.
6.7.1 Three Variables.
6.7.2 Four Variables.
6.7.3 Five Variables or More.
6.8 Problems.
7 Saturated Designs.
7.1 Introduction.
7.2 Towards a Better Oil?
7.3 The Experiment.
7.4 An Alternative Procedure for Estimating the Residual SD.
7.5 Did Doug Achieve the Aims of His Experiment?
7.6 How Rugged is My Method?
7.7 Analysis of the Design.
7.8 Conclusions from the Experiment.
7.9 Did Serena Achieve Her Aims?
7.10 Which Order Should I Use for the Trials?
7.11 How to Obtain the Designs.
7.12 Other Uses of Saturated Designs.
7.13 Problems.
8 Regression Analysis.
8.1 Introduction.
8.2 Example: Keeping Quality of Sprouts.
8.3 How Good a Fit Has the Line to the Data?
8.4 Residuals.
8.5 Percentage Fit.
8.6 Correlation Coefficient.
8.7 Percentage Fit - An Easier Method.
8.8 Is There a Significant Relationship between the Variables?
8.9 Confidence Intervals for the Regression Statistics.
8.10 Assumptions.
8.11 Problem.
9 Multiple Regression: The First Essentials.
9.1 Introduction.
9.2 An Experiment to Improve the Yield.
9.3 Building a Regression Model.
9.4 Selecting the First Independent Variable.
9.5 Relationship between Yield and Weight.
9.6 Model Building.
9.7 Selecting the Second Independent Variable.
9.8 An Alternative Model.
9.9 Limitations to the Analysis.
9.10 Was the Experiment Successful?
9.11 Problems.
10 Designs to Generate Response Surfaces.
10.1 Introduction.
10.2 An Example: Easing the Digestion.
10.3 Analysis of Crushing Strength.
10.4 Analysis of Dissolution Time.
10.5 How Many Levels of a Variable Should We Use in a Design?
10.6 Was the Experiment Successful?
10.7 Problem.
11 Outliers and Influential Observations.
11.1 Introduction.
11.2 An Outlier in One Variable.
11.3 Other Outlier Tests.
11.4 Outliers in Regression.
11.5 Influential Observations.
11.6 Outliers and Influence in Multiple Regression.
11.7 What to Do After Detection?
12 Central Composite Designs.
12.1 Introduction.
12.2 An Example: Design the Crunchiness.
12.3 Estimating the Variability.
12.4 Estimating the Effects.
12.5 Using Multiple Regression.
12.6 Second Stage of the Design.
12.7 Has the Experiment Been Successful?
12.8 Choosing a Central Composite Design.
12.9 Critique of Central Composite Designs.
13 Designs For Mixtures.
13.1 Introduction.
13.2 Mixtures of Two Components.
13.3 A Concrete Case Study.
13.4 Design and Analysis for a 3-Component Mixture.
13.5 Designs with Mixture Variables and Process Variables.
13.6 Fractional Experiments.
13.7 Was the Experiment Successful?
14 Computer-Aided Experimental Design (CAED).
14.1 Introduction.
14.2 How It Works.
14.3 An Example.
14.4 Selecting the Repertoire.
14.5 Selecting the Model and Number of Trials.
14.6 How the Program Chooses the Design Set.
14.7 Summary.
14.8 Problems.
15 Optimization Designs.
15.1 Introduction.
15.2 The Principles behind EVOP.
15.3 EVOP: The Experimental Design.
15.4 Running EVOP Programmes.
15.5 The Principles of Simplex Optimization.
15.6 Simplex Optimization: An Experiment.
15.7 Comparison of EVOP, Simplex and Response Surface Methods.
15.7.1 Number of Trials (Batches) Required.
15.7.2 Production of In-Specification Product.
15.7.3 Obtaining Optimum.
15.7.4 Understanding Variables.
15.7.5 Amount of Judgement Required.
15.7.6 Coping with Nuisance Variables.
16 Improving a Bad Experiment.
16.1 Introduction.
16.2 Was the Experiment Successful?
17 How to Compare Several Treatments.
17.1 Introduction.
17.2 An Example: Which is the Best Treatment?
17.3 Analysis of Variance.
17.4 Multiple Comparison Test.
17.5 Are the Standard Deviations Significantly Different?
17.6 Cochran's Test for Standard Deviations.
17.7 When Should the Above Method Not be Used?
17.8 Was Golightly's Experiment Successful?
17.9 Problems.
18 Experiments in Blocks.
18.1 Introduction.
18.2 An Example: Kill the Sweat.
18.3 Analysis of the Data.
18.4 Benefits of a Randomized Block Experiment.
18.5 Was the Experiment Successful?
18.6 Double and Treble Blocking.
18.7 Example: A Dog's Life.
18.8 The Latin Square Design.
18.9 Latin Square Analysis of Variance.
18.10 Properties and Assumptions of the Latin Square Design.
18.11 Examples of Latin Squares.
18.12 Was the Experiment Successful?
18.13 An Extra Blocking Factor - Graeco-Latin Square.
18.14 Problem.
19 Two-Way Designs.
19.1 Introduction.
19.2 An Example: Improving the Taste of Coffee.
19.3 Two-Way Analysis of Variance.
19.4 Multiple Comparison Test.
19.5 Was the Experiment Successful?
19.6 Problem.
20 Too Much at Once: Incomplete Block Experiments.
20.1 Introduction.
20.2 Example: An Incomplete Block Experiment.
20.3 Adjusted Means.
20.4 Analysis of Variance for Balanced Incomplete Block Design.
20.5 Alternative Designs.
20.6 A Design with a Control.
20.7 Was Jeremy's Experiment Successful?
20.8 Problem.
21 23 Ways of Messing Up an Experiment.
21.1 Introduction.
21.2 23 Ways of Messing Up an Experiment.
21.3 Initial Thoughts When Planning an Experiment.
21.4 Developing the Ideas.
21.5 Designing the Experiment.
21.6 Conducting the Experiment.
21.7 Analysing the Data.
21.8 Summary.
Solutions to Problems.
Statistical Tables.
Index.